A unified hybrid transformer for joint MRI sequences super-resolution and missing data imputation
Physics in medicine and biology(2023)
摘要
Objective. High-resolution multi-modal magnetic resonance imaging (MRI) is crucial in clinical practice for accurate diagnosis and treatment. However, challenges such as budget constraints, potential contrast agent deposition, and image corruption often limit the acquisition of multiple sequences from a single patient. Therefore, the development of novel methods to reconstruct under-sampled images and synthesize missing sequences is crucial for clinical and research applications. Approach. In this paper, we propose a unified hybrid framework called SIFormer, which utilizes any available low-resolution MRI contrast configurations to complete super-resolution (SR) of poor-quality MR images and impute missing sequences simultaneously in one forward process. SIFormer consists of a hybrid generator and a convolution-based discriminator. The generator incorporates two key blocks. First, the dual branch attention block combines the long-range dependency building capability of the transformer with the high-frequency local information capture capability of the convolutional neural network in a channel-wise split manner. Second, we introduce a learnable gating adaptation multi-layer perception in the feed-forward block to optimize information transmission efficiently. Main results. Comparative evaluations against six state-of-the-art methods demonstrate that SIFormer achieves enhanced quantitative performance and produces more visually pleasing results for image SR and synthesis tasks across multiple datasets. Significance. Extensive experiments conducted on multi-center multi-contrast MRI datasets, including both healthy individuals and brain tumor patients, highlight the potential of our proposed method to serve as a valuable supplement to MRI sequence acquisition in clinical and research settings.
更多查看译文
关键词
deep learning,image super-resolution,missing MRI synthesis,multi-contrast MRI,transformer
AI 理解论文
溯源树
样例
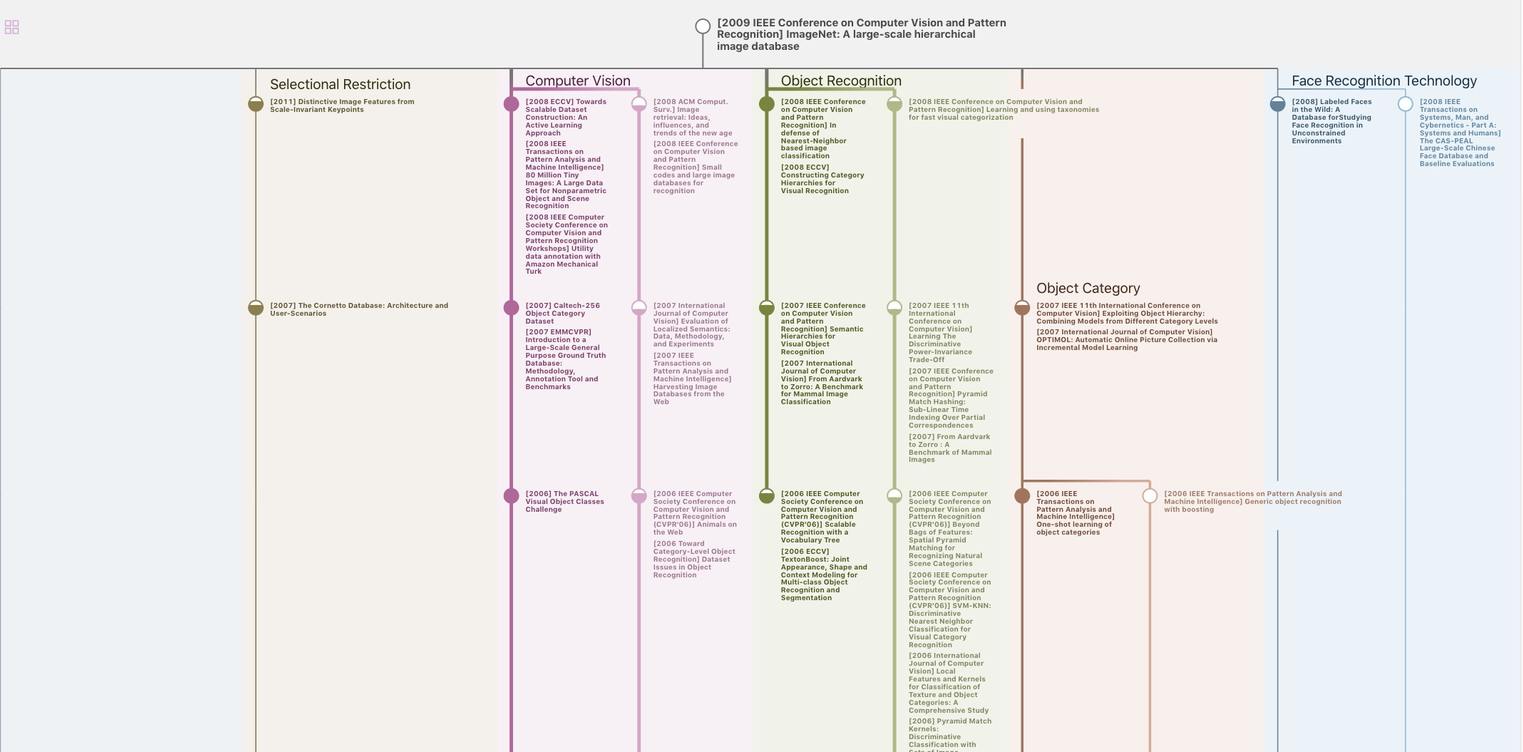
生成溯源树,研究论文发展脉络
Chat Paper
正在生成论文摘要