X-ray image decomposition for improved magnetic navigation
International journal of computer assisted radiology and surgery(2023)
摘要
Purpose Existing field generators (FGs) for magnetic tracking cause severe image artifacts in X-ray images. While FG with radio-lucent components significantly reduces these imaging artifacts, traces of coils and electronic components may still be visible to trained professionals. In the context of X-ray-guided interventions using magnetic tracking, we introduce a learning-based approach to further reduce traces of field-generator components from X-ray images to improve visualization and image guidance. Methods An adversarial decomposition network was trained to separate the residual FG components (including fiducial points introduced for pose estimation), from the X-ray images. The main novelty of our approach lies in the proposed data synthesis method, which combines existing 2D patient chest X-ray and FG X-ray images to generate 20,000 synthetic images, along with ground truth (images without the FG) to effectively train the network. Results For 30 real images of a torso phantom, our enhanced X-ray image after image decomposition obtained an average local PSNR of 35.04 and local SSIM of 0.97, whereas the unenhanced X-ray images averaged a local PSNR of 31.16 and local SSIM of 0.96. Conclusion In this study, we proposed an X-ray image decomposition method to enhance X-ray image for magnetic navigation by removing FG-induced artifacts, using a generative adversarial network. Experiments on both synthetic and real phantom data demonstrated the efficacy of our method.
更多查看译文
关键词
X-ray simulation,Magnetic tracking,X-ray-guided interventions,Image decomposition
AI 理解论文
溯源树
样例
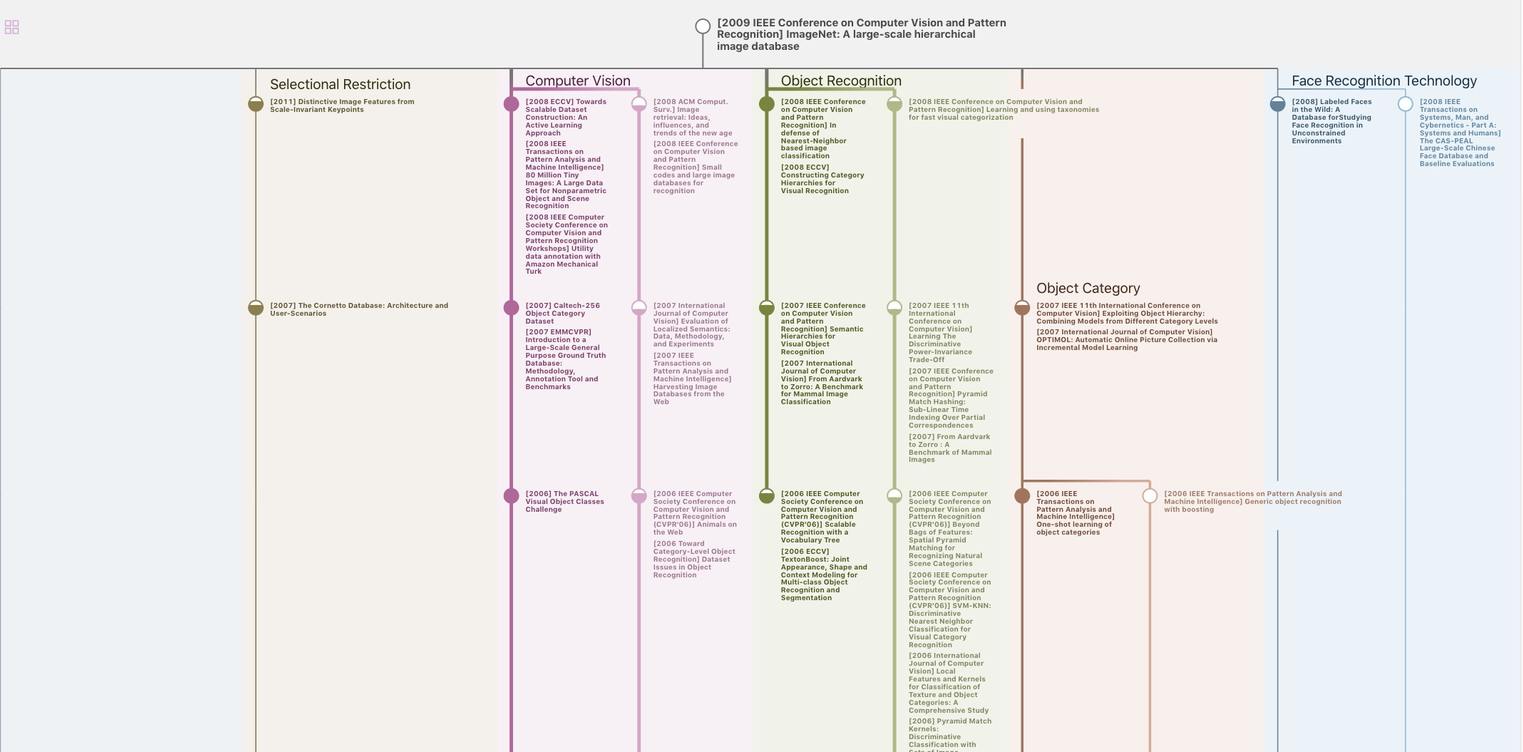
生成溯源树,研究论文发展脉络
Chat Paper
正在生成论文摘要