Prediction of secondary electron yield for metal materials using deep learning
MICROSCOPY(2024)
摘要
This article describes a neural network system for predicting the secondary electron yield of metallic materials. For bulk metals, experimental values are used as training data. Due to the strong correlation between the secondary electron yield and the work function, deep learning predicts the secondary electron yield with relatively high accuracy even with a small amount of training data. Our approach demonstrates the importance of the work function in predicting the secondary electron yield. For the secondary electron yield of thin metal films on metal substrates, deep learning predictions are generated using training data obtained by Monte Carlo simulations. The accuracy of the secondary yield predictions of thin films on substrates could be improved by adding experimental values of bulk metals to the training data.
更多查看译文
关键词
secondary electron,metal,deep learning,Monte Carlo simulation,thin film,work function
AI 理解论文
溯源树
样例
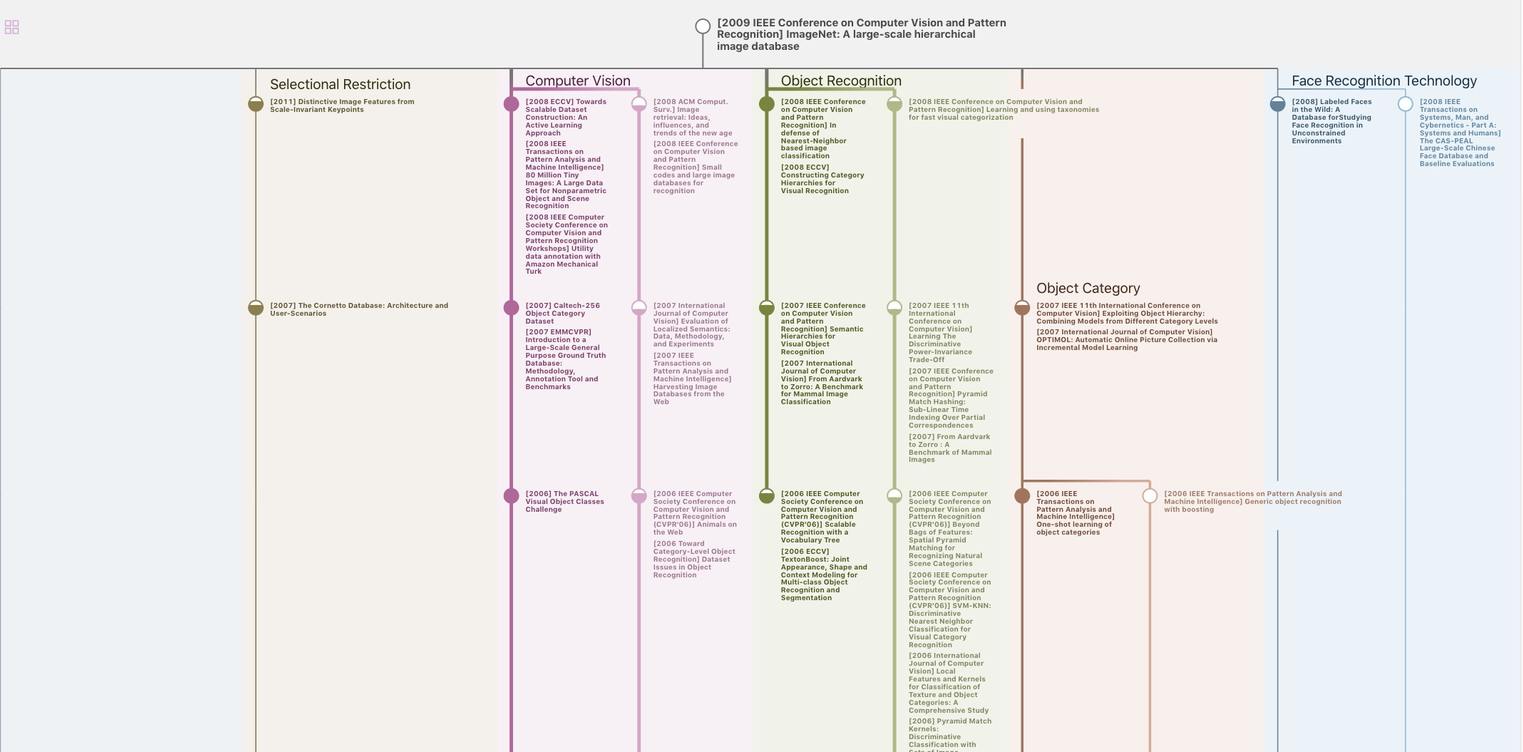
生成溯源树,研究论文发展脉络
Chat Paper
正在生成论文摘要