Can Radiomics Bridge the Gap Between Immunotherapy and Precision Medicine in Lung Cancer?
Journal of thoracic oncology : official publication of the International Association for the Study of Lung Cancer(2023)
摘要
Immunotherapy has ushered in a new era of cancer therapeutics by exhibiting unprecedented rates of durable responses even in traditionally difficult-to-treat malignancies such as lung cancer. Whereas advances in genomic profiling have significantly impacted therapeutic strategies for patients with oncogene-driven tumors, there has been a relative lag in progressive techniques for optimizing treatment with immunotherapy. Biomarker discovery for immunotherapy is fraught with challenges because of our incomplete understanding of tumor intrinsic or extrinsic factors that impact responses, the dynamic nature of the tumor immune milieu, and the lack of uniform integration of biomarkers in clinical trials. Therefore, there seems to be an insidious shift back toward an “all-inclusive” management approach in patients with advanced NSCLC without a driver mutation. Albeit imperfect, programmed cell death ligand 1 (PD-L1) is the most predictive biomarker for immunotherapy in lung cancer to date. Because tumor PD-L1 expression is heterogeneous, dynamic, and often discordant between sites of metastatic disease; a noninvasive tool to predict PD-L1 expression could prove to be invaluable to overcome some of these limitations. There is a wealth of unexploited data available from computed tomography (CT) and positron emission tomography images in patients treated with immunotherapy. In this month’s issue of the Journal of Thoracic Oncology, Chen et al.1Chen M. Lu H. Copley S.J. et al.A novel radiogenomics biomarker for predicting treatment response and pneumotoxicity from programmed cell death protein or ligand-1 inhibition immunotherapy in NSCLC.J Thorac Oncol. 2023; 18: 718-730Abstract Full Text Full Text PDF Scopus (1) Google Scholar address an important clinical concept: could this radiographic data be mined to discover predictive and prognostic biomarkers that would allow more precise allocation of immunotherapeutic agents in patients with advanced lung cancer? Radiomics refers to the high-throughput extraction of large amounts of quantitative features from radiographic images, a method used to improve analysis of medical images beyond what can be observed by the human eye.2Lambin P. Rios-Velazquez E. Leijenaar R. et al.Radiomics: extracting more information from medical images using advanced feature analysis.Eur J Cancer. 2012; 48: 441-446Abstract Full Text Full Text PDF PubMed Scopus (3002) Google Scholar,3Viswanathan V.S. Gupta A. Madabhushi A. Novel imaging biomarkers to assess oncologic treatment–related changes.Am Soc Clin Oncol Educ Book. 2022; 42: 1-13PubMed Google Scholar Using automatic or semiautomatic software, quantitative analysis of radiographic images can provide relevant information about differences in tumor shape, texture, and the surrounding environment.2Lambin P. Rios-Velazquez E. Leijenaar R. et al.Radiomics: extracting more information from medical images using advanced feature analysis.Eur J Cancer. 2012; 48: 441-446Abstract Full Text Full Text PDF PubMed Scopus (3002) Google Scholar,4Thawani R. McLane M. Beig N. et al.Radiomics and radiogenomics in lung cancer: a review for the clinician.Lung Cancer. 2018; 115: 34-41Abstract Full Text Full Text PDF PubMed Scopus (286) Google Scholar In recent years, there has been mounting interest in exploring the use of radiomics in assessing treatment-related changes, the risk for toxicity, and predicting response to treatment.5Bera K. Braman N. Gupta A. Velcheti V. Madabhushi A. Predicting cancer outcomes with radiomics and artificial intelligence in radiology.Nat Rev Clin Oncol. 2022; 19: 132-146Crossref PubMed Scopus (79) Google Scholar,6Bera K. Velcheti V. Madabhushi A. Novel quantitative imaging for predicting response to therapy: techniques and clinical applications.Am Soc Clin Oncol Educ Book. 2018; 38: 1008-1018Crossref PubMed Scopus (41) Google Scholar Moreover, artificial intelligence and machine learning tools used in digital pathology can mine subvisual morphometric phenotypes. A multiomics approach by integrating such data with radiomic algorithms has the potential to not only provide insight into the biological underpinnings of informative radiomic features but also improve the estimation of patient outcomes.7Bera K. Schalper K.A. Rimm D.L. Velcheti V. Madabhushi A. Artificial intelligence in digital pathology - new tools for diagnosis and precision oncology.Nat Rev Clin Oncol. 2019; 16: 703-715Crossref PubMed Scopus (485) Google Scholar Through methods of radiomic feature extraction and analysis from CT images of patients with advanced NSCLC treated with immune checkpoint inhibitors, Chen et al.1Chen M. Lu H. Copley S.J. et al.A novel radiogenomics biomarker for predicting treatment response and pneumotoxicity from programmed cell death protein or ligand-1 inhibition immunotherapy in NSCLC.J Thorac Oncol. 2023; 18: 718-730Abstract Full Text Full Text PDF Scopus (1) Google Scholar utilized CD274 (also referred to as PD-L1) as a response vector to develop a novel predictive model, which they term lung cancer immunotherapy –radiomics prediction vector (LCI-RPV).1Chen M. Lu H. Copley S.J. et al.A novel radiogenomics biomarker for predicting treatment response and pneumotoxicity from programmed cell death protein or ligand-1 inhibition immunotherapy in NSCLC.J Thorac Oncol. 2023; 18: 718-730Abstract Full Text Full Text PDF Scopus (1) Google Scholar The discovery cohort consisted of 85 patients from five public domain data sets and was therefore acquired on a range of scanners in the United States. Two independent cohorts (n = 109) were used for validation of their radiomics-based model. Ventana PD-L1 (SP263) rabbit monoclonal primary antibody or Dako PD-L1 (22C3) PharmDx assays (Agilent, Santa Clara, CA) were used to determine levels of PD-L1 expression by immunohistochemistry. The investigators found that LCI-RPV has predictive value for PD-L1 positivity in both testing cohorts (area under the curve [AUC] = 0.70). They further tested this model’s ability to predict cases of high PD-L1 expression and revealed an AUC of 0.72 for PD-L1 greater than 50% and an AUC of 0.66 for PD-L1 greater than 90% in one of the validation cohorts (ICHNT, n = 66). In addition, LCI-RPV exhibited a reasonable predictive value for objective response to treatment at 3 months with an AUC of 0.68 in the same cohort. Less so, the model was able to predict the occurrence of pneumonitis in those undergoing checkpoint inhibitor therapy with an AUC of 0.64. It is important to note that the lower 95% confidence interval crosses an AUC of 0.50 for the following three predictive models: (1) PD-L1 positivity in one of the two testing cohorts; (2) PD-L1 expression greater than 90%; and (3) risk of developing pneumonitis. Several other studies describe the use of radiomic features for estimating PD-L1 expression but with varying imaging techniques.8Wen Q. Yang Z. Dai H. Feng A. Li Q. Radiomics study for predicting the expression of PD-L1 and tumor mutation burden in non-small cell lung cancer based on CT images and clinicopathological features.Front Oncol. 2021; 11620246Crossref Scopus (20) Google Scholar, 9Mu W. Jiang L. Shi Y. et al.Non-invasive measurement of PD-L1 status and prediction of immunotherapy response using deep learning of PET/CT images.J Immunother Cancer. 2021; 9e002118Crossref Scopus (48) Google Scholar, 10Li J. Ge S. Sang S. Hu C. Deng S. Evaluation of PD-L1 expression level in patients with non-small cell lung cancer by 18F-FDG PET/CT radiomics and clinicopathological characteristics.Front Oncol. 2021; 11789014Crossref Scopus (16) Google Scholar Aside from variations in image acquisition, however, the investigators stray from previous research in this field by performing gene set enrichment analysis and single-cell RNA sequencing to correlate their predictive radiomic features with that of genomic expression profiles involved in antitumor immunity. This was an attempt to understand the biological correlates in the tumor microenvironment that are reflected in radiomic features investigated by their model—a technique infrequently explored for lung cancer and other similarly conducted studies. The relationships derived among LCI-RPV phenotype, myeloid cell localization and abundance, and hypoxia-associated gene pathways complement findings from other investigations,11Tunali I. Tan Y. Gray J.E. et al.Hypoxia-related radiomics and immunotherapy response: a multicohort study of non-small cell lung cancer.JNCI Cancer Spectr. 2021; 5: pkab048Crossref PubMed Scopus (14) Google Scholar,12Strauss L. Mahmoud M.A.A. Weaver J.D. et al.Targeted deletion of PD-1 in myeloid cells induces anti-tumor immunity.Sci Immunol. 2020; 5eaay1863Crossref PubMed Scopus (200) Google Scholar therefore, making a case for further exploration within this area of research. Given that the complex interactions between immune checkpoint inhibitors and the tumor immune microenvironment are poorly understood, advancing our knowledge in this area is bound to offer insight into the mechanisms of checkpoint inhibitor resistance within future studies. Of note, there were several limitations with this study including its retrospective nature, relatively small sample size, the unequal performance of the model across different thresholds of PD-L1 expression, and possible inconsistencies because of a training data set obtained from multiple sources. In clinical practice, the level of PD-L1 expression has major implications when developing a patient’s individualized treatment plan. In addition, we find that LCI-RPV can predict the risk of pneumonitis but do not fully understand the model’s ability to do so or how this should be incorporated into clinical practice. Such predictions, albeit interesting, should not deter oncologists from initiating immunotherapy but instead increase one’s suspicion for the development of pneumonitis and, thus, prevent delays in necessary intervention. Nevertheless, the authors confront what is considered a major limitation of radiomic studies: the generalizability of their model. Given the differences in the rates and volumes of contrast injection per case and the various types of CT scanners used to acquire imaging data in the training cohort, the investigators improve the reproducibility of LCI-RPV. Incorporating and advancing such methods within future studies is likely to result in more widely available models for consumer use; currently an unmet need within the field of radiomics. The crux behind recent advancements in radiomics has been its use in predicting responses to therapy. Whereas the LCI-RPV was predictive of responses to immunotherapy at 3 months, it remains to be seen whether it might be predictive of longer-term responses. Previously described predictive and prognostic models have incorporated multimodal data obtained from interrogating different components of the tumor microenvironment such as tumor-infiltrating lymphocyte density13Khorrami M. Prasanna P. Gupta A. et al.Changes in CT radiomic features associated with lymphocyte distribution predict overall survival and response to immunotherapy in non–small cell lung cancer.Cancer Immunol Res. 2020; 8: 108-119Crossref PubMed Scopus (122) Google Scholar and quantitative vessel tortuosity.14Alilou M. Khorrami M. Prasanna P. et al.A tumor vasculature–based imaging biomarker for predicting response and survival in patients with lung cancer treated with checkpoint inhibitors.Sci Adv. 2022; 8eabq4609Crossref PubMed Scopus (1) Google Scholar Integration of various layers of biological data into future radiomic models is likely to not only improve the accuracy of prediction but also provide a biological context to informative radiomic features. Akin to any other radiomic study, validation of LCI-RPV in larger independent data sets or a prospective manner is essential to circumvent the risk of overfitting and better understand its use in clinical practice. Embedding similar novel tools into prospective trials remains a challenge but is crucial for comprehending how they will perform when guiding medical decisions. Through proper validation, LCI-RPV and similar radiomic models have the potential to help guide informed decisions between oncologists and their patients; a necessary step toward bridging the gap between immunotherapy and precision medicine in lung cancer. Lukas Delasos: Conceptualization, Writing original draft of the manuscript, Editing. Anant Madabhushi: Conceptualization, Writing- Review and Editing. Pradnya D. Patil: Conceptualization, Writing original draft of the manuscript, Review and Editing. A Novel Radiogenomics Biomarker for Predicting Treatment Response and Pneumotoxicity From Programmed Cell Death Protein or Ligand-1 Inhibition Immunotherapy in NSCLCJournal of Thoracic OncologyVol. 18Issue 6PreviewPatient selection for checkpoint inhibitor immunotherapy is currently guided by programmed death-ligand 1 (PD-L1) expression obtained from immunohistochemical staining of tumor tissue samples. This approach is susceptible to limitations resulting from the dynamic and heterogeneous nature of cancer cells and the invasiveness of the tissue sampling procedure. To address these challenges, we developed a novel computed tomography (CT) radiomic-based signature for predicting disease response in patients with NSCLC undergoing programmed cell death protein 1 (PD-1) or PD-L1 checkpoint inhibitor immunotherapy. Full-Text PDF Open Access
更多查看译文
AI 理解论文
溯源树
样例
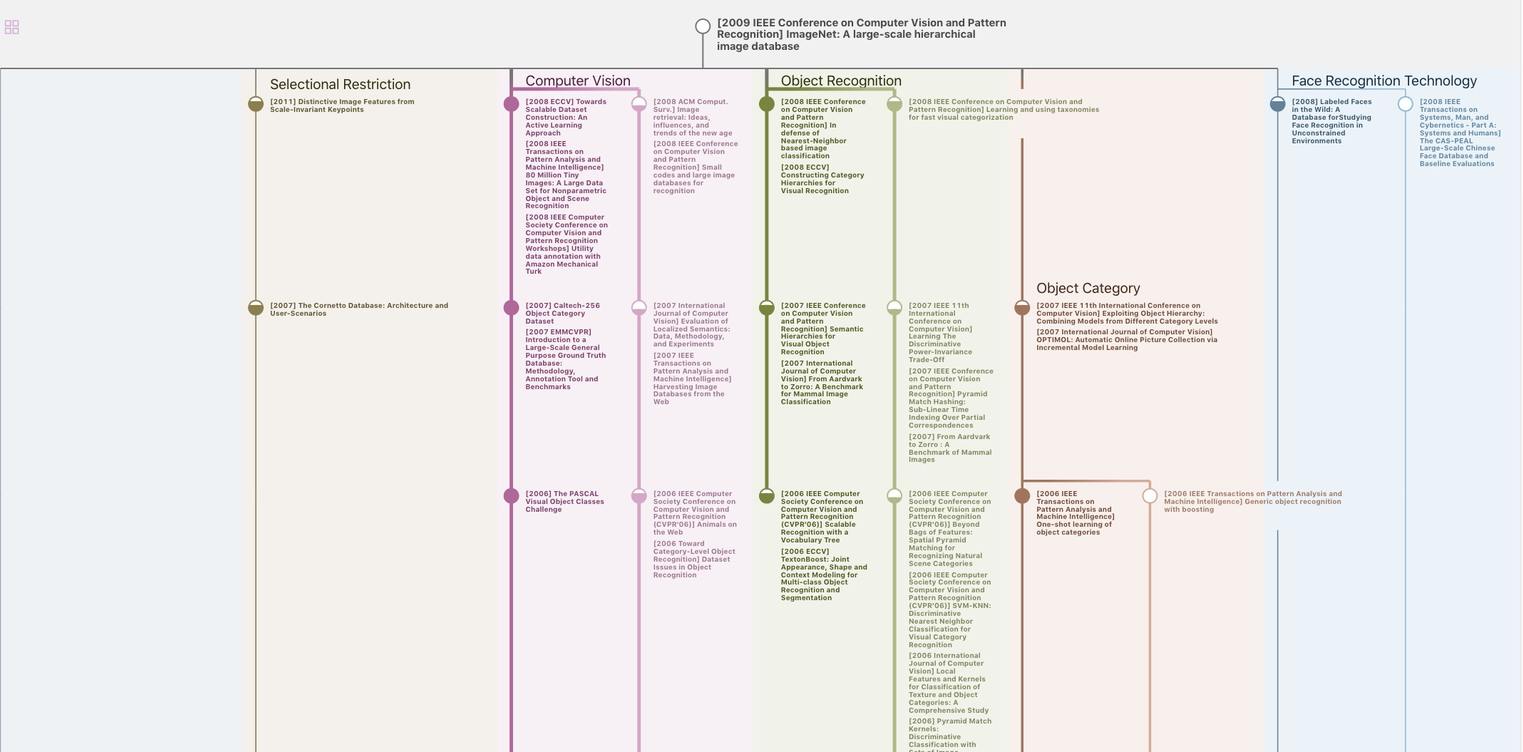
生成溯源树,研究论文发展脉络
Chat Paper
正在生成论文摘要