Adaptively leveraging external data with robust meta-analytical-predictive prior using empirical Bayes.
Pharmaceutical statistics(2023)
摘要
The robust meta-analytical-predictive (rMAP) prior is a popular method to robustly leverage external data. However, a mixture coefficient would need to be pre-specified based on the anticipated level of prior-data conflict. This can be very challenging at the study design stage. We propose a novel empirical Bayes robust MAP (EB-rMAP) prior to address this practical need and adaptively leverage external/historical data. Built on Box's prior predictive p-value, the EB-rMAP prior framework balances between model parsimony and flexibility through a tuning parameter. The proposed framework can be applied to binomial, normal, and time-to-event endpoints. Implementation of the EB-rMAP prior is also computationally efficient. Simulation results demonstrate that the EB-rMAP prior is robust in the presence of prior-data conflict while preserving statistical power. The proposed EB-rMAP prior is then applied to a clinical dataset that comprises 10 oncology clinical trials, including the prospective study.
更多查看译文
关键词
empirical Bayes,meta-analytical-predictive prior,prior-data conflict,robustness
AI 理解论文
溯源树
样例
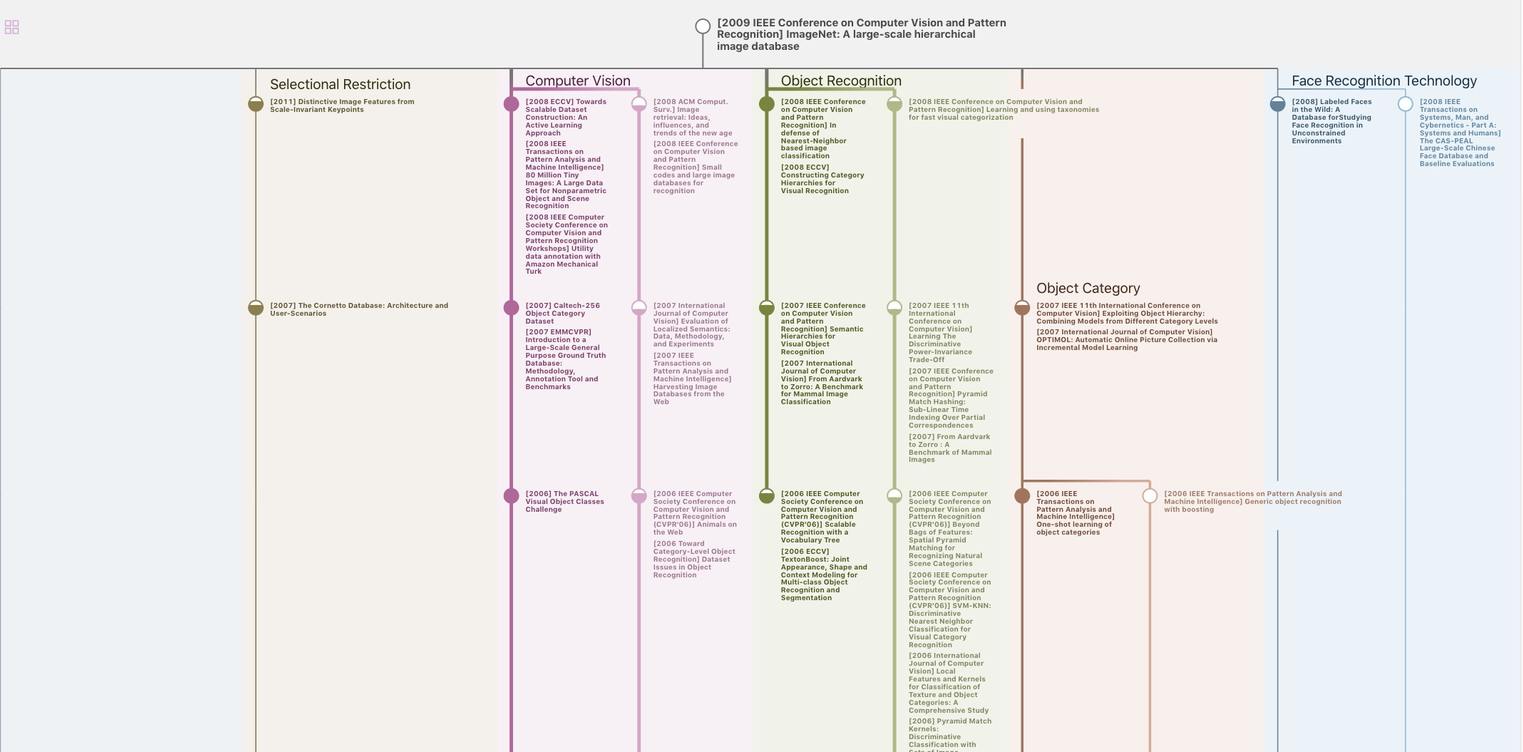
生成溯源树,研究论文发展脉络
Chat Paper
正在生成论文摘要