Deep-learning CT reconstruction in clinical scans of the abdomen: a systematic review and meta-analysis
Abdominal radiology (New York)(2023)
摘要
Objective To perform a systematic literature review and meta-analysis of the two most common commercially available deep-learning algorithms for CT. Methods We used PubMed, Scopus, Embase, and Web of Science to conduct systematic searches for studies assessing the most common commercially available deep-learning CT reconstruction algorithms: True Fidelity (TF) and Advanced intelligent Clear-IQ Engine (AiCE) in the abdomen of human participants since only these two algorithms currently have adequate published data for robust systematic analysis. Results Forty-four articles fulfilled inclusion criteria. 32 studies evaluated TF and 12 studies assessed AiCE. DLR algorithms produced images with significantly less noise (22–57.3% less than IR) but preserved a desirable noise texture with increased contrast-to-noise ratios and improved lesion detectability on conventional CT. These improvements with DLR were similarly noted in dual-energy CT which was only assessed for a single vendor. Reported radiation reduction potential was 35.1–78.5%. Nine studies assessed observer performance with the two dedicated liver lesion studies being performed on the same vendor reconstruction (TF). These two studies indicate preserved low contrast liver lesion detection (> 5 mm) at CTDI vol 6.8 mGy (BMI 23.5 kg/m 2 ) to 12.2 mGy (BMI 29 kg/m 2 ). If smaller lesion detection and improved lesion characterization is needed, a CTDI vol of 13.6–34.9 mGy is needed in a normal weight to obese population. Mild signal loss and blurring have been reported at high DLR reconstruction strengths. Conclusion Deep learning reconstructions significantly improve image quality in CT of the abdomen. Assessment of other dose levels and clinical indications is needed. Careful choice of radiation dose levels is necessary, particularly for small liver lesion assessment. Graphical abstract
更多查看译文
关键词
CT,Image reconstruction,Convolutional neural network,Deep learning,Artificial intelligence,Dose reduction,Abdomen
AI 理解论文
溯源树
样例
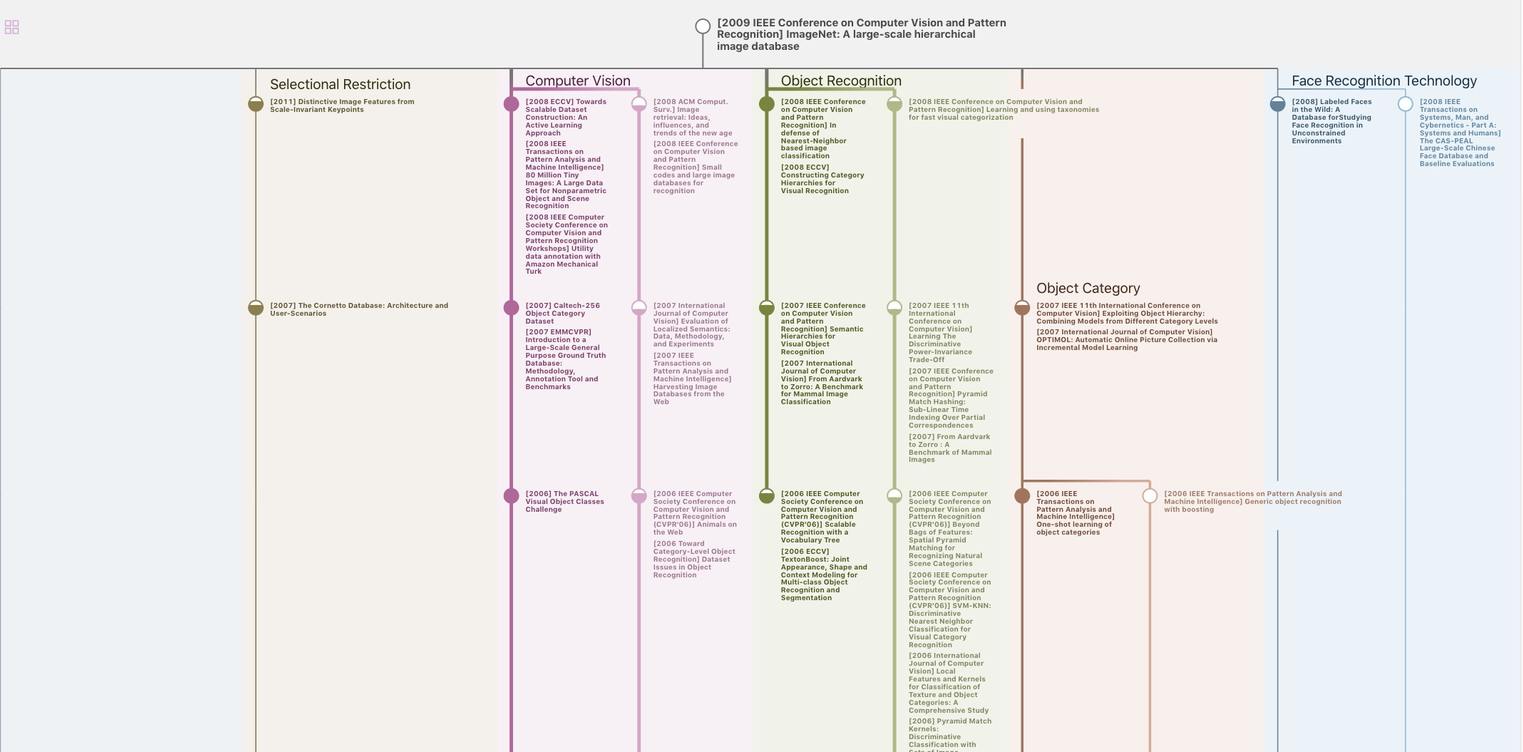
生成溯源树,研究论文发展脉络
Chat Paper
正在生成论文摘要