Coarse-Refined Consistency Learning Using Pixel-Level Features for Semi-Supervised Medical Image Segmentation.
IEEE journal of biomedical and health informatics(2023)
摘要
Pixel-level annotations are extremely expensive for medical image segmentation tasks as both expertise and time are needed to generate accurate annotations. Semi-supervised learning (SSL) for medical image segmentation has recently attracted growing attention because it can alleviate the exhausting manual annotations for clinicians by leveraging unlabeled data. However, most of the existing SSL methods do not take pixel-level information (e.g., pixel-level features) of labeled data into account, i.e., the labeled data are underutilized. Hence, in this work, an innovative Coarse-Refined Network with pixel-wise Intra-patch ranked loss and patch-wise Inter-patch ranked loss (CRII-Net) is proposed. It provides three advantages: i) it can produce stable targets for unlabeled data, as a simple yet effective coarse-refined consistency constraint is designed; ii) it is very effective for the extreme case where very scarce labeled data are available, as the pixel-level and patch-level features are extracted by our CRII-Net; and iii) it can output fine-grained segmentation results for hard regions (e.g., blurred object boundaries and low-contrast lesions), as the proposed Intra-Patch Ranked Loss (Intra-PRL) focuses on object boundaries and Inter-Patch Ranked loss (Inter-PRL) mitigates the adverse impact of low-contrast lesions. Experimental results on two common SSL tasks for medical image segmentation demonstrate the superiority of our CRII-Net. Specifically, when there are only 4% labeled data, our CRII-Net improves the Dice similarity coefficient (DSC) score by at least 7.49% when compared to five classical or state-of-the-art (SOTA) SSL methods. For hard samples/regions, our CRII-Net also significantly outperforms other compared methods in both quantitative and visualization results.
更多查看译文
关键词
segmentation,consistency,coarse-refined,pixel-level,semi-supervised
AI 理解论文
溯源树
样例
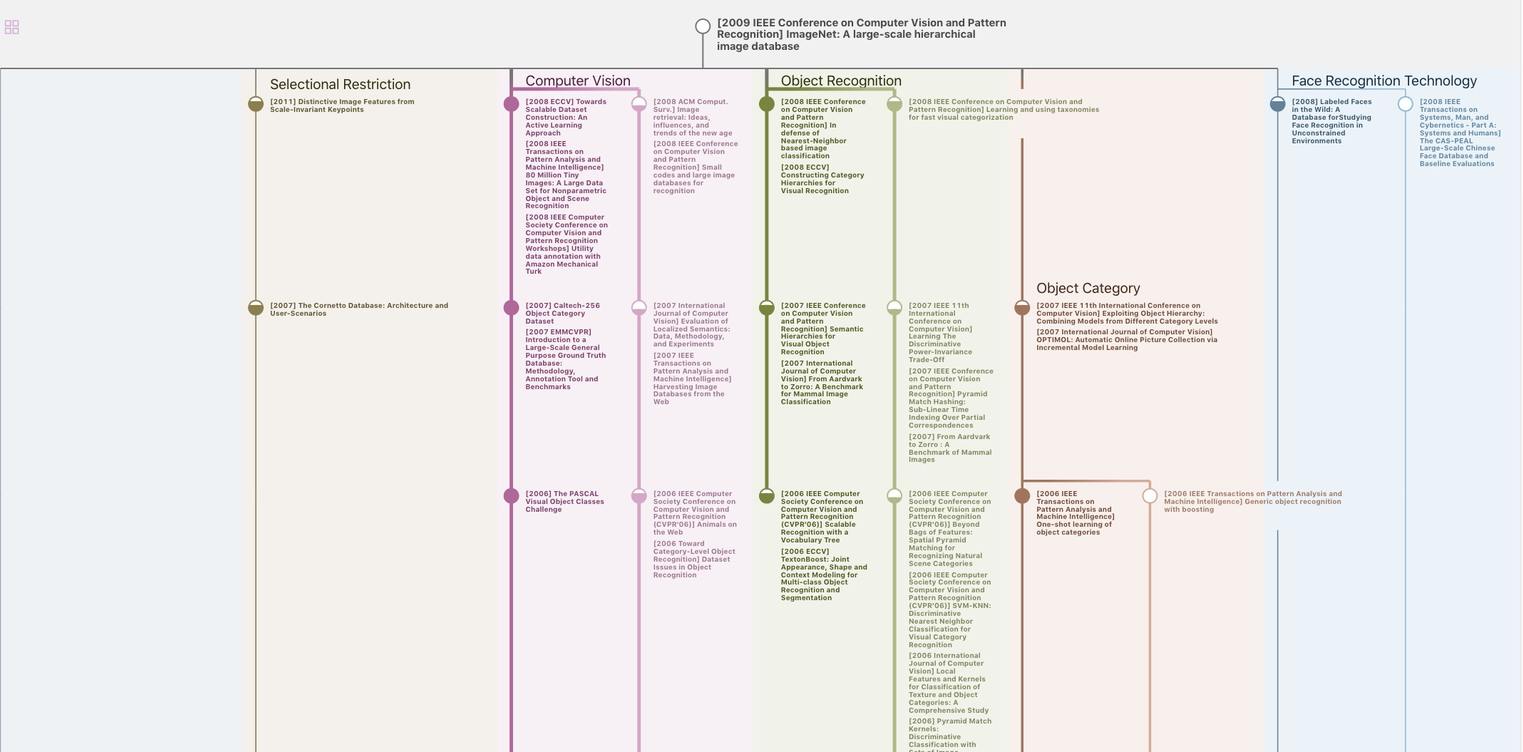
生成溯源树,研究论文发展脉络
Chat Paper
正在生成论文摘要