Multinomial Logistic Factor Regression for Multi-source Functional Block-wise Missing Data
Psychometrika(2023)
摘要
Multi-source functional block-wise missing data arise more commonly in medical care recently with the rapid development of big data and medical technology, hence there is an urgent need to develop efficient dimension reduction to extract important information for classification under such data. However, most existing methods for classification problems consider high-dimensional data as covariates. In the paper, we propose a novel multinomial imputed-factor Logistic regression model with multi-source functional block-wise missing data as covariates. Our main contribution is to establishing two multinomial factor regression models by using the imputed multi-source functional principal component scores and imputed canonical scores as covariates, respectively, where the missing factors are imputed by both the conditional mean imputation and the multiple block-wise imputation approaches. Specifically, the univariate FPCA is carried out for the observable data of each data source firstly to obtain the univariate principal component scores and the eigenfunctions. Then, the block-wise missing univariate principal component scores instead of the block-wise missing functional data are imputed by the conditional mean imputation method and the multiple block-wise imputation method, respectively. After that, based on the imputed univariate factors, the multi-source principal component scores are constructed by using the relationship between the multi-source principal component scores and the univariate principal component scores; and at the same time, the canonical scores are obtained by the multiple-set canonial correlation analysis. Finally, the multinomial imputed-factor Logistic regression model is established with the multi-source principal component scores or the canonical scores as factors. Numerical simulations and real data analysis on ADNI data show the proposed method works well.
更多查看译文
关键词
Multi-source functional block-wise missing data,Multi-source functional principal component analysis (MFPCA),Multi-source principal component scores,Multiple-set canonical correlation analysis (MCCA),Canonical scores,Conditional mean imputation,Multiple block-wise imputation,Multinomial Logistic factor regression model,ADNI
AI 理解论文
溯源树
样例
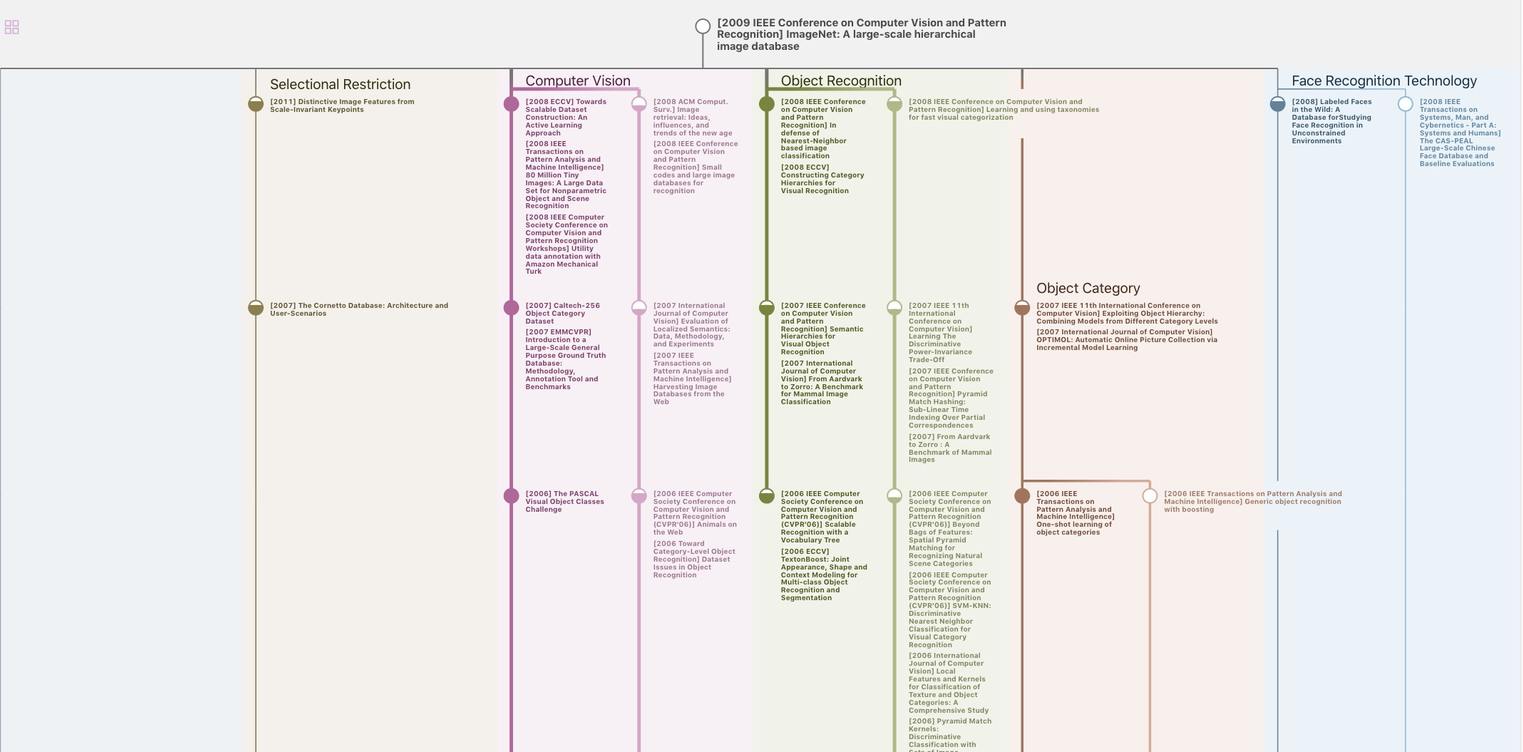
生成溯源树,研究论文发展脉络
Chat Paper
正在生成论文摘要