Intra-Patient Lung CT Registration through Large Deformation Decomposition and Attention-Guided Refinement.
Bioengineering (Basel, Switzerland)(2023)
摘要
Deformable lung CT image registration is an essential task for computer-assisted interventions and other clinical applications, especially when organ motion is involved. While deep-learning-based image registration methods have recently achieved promising results by inferring deformation fields in an end-to-end manner, large and irregular deformations caused by organ motion still pose a significant challenge. In this paper, we present a method for registering lung CT images that is tailored to the specific patient being imaged. To address the challenge of large deformations between the source and target images, we break the deformation down into multiple continuous intermediate fields. These fields are then combined to create a spatio-temporal motion field. We further refine this field using a self-attention layer that aggregates information along motion trajectories. By leveraging temporal information from a respiratory cycle, our proposed methods can generate intermediate images that facilitate image-guided tumor tracking. We evaluated our approach extensively on a public dataset, and our numerical and visual results demonstrate the effectiveness of the proposed method.
更多查看译文
关键词
large deformation decomposition,lung,ct,refinement,intra-patient,attention-guided
AI 理解论文
溯源树
样例
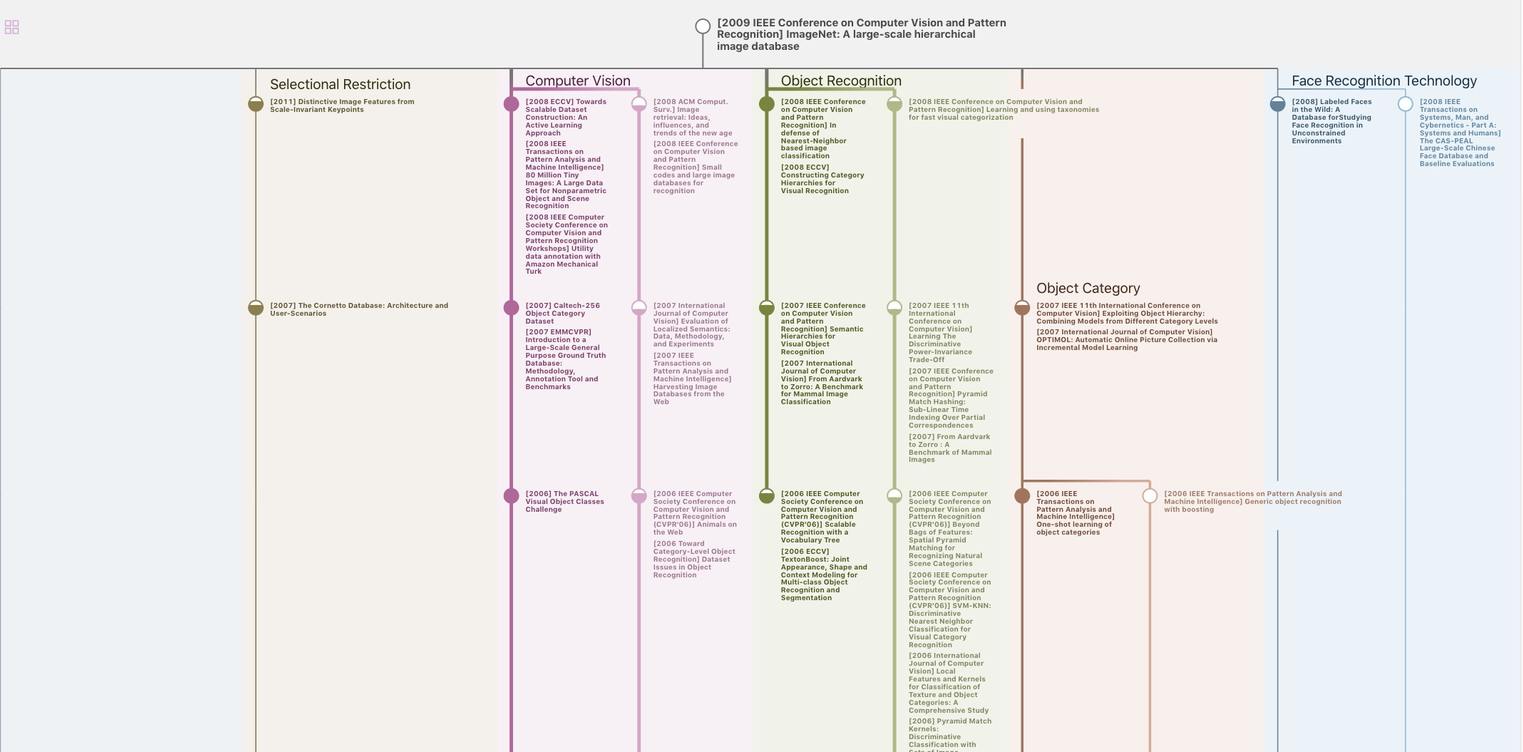
生成溯源树,研究论文发展脉络
Chat Paper
正在生成论文摘要