Accelerating the Discovery of Transition Metal Borides by Machine Learning on Small Data Sets.
ACS applied materials & interfaces(2023)
摘要
Accurate and efficient prediction of the stability and structure-stability relationship is important to discover materials; however, it requires tremendous efforts via traditional trial-and-error schemes. Here, we presented a small-data set machine learning (ML) method to accelerate the discovery of promising ternary transition metal boride (MAB) candidates. Based on data sets obtained by ab initio calculations, we developed three robust neural networks to predict the decomposition energy (Δ) and assess the thermodynamic stability of 212-typed MABs (MAB). The quantitative relation between Δ and stability was unraveled by several composition-and-structure descriptors. Three hexagonal MAB, i.e., NbPB, NbAsB, and ZrSB, were discovered to be stable with negative Δ, and 75 metastable MABs were identified with Δ less than 70 meV/atom. Finally, the dynamical stability and mechanical properties of MABs were investigated by ab initio calculations, whose results further verified the reliability of our ML models. This work provided a ML approach on small data sets to accelerate the discovery of compounds and expanded the MAB phase family to V and VI groups.
更多查看译文
关键词
MAB phases, stability, machine learning, small data sets, ab initio calculations
AI 理解论文
溯源树
样例
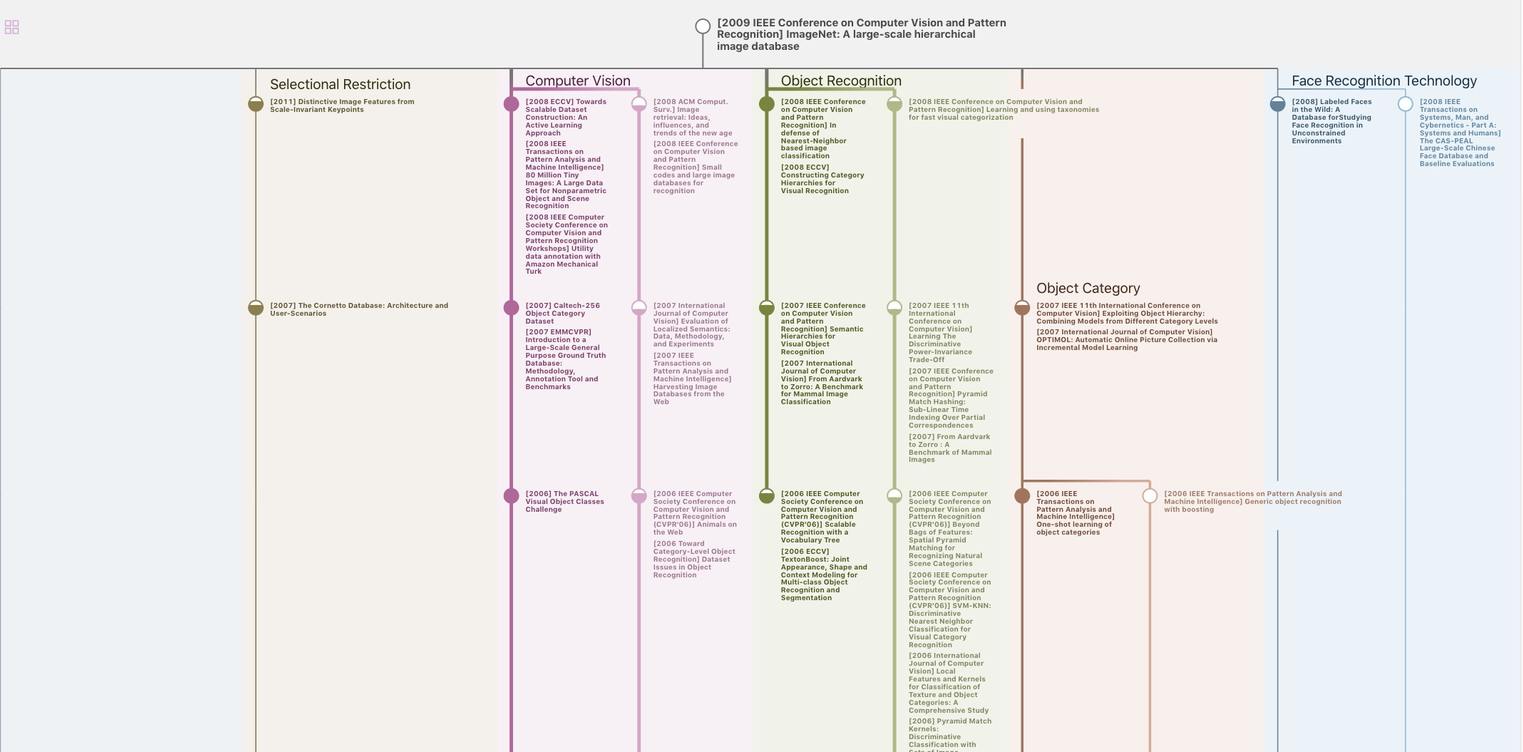
生成溯源树,研究论文发展脉络
Chat Paper
正在生成论文摘要