SC-AIR-BERT: a pre-trained single-cell model for predicting the antigen-binding specificity of the adaptive immune receptor.
Briefings in bioinformatics(2023)
摘要
Accurately predicting the antigen-binding specificity of adaptive immune receptors (AIRs), such as T-cell receptors (TCRs) and B-cell receptors (BCRs), is essential for discovering new immune therapies. However, the diversity of AIR chain sequences limits the accuracy of current prediction methods. This study introduces SC-AIR-BERT, a pre-trained model that learns comprehensive sequence representations of paired AIR chains to improve binding specificity prediction. SC-AIR-BERT first learns the 'language' of AIR sequences through self-supervised pre-training on a large cohort of paired AIR chains from multiple single-cell resources. The model is then fine-tuned with a multilayer perceptron head for binding specificity prediction, employing the K-mer strategy to enhance sequence representation learning. Extensive experiments demonstrate the superior AUC performance of SC-AIR-BERT compared with current methods for TCR- and BCR-binding specificity prediction.
更多查看译文
关键词
T-cell receptors, B-cell receptors, antigen-binding specificity, deep learning, BERT, GPT
AI 理解论文
溯源树
样例
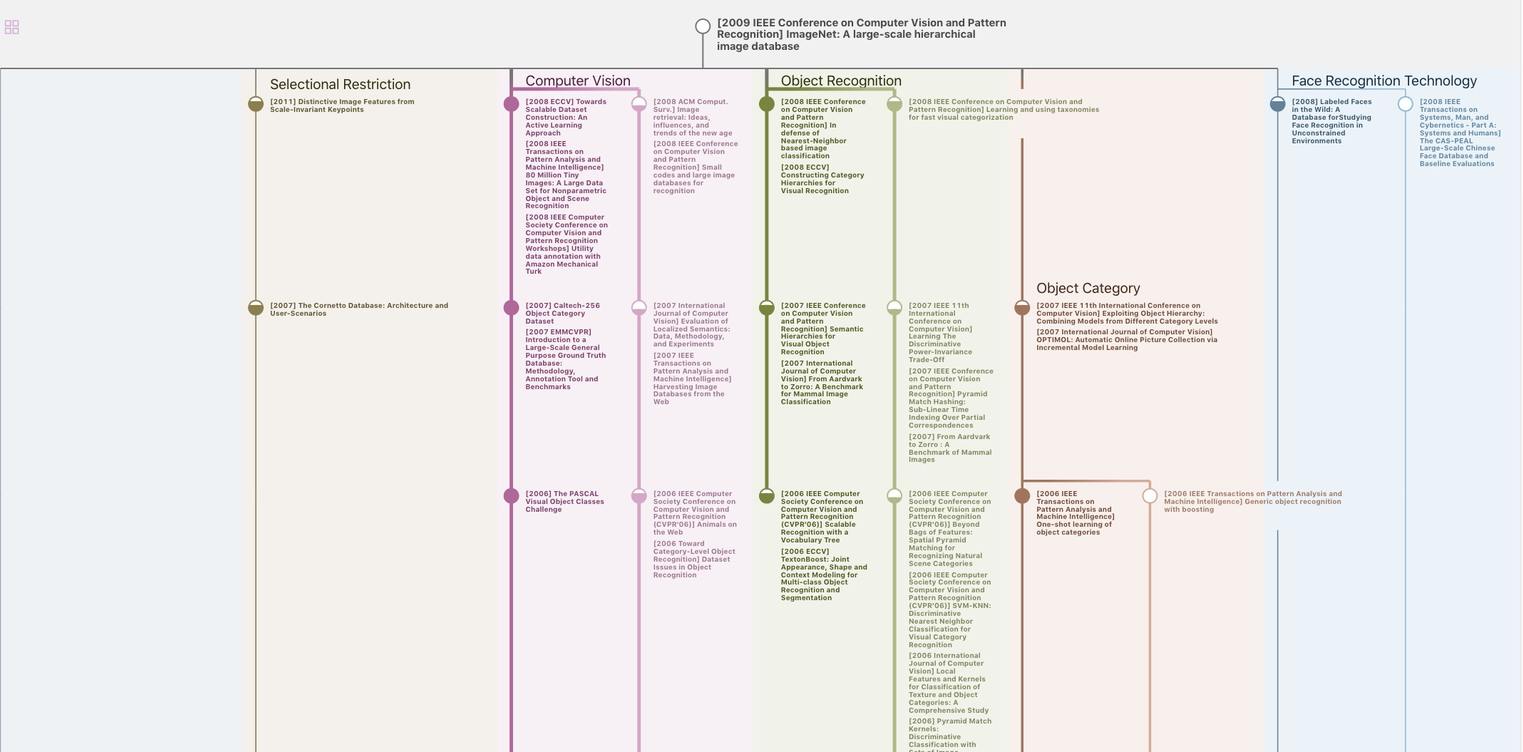
生成溯源树,研究论文发展脉络
Chat Paper
正在生成论文摘要