Efficient Fully-Offline Meta-Reinforcement Learning via Distance Metric Learning and Behavior Regularization
international conference on learning representations(2021)
摘要
We study the offline meta-reinforcement learning (OMRL) problem, a paradigm which enables reinforcement learning (RL) algorithms to quickly adapt to unseen tasks without any interactions with the environments, making RL truly practical in many real-world applications. This problem is still not fully understood, for which two major challenges need to be addressed. First, offline RL often suffers from bootstrapping errors of out-of-distribution state-actions which leads to divergence of value functions. Second, meta-RL requires efficient and robust task inference learned jointly with control policy. In this work, we enforce behavior regularization on learned policy as a general approach to offline RL, combined with a deterministic context encoder for efficient task inference. We propose a novel negative-power distance metric on bounded context embedding space, whose gradients propagation is detached from that of the Bellman backup. We provide analysis and insight showing that some simple design choices can yield substantial improvements over recent approaches involving meta-RL and distance metric learning. To the best of our knowledge, our method is the first model-free and end-to-end OMRL algorithm, which is computationally efficient and demonstrated to outperform prior algorithms on several meta-RL benchmarks.
更多查看译文
关键词
distance metric learning,fully-offline,meta-reinforcement
AI 理解论文
溯源树
样例
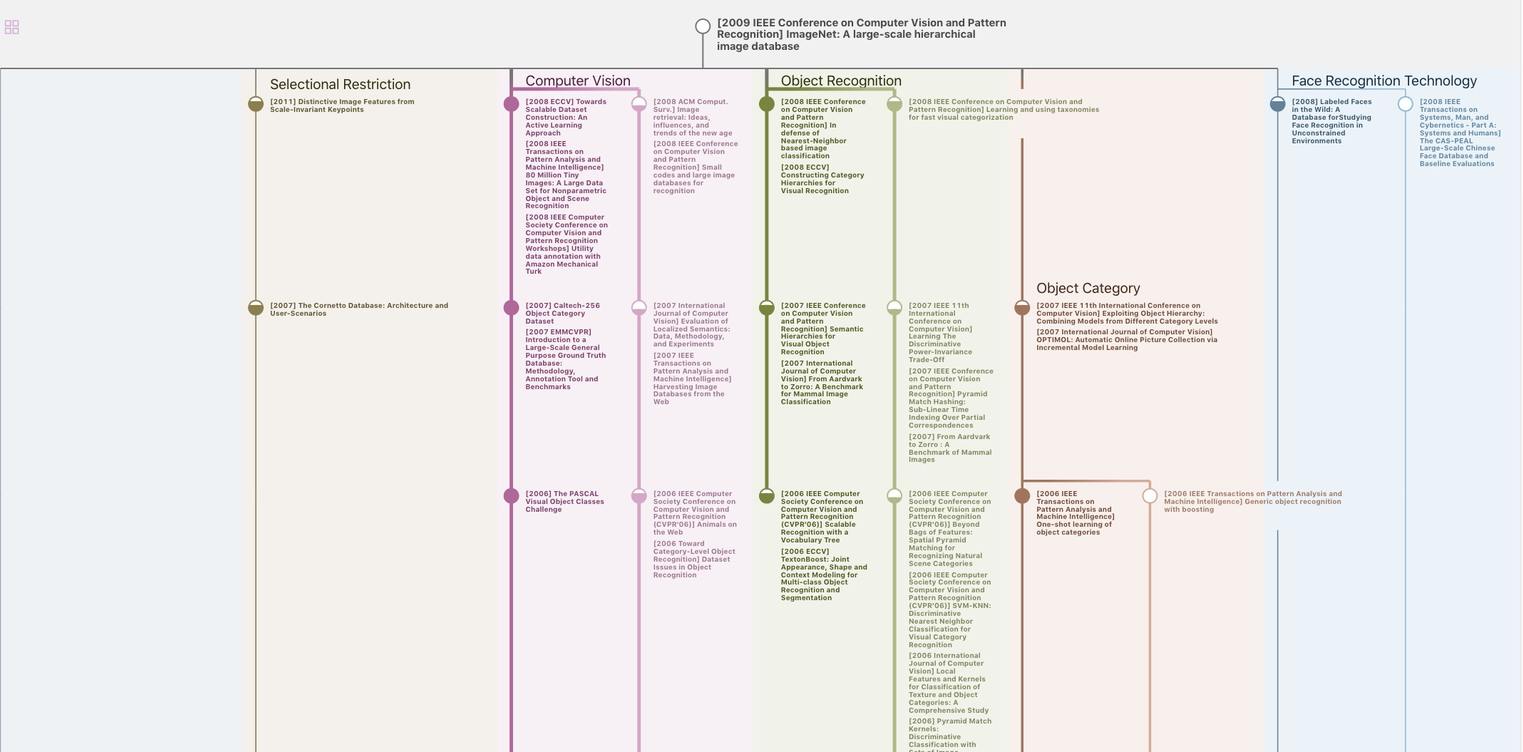
生成溯源树,研究论文发展脉络
Chat Paper
正在生成论文摘要