Improved Differential Diagnosis Based on BI-RADS Descriptors and Apparent Diffusion Coefficient for Breast Lesions: A Multiparametric MRI Analysis As Compared to Kaiser Score.
Academic Radiology(2023)
关键词
MRI,Kaiser score,Breast cancer,Nomogram,Breast biopsies
AI 理解论文
溯源树
样例
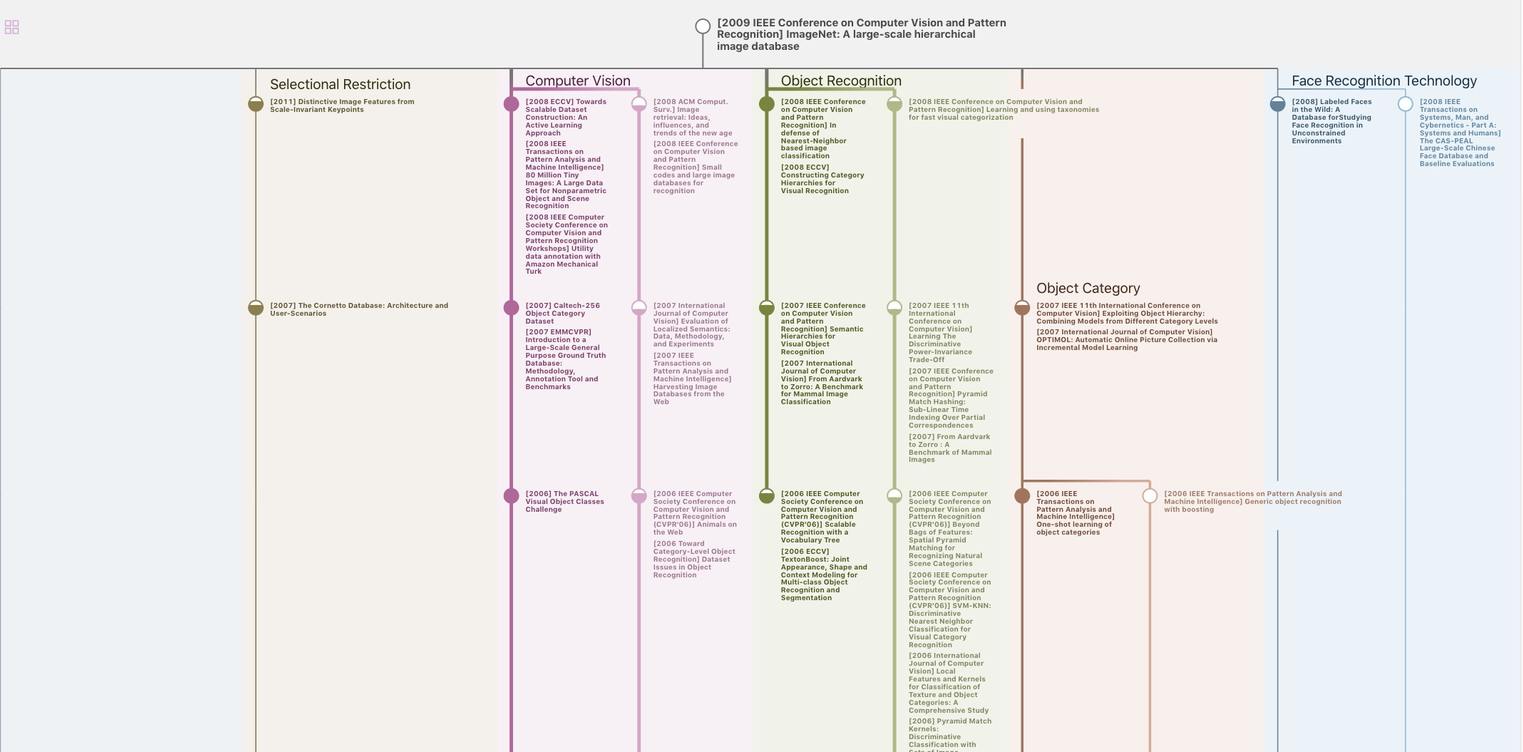
生成溯源树,研究论文发展脉络
Chat Paper
正在生成论文摘要