Collaborative Transfer Network for Multi-Classification of Breast Cancer Histopathological Images
IEEE JOURNAL OF BIOMEDICAL AND HEALTH INFORMATICS(2024)
摘要
The incidence of breast cancer is increasing rapidly around the world. Accurate classification of the breast cancer subtype from hematoxylin and eosin images is the key to improve the precision of treatment. However, the high consistency of disease subtypes and uneven distribution of cancer cells seriously affect the performance of multi-classification methods. Furthermore, it is difficult to apply existing classification methods to multiple datasets. In this article, we propose a collaborative transfer network (CTransNet) for multi-classification of breast cancer histopathological images. CTransNet consists of a transfer learning backbone branch, a residual collaborative branch, and a feature fusion module. The transfer learning branch adopts the pre-trained DenseNet structure to extract image features from ImageNet. The residual branch extracts target features from pathological images in a collaborative manner. The feature fusion strategy of optimizing these two branches is used to train and fine-tune CTransNet. Experiments show that CTransNet achieves 98.29% classification accuracy on the public BreaKHis breast cancer dataset, exceeding the performance of state-of-the-art methods. Visual analysis is carried out under the guidance of oncologists. Based on the training parameters of the BreaKHis dataset, CTransNet achieves superior performance on other two public breast cancer datasets (breast-cancer-grade-ICT and ICIAR2018_BACH_Challenge), indicating that CTransNet has good generalization performance.
更多查看译文
关键词
Breast cancer,Transfer learning,Cancer,Feature extraction,Pathology,Tumors,Deep learning,feature fusion,multi-classification,pathological images,transfer learning
AI 理解论文
溯源树
样例
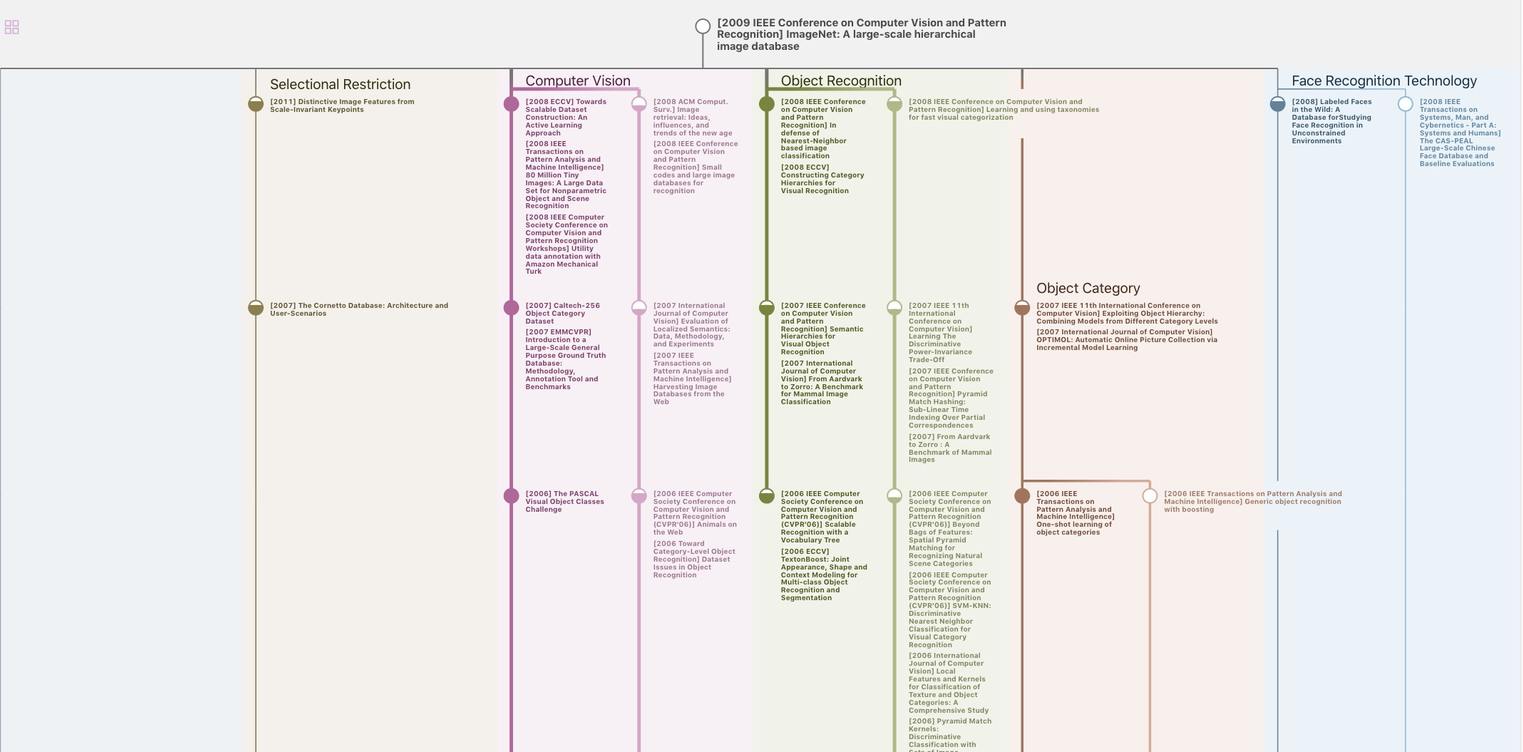
生成溯源树,研究论文发展脉络
Chat Paper
正在生成论文摘要