ADEPT: Autoencoder with differentially expressed genes and imputation for robust spatial transcriptomics clustering.
iScience(2023)
摘要
Advancements in spatial transcriptomics (ST) have enabled an in-depth understanding of complex tissues by quantifying gene expression at spatially localized spots. Several notable clustering methods have been introduced to utilize both spatial and transcriptional information in the analysis of ST datasets. However, data quality across different ST sequencing techniques and types of datasets influence the performance of different methods and benchmarks. To harness spatial context and transcriptional profile in ST data, we developed a graph-based, multi-stage framework for robust clustering, called ADEPT. To control and stabilize data quality, ADEPT relies on a graph autoencoder backbone and performs an iterative clustering on imputed, differentially expressed genes-based matrices to minimize the variance of clustering results. ADEPT outperformed other popular methods on ST data generated by different platforms across analyses such as spatial domain identification, visualization, spatial trajectory inference, and data denoising.
更多查看译文
关键词
Automation in bioinformatics,Data processing in systems biology,Transcriptomics
AI 理解论文
溯源树
样例
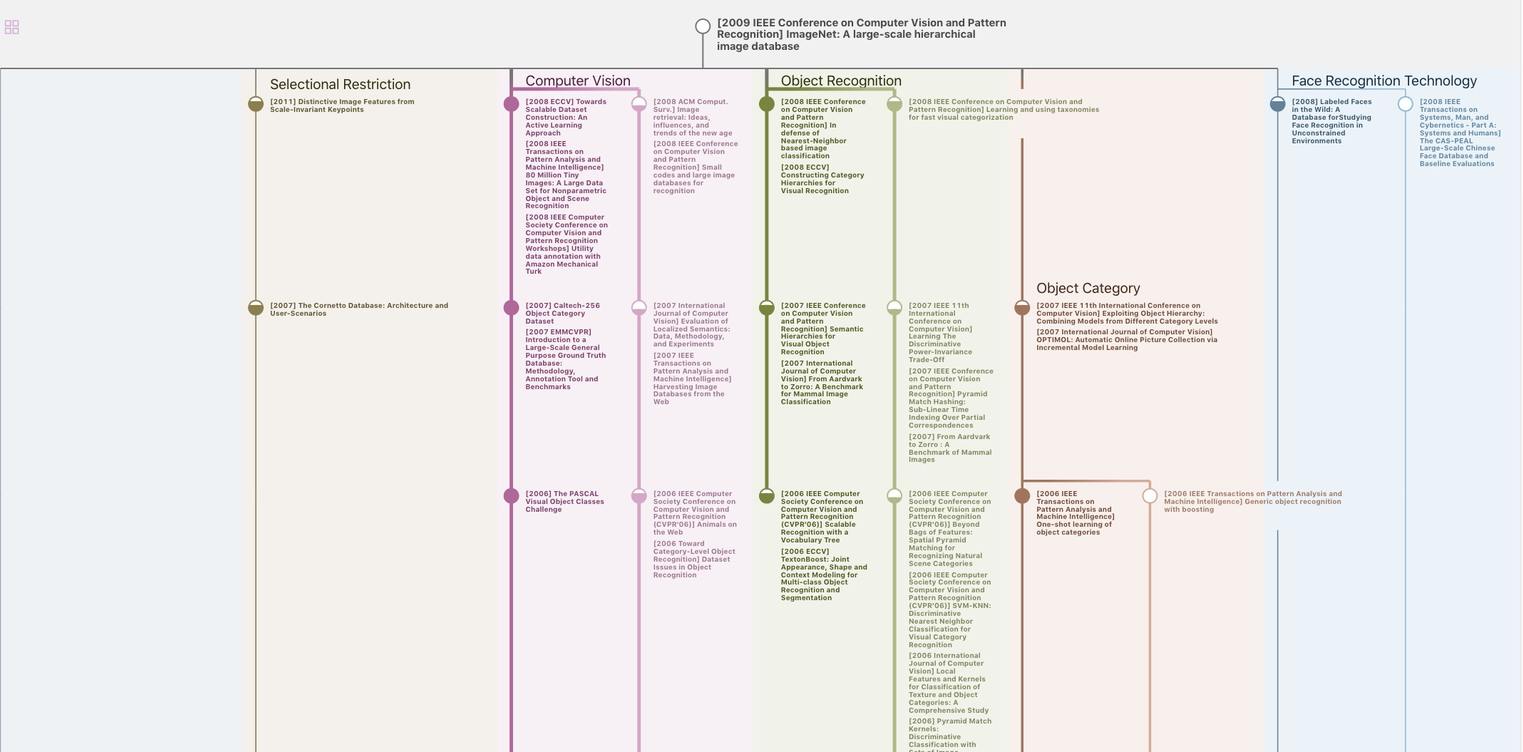
生成溯源树,研究论文发展脉络
Chat Paper
正在生成论文摘要