S N Ar Regioselectivity Predictions: Machine Learning Triggering DFT Reaction Modeling through Statistical Threshold.
Journal of chemical information and modeling(2023)
摘要
Fast and accurate prospective predictions of regioselectivity can significantly reduce the time and resources spent on unproductive transformations in the pharmaceutical industry. Density functional theory (DFT) reaction modeling through transition state theory (TST) and machine learning (ML) methods has been widely used to predict reaction outcomes such as selectivity. However, TST reaction modeling and ML methods are either time-consuming or data-dependent. Herein, we introduce a prototype seamlessly bridging ML and TST modeling by triggering resource-intensive but much less domain-sensitive DFT calculations only on less confident ML predictions. The proposed workflow was trained and tested on both the Pfizer internal dataset and the USPTO public dataset to predict regioselectivity for SAr reactions. Our method is accurate and fast, which achieves 96.3 and 94.7% accuracy in predicting the correct major product on Pfizer and USPTO datasets, respectively, in a fraction of conventional TST computing time.
更多查看译文
AI 理解论文
溯源树
样例
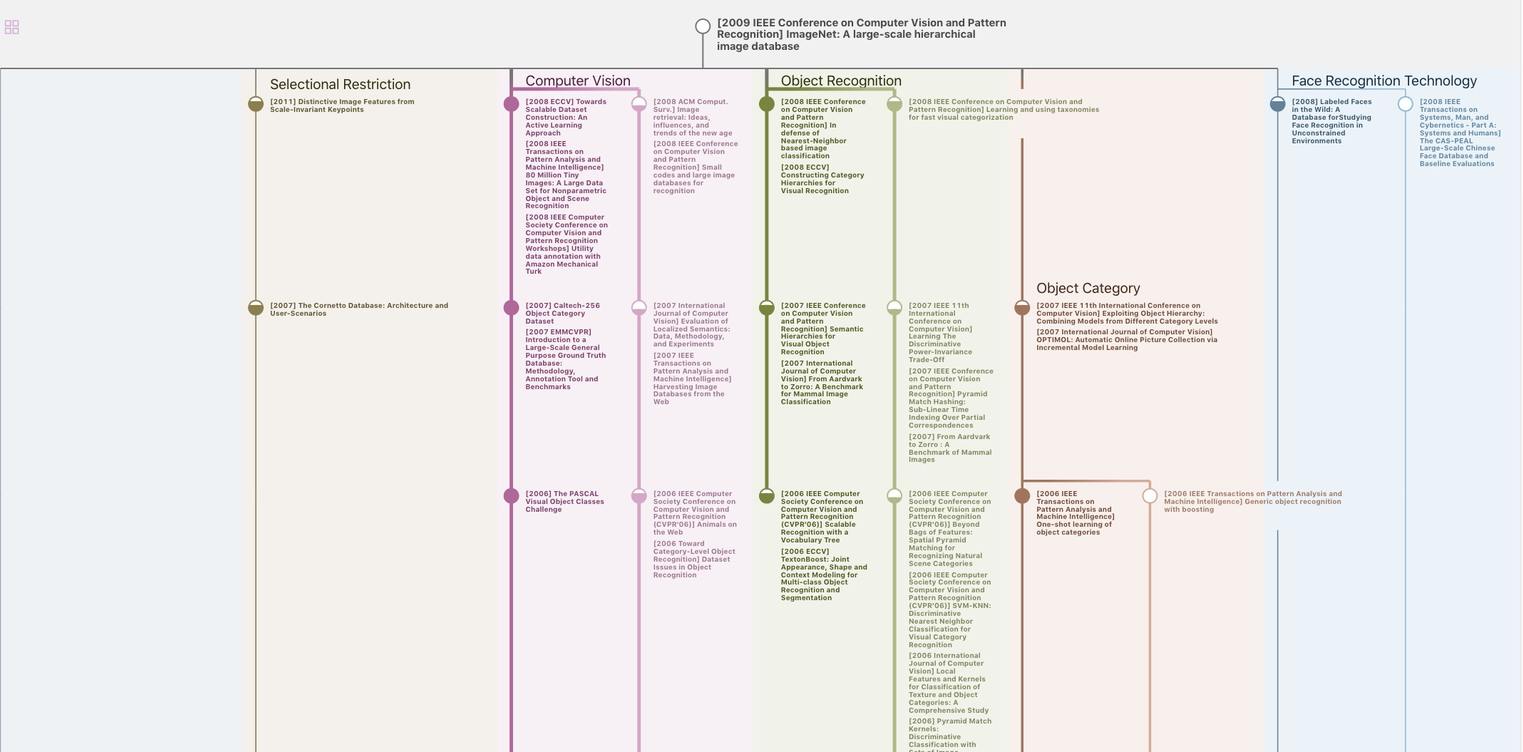
生成溯源树,研究论文发展脉络
Chat Paper
正在生成论文摘要