NeRF-Art: Text-Driven Neural Radiance Fields Stylization.
IEEE transactions on visualization and computer graphics(2023)
摘要
As a powerful representation of 3D scenes, the neural radiance field (NeRF) enables high-quality novel view synthesis from multi-view images. Stylizing NeRF, however, remains challenging, especially in simulating a text-guided style with both the appearance and the geometry altered simultaneously. In this paper, we present NeRF-Art, a text-guided NeRF stylization approach that manipulates the style of a pre-trained NeRF model with a simple text prompt. Unlike previous approaches that either lack sufficient geometry deformations and texture details or require meshes to guide the stylization, our method can shift a 3D scene to the target style characterized by desired geometry and appearance variations without any mesh guidance. This is achieved by introducing a novel global-local contrastive learning strategy, combined with the directional constraint to simultaneously control both the trajectory and the strength of the target style. Moreover, we adopt a weight regularization method to effectively suppress cloudy artifacts and geometry noises which arise easily when the density field is transformed during geometry stylization. Through extensive experiments on various styles, we demonstrate that our method is effective and robust regarding both single-view stylization quality and cross-view consistency. The code and more results can be found on our project page: https://cassiepython.github.io/nerfart/.
更多查看译文
关键词
Text-Driven Stylization,Neural Radiance Fields,CLIP
AI 理解论文
溯源树
样例
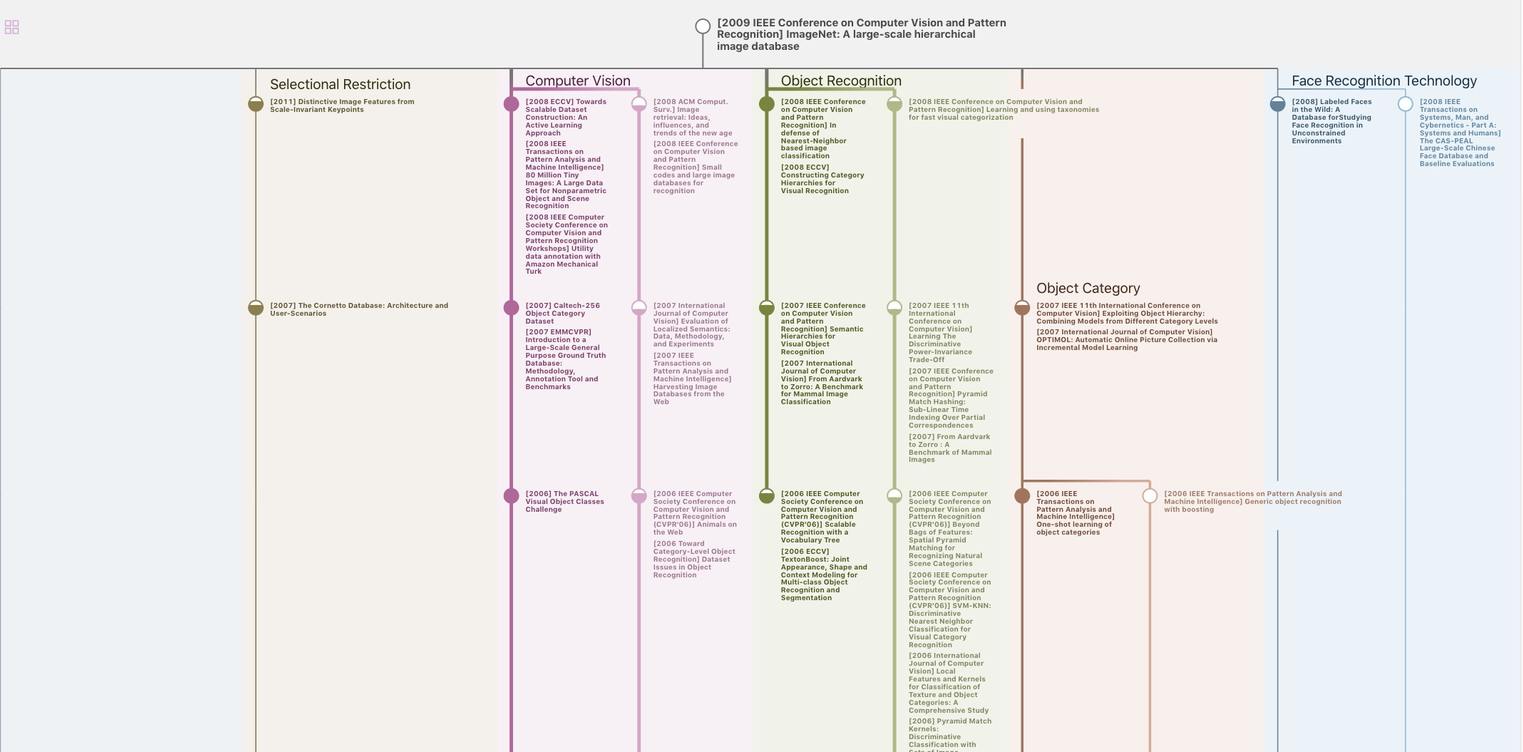
生成溯源树,研究论文发展脉络
Chat Paper
正在生成论文摘要