Development of a deep-learning model for classification of LI-RADS major features by using subtraction images of MRI: a preliminary study
Abdominal radiology (New York)(2023)
摘要
Purpose Liver Imaging Reporting and Data System (LI-RADS) is limited by interreader variability. Thus, our study aimed to develop a deep-learning model for classifying LI-RADS major features using subtraction images using magnetic resonance imaging (MRI). Methods This single-center retrospective study included 222 consecutive patients who underwent resection for hepatocellular carcinoma (HCC) between January, 2015 and December, 2017. Subtraction arterial, portal venous, and transitional phase images of preoperative gadoxetic acid-enhanced MRI were used to train and test the deep-learning models. Initially, a three-dimensional (3D) nnU-Net-based deep-learning model was developed for HCC segmentation. Subsequently, a 3D U-Net-based deep-learning model was developed to assess three LI-RADS major features (nonrim arterial phase hyperenhancement [APHE], nonperipheral washout, and enhancing capsule [EC]), utilizing the results determined by board-certified radiologists as reference standards. The HCC segmentation performance was assessed using the Dice similarity coefficient (DSC), sensitivity, and precision. The sensitivity, specificity, and accuracy of the deep-learning model for classifying LI-RADS major features were calculated. Results The average DSC, sensitivity, and precision of our model for HCC segmentation were 0.884, 0.891, and 0.887, respectively, across all the phases. Our model demonstrated a sensitivity, specificity, and accuracy of 96.6% (28/29), 66.7% (4/6), and 91.4% (32/35), respectively, for nonrim APHE; 95.0% (19/20), 50.0% (4/8), and 82.1% (23/28), respectively, for nonperipheral washout; and 86.7% (26/30), 54.2% (13/24), and 72.2% (39/54) for EC, respectively. Conclusion We developed an end-to-end deep-learning model that classifies the LI-RADS major features using subtraction MRI images. Our model exhibited satisfactory performance in classifying LI-RADS major features. Graphical Abstract
更多查看译文
关键词
mri,deep-learning deep-learning,subtraction images,li-rads
AI 理解论文
溯源树
样例
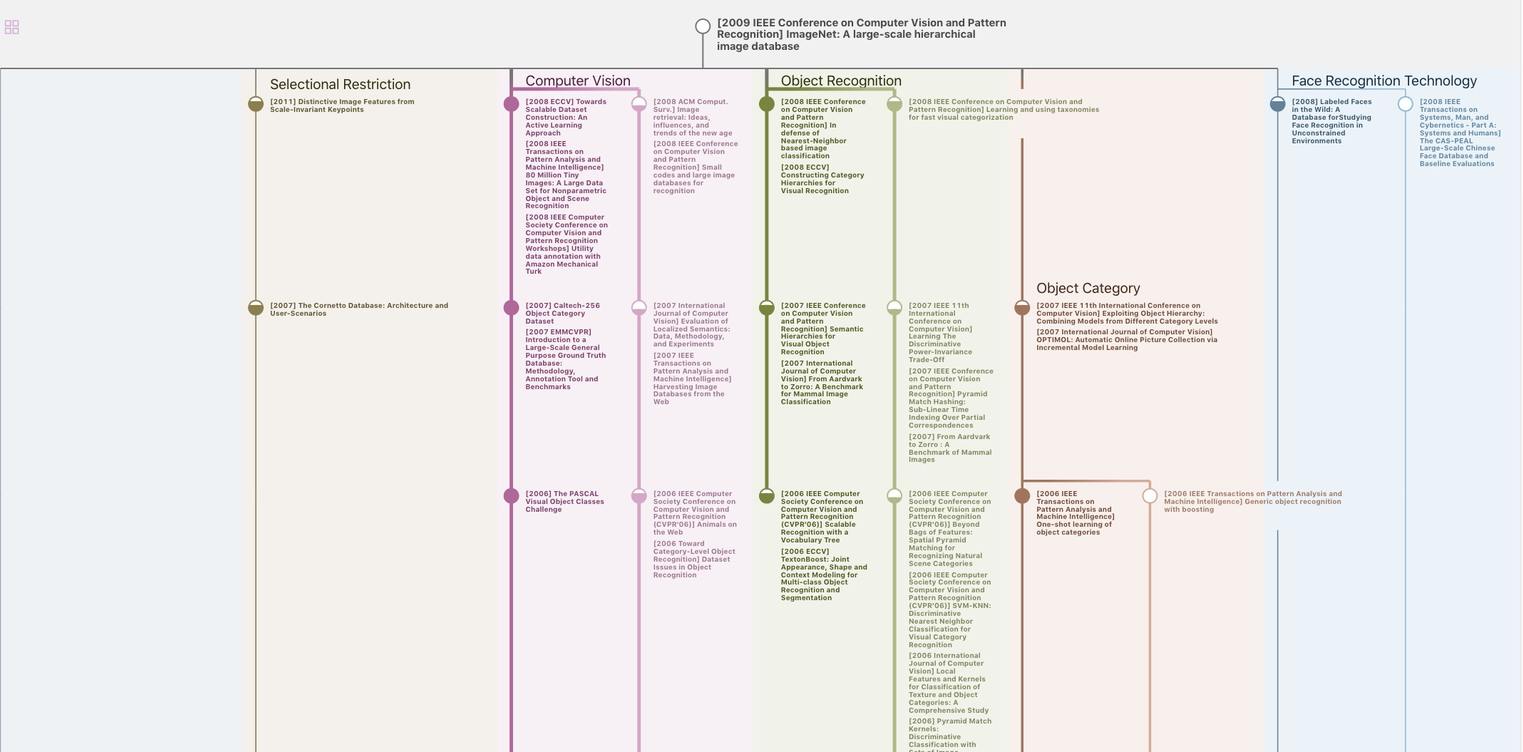
生成溯源树,研究论文发展脉络
Chat Paper
正在生成论文摘要