A Perceptual Measure for Deep Single Image Camera and Lens Calibration.
IEEE transactions on pattern analysis and machine intelligence(2023)
摘要
Image editing and compositing have become ubiquitous in entertainment, from digital art to AR and VR experiences. To produce beautiful composites, the camera needs to be geometrically calibrated, which can be tedious and requires a physical calibration target. In place of the traditional multi-image calibration process, we propose to infer the camera calibration parameters such as pitch, roll, field of view, and lens distortion directly from a single image using a deep convolutional neural network. We train this network using automatically generated samples from a large-scale panorama dataset, yielding competitive accuracy in terms of standard l error. However, we argue that minimizing such standard error metrics might not be optimal for many applications. In this work, we investigate human sensitivity to inaccuracies in geometric camera calibration. To this end, we conduct a large-scale human perception study where we ask participants to judge the realism of 3D objects composited with correct and biased camera calibration parameters. Based on this study, we develop a new perceptual measure for camera calibration and demonstrate that our deep calibration network outperforms previous single-image based calibration methods both on standard metrics as well as on this novel perceptual measure. Finally, we demonstrate the use of our calibration network for several applications, including virtual object insertion, image retrieval, and compositing.
更多查看译文
关键词
Single image camera calibration,human perception,horizon estimation,lens distortion
AI 理解论文
溯源树
样例
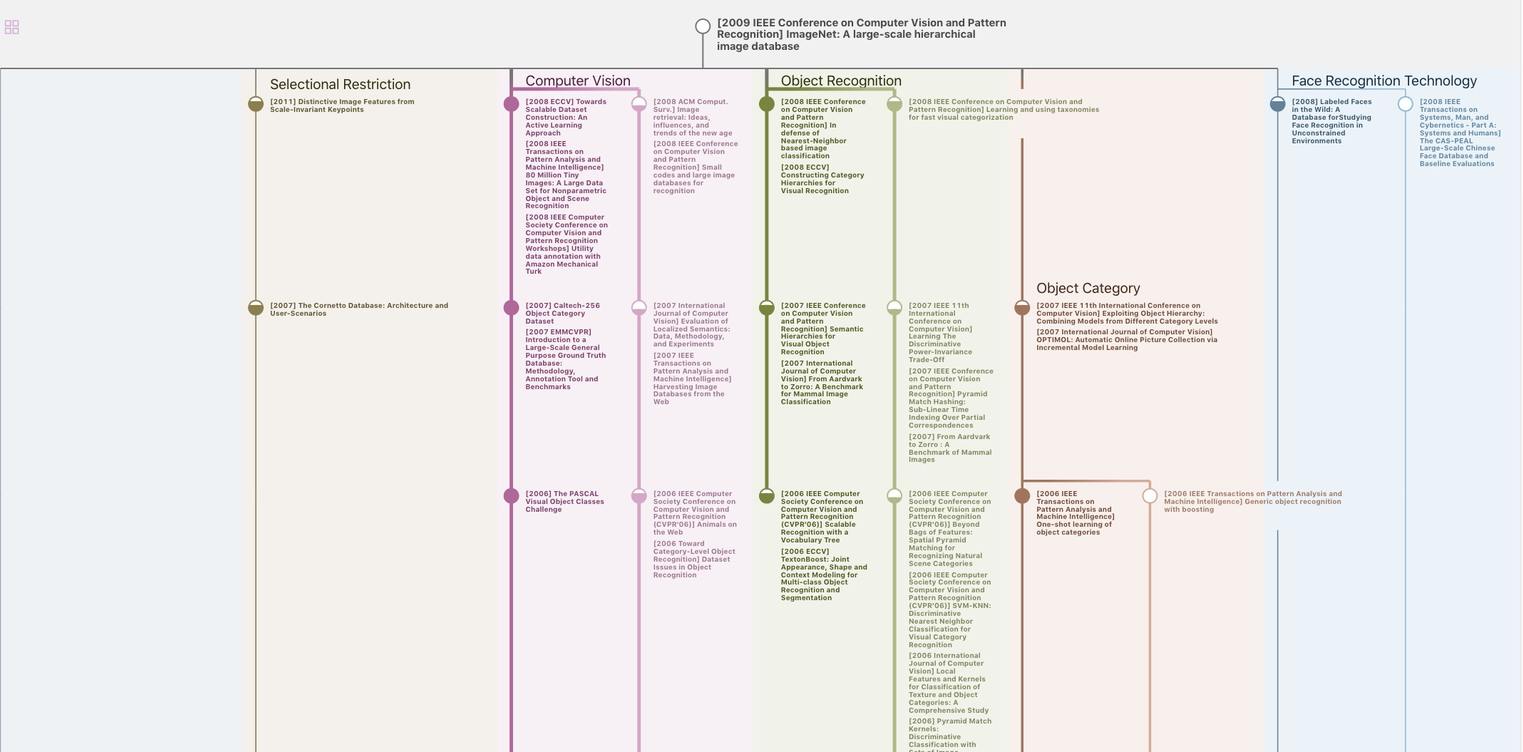
生成溯源树,研究论文发展脉络
Chat Paper
正在生成论文摘要