Machine Learning Predictions of Simulated Self-Diffusion Coefficients for Bulk and Confined Pure Liquids
Journal of chemical theory and computation(2023)
摘要
Diffusionproperties of bulk fluids have been predicted using empiricalexpressions and machine learning (ML) models, suggesting that predictionsof diffusion also should be possible for fluids in confined environments.The ability to quickly and accurately predict diffusion in porousmaterials would enable new discoveries and spur development in relevanttechnologies such as separations, catalysis, batteries, and subsurfaceapplications. In this work, we apply artificial neural network (ANN)models to predict the simulated self-diffusion coefficients of realliquids in both bulk and pore environments. The training data setswere generated from molecular dynamics (MD) simulations of Lennard-Jonesparticles representing a diverse set of 14 molecules ranging fromammonia to dodecane over a range of liquid pressures and temperatures.Planar, cylindrical, and hexagonal pore models consisted of wallscomposed of carbon atoms. Our simple model for these liquids was primarilyused to generate ANN training data, but the simulated self-diffusioncoefficients of bulk liquids show excellent agreement with experimentaldiffusion coefficients. ANN models based on simple descriptors accuratelyreproduced the MD diffusion data for both bulk and confined liquids,including the trend of increased mobility in large pores relativeto the corresponding bulk liquid.
更多查看译文
关键词
machine learning,self-diffusion
AI 理解论文
溯源树
样例
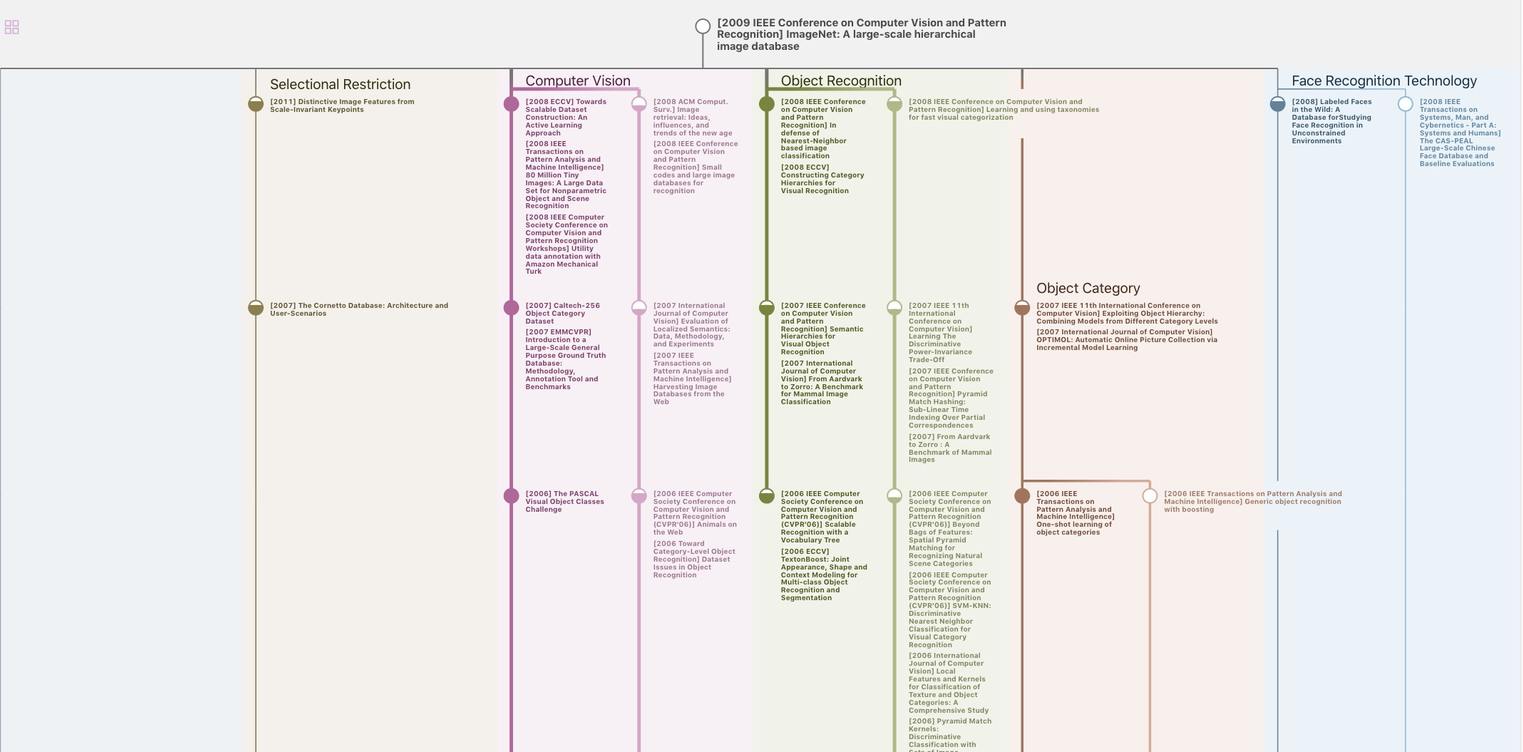
生成溯源树,研究论文发展脉络
Chat Paper
正在生成论文摘要