Long-Term Air Pollution Exposure and Mitochondrial DNA Copy Number: An Analysis of UK Biobank Data.
Environmental health perspectives(2023)
摘要
Vol. 131, No. 5 Research LetterOpen AccessLong-Term Air Pollution Exposure and Mitochondrial DNA Copy Number: An Analysis of UK Biobank Data Yun Soo Hong, Stephanie L. Battle, Daniela Puiu, Wen Shi, Nathan Pankratz, Di Zhao, Dan E. Arking, and Eliseo Guallar Yun Soo Hong Department of Epidemiology, Johns Hopkins Bloomberg School of Public Health, Baltimore, Maryland, USA Welch Center for Prevention, Epidemiology, and Clinical Research, Johns Hopkins Bloomberg School of Public Health, Baltimore, Maryland, USA McKusick-Nathans Institute, Department of Genetic Medicine, Johns Hopkins Medicine, Baltimore, Maryland, USA Search for more papers by this author , Stephanie L. Battle McKusick-Nathans Institute, Department of Genetic Medicine, Johns Hopkins Medicine, Baltimore, Maryland, USA Search for more papers by this author , Daniela Puiu Department of Biomedical Engineering, Johns Hopkins University, Baltimore, Maryland, USA Search for more papers by this author , Wen Shi McKusick-Nathans Institute, Department of Genetic Medicine, Johns Hopkins Medicine, Baltimore, Maryland, USA Search for more papers by this author , Nathan Pankratz Department of Laboratory Medicine and Pathology, University of Minnesota Medical School, Minneapolis, Minnesota, USA Search for more papers by this author , Di Zhao Department of Epidemiology, Johns Hopkins Bloomberg School of Public Health, Baltimore, Maryland, USA Welch Center for Prevention, Epidemiology, and Clinical Research, Johns Hopkins Bloomberg School of Public Health, Baltimore, Maryland, USA Search for more papers by this author , Dan E. Arking Address correspondence to Eliseo Guallar, Welch Center for Prevention, Epidemiology and Clinical Research, Johns Hopkins University Bloomberg School of Public Health, 2024 E. Monument St., Room 2-645, Baltimore, MD 21205 USA. Telephone: (410) 614-0574. Email: E-mail Address: [email protected]. And, Dan E. Arking, Johns Hopkins University School of Medicine, 733 N. Broadway, Miller Research Bldg, Room 459, Baltimore, MD 21205 USA. Telephone: (410) 502-4867. Email: E-mail Address: [email protected] McKusick-Nathans Institute, Department of Genetic Medicine, Johns Hopkins Medicine, Baltimore, Maryland, USA Search for more papers by this author , and Eliseo Guallar Address correspondence to Eliseo Guallar, Welch Center for Prevention, Epidemiology and Clinical Research, Johns Hopkins University Bloomberg School of Public Health, 2024 E. Monument St., Room 2-645, Baltimore, MD 21205 USA. Telephone: (410) 614-0574. Email: E-mail Address: [email protected]. And, Dan E. Arking, Johns Hopkins University School of Medicine, 733 N. Broadway, Miller Research Bldg, Room 459, Baltimore, MD 21205 USA. Telephone: (410) 502-4867. Email: E-mail Address: [email protected] https://orcid.org/0000-0002-4471-9565 Department of Epidemiology, Johns Hopkins Bloomberg School of Public Health, Baltimore, Maryland, USA Welch Center for Prevention, Epidemiology, and Clinical Research, Johns Hopkins Bloomberg School of Public Health, Baltimore, Maryland, USA Search for more papers by this author Published:16 May 2023CID: 057703https://doi.org/10.1289/EHP11946AboutSectionsPDF ToolsDownload CitationsTrack Citations ShareShare onFacebookTwitterLinked InReddit IntroductionThe adverse health effects of air pollution are believed to be mediated in part through oxidative stress.1 Mitochondrial DNA (mtDNA) is susceptible to oxidative stress and damaged mtDNA produces excessive reactive oxygen species (ROS), further aggravating mtDNA damage. mtDNA copy number (mtDNA-CN) is a marker of mitochondrial dysfunction that can be measured in peripheral blood, and low mtDNA-CN has been associated with adverse health effects.2Although air pollution is a pervasive source of oxidative stress, the association between long-term exposure to air pollution and mtDNA-CN has been inconclusive,3–5 limited by small sample sizes,4,5 wide variations in exposure concentration and composition, and heterogeneity in exposure and mtDNA-CN measurement. We therefore evaluated the association between long-term exposure to air pollution [particulate matter greater than 10>10 (particulate matter begin subscript 10 end subscriptPM10) and less than or equal to 2.5 micrograms≤2.5μg in aerodynamic diameter (particulate matter begin subscript 2.5 end subscriptPM2.5), black carbon, and nitrogen dioxide (nitrogen dioxideNO2)] with mtDNA-CN in over 45,000 adults from the UK Biobank study.MethodsWe used data on 45,665 participants from the UK Biobank who were enrolled in 2010 with available whole genome sequencing (WGS) data.6 Study participants provided demographic information, medical history, blood samples, and underwent a physical examination at enrollment.Air pollutant concentrations were modeled using a land-use regression model developed for the European Study of Cohorts for Air Pollution Effects (ESCAPE) project.7,8 The leave-one-out cross-validation uppercase italic r squaredR2 for particulate matter begin subscript 10 end subscriptPM10, particulate matter begin subscript 2.5 end subscriptPM2.5, black carbon, and nitrogen dioxideNO2 in the UK were 0.75–0.88, 0.21–0.77, 0.81–0.92, and 0.75–0.87, respectively. The annual average concentrations of air pollutants in 2010 were estimated for each participant’s residential address.To estimate mtDNA-CN, we ran the MitoHPC pipeline9 on WGS data, given that WGS performs substantially better than quantitative real-time polymerase chain reaction or whole exome sequencing techniques for mtDNA-CN. mtDNA-CN was calculated as mitochondrial coverage relative to nuclear genome coverage, log-transformed, and standardized so that the mtDNA-CN metric represents standard deviation (SD) units (z-scores).We used linear regression models to estimate the association between a 10 microgram per meter cubed10-μg/m3 increase in air pollutant exposure (1 microgram per meter cubed1 μg/m3 for black carbon) and mtDNA-CN. Standard errors were estimated using sandwich covariance matrix estimation (sandwich package in R) to account for the dependence among genetically related participants. In addition, we compared the differences in mtDNA-CN by quintiles of air pollutant concentrations using the lowest quintile as the reference. We evaluated linear trends across quintiles of exposure by including the quintile indicator as a continuous variable. We further modeled the dose–response relationship using restricted cubic splines (3 knots at the 10th, 50th, and 90th percentiles of the distribution of each air pollutant). All analyses were performed using R (version 4.1.3; R Development Core Team).All participants provided written informed consent. The protocol and procedures of the UK Biobank study were approved by the UK North West–Haydock Research Ethics Committee. Ethical approval was provided by the institutional review board of the Johns Hopkins School of Medicine.ResultsThe mean age plus or minus the standard deviation±SD of the 45,665 study participants (20,771 men and 24,894 women) was 56.8 plus or minus 8.156.8±8.1 years. The median (interquartile range) annual average concentrations of particulate matter begin subscript 10 end subscriptPM10, particulate matter begin subscript 2.5 end subscriptPM2.5, black carbon, and nitrogen dioxideNO2 were 16.2 (15.5–17.1), 9.9 (9.3–10.4), 1.2 (1.0–1.4), and 27.8 open parenthesis 22.7 to 32.4 close parenthesis micrograms per meter cubed27.8(22.7–32.4) μg/m3, respectively. mtDNA-CN ranged from negative 4.57−4.57 to 6.92. Compared with participants in the lowest quintile of mtDNA-CN, those in the highest quintile were younger (mean age equals 57.9 plus or minus 8.1=57.9±8.1 vs. 55.6 plus or minus 8.155.6±8.1), more likely to be never/former smokers (89.1% vs. 90.8%), and less likely to have prevalent hypertension, diabetes, hyperlipidemia, or cardiovascular disease.In the fully adjusted model (Table 1), a 10 micrograms per meter cubed10-μg/m3 increase in particulate matter begin subscript 10 end subscriptPM10, particulate matter begin subscript 2.5 end subscriptPM2.5, and nitrogen dioxideNO2 was associated with a difference in mtDNA-CN of negative 0.058−0.058 units [95% confidence interval (CI): negative 0.108−0.108 units, negative 0.007−0.007], negative 0.043−0.043 units (negative 0.146−0.146, 0.060), and negative 0.008−0.008 units (negative 0.021−0.021, 0.005), respectively. For black carbon, the corresponding difference in mtDNA-CN associated with a 1 microgram per meter cubed1-μg/m3 increase in exposure was negative 0.036−0.036 units (negative 0.068−0.068, negative 0.004−0.004). There was a linear inverse association of particulate matter begin subscript 10 end subscriptPM10 with mtDNA-CN and an inverse association with mtDNA-CN at higher concentrations of black carbon and nitrogen dioxideNO2 (Figure 1).Table 1 Average difference in mtDNA-CN (95% CI) associated with a 10 microgram per meter cubed10-μg/m3 increase in each air pollutant and by quintile of air pollutant in 2010, among 45,665 participants from the UK Biobank.Linear (per 10 microgram per meter cubed10-μg/m3 increase)Quintilelowercase italic p for trendpTrend12345particulate matter begin subscript 10 end subscriptPM10—11.78, 15.22(lowercase italic n equals 9,098n=9,098)15.23, 15.94(lowercase italic n equals 8,978n=8,978)15.95, 16.52(lowercase italic n equals 8,982n=8,982)16.53, 17.41(lowercase italic n equals 9,006n=9,006)17.42, 26.16(lowercase italic n equals 8,982n=8,982)— Model 1negative 0.083−0.083(negative 0.134−0.134, negative 0.031−0.031)Refnegative 0.022−0.022(negative 0.051−0.051, 0.007)negative 0.013−0.013(negative 0.041−0.041, 0.016)negative 0.055−0.055(negative 0.084−0.084, negative 0.027−0.027)negative 0.031−0.031(negative 0.060−0.060, negative 0.002−0.002)0.004 Model 2negative 0.084−0.084(negative 0.139−0.139, negative 0.029−0.029)Refnegative 0.006−0.006(negative 0.037−0.037, 0.025)negative 0.007−0.007(negative 0.038−0.038, 0.024)negative 0.057−0.057(negative 0.088−0.088, negative 0.026−0.026)negative 0.033−0.033(negative 0.064−0.064, negative 0.002−0.002)0.001 Model 3negative 0.058−0.058(negative 0.108−0.108, negative 0.007−0.007)Ref0.004(negative 0.025−0.025, 0.032)0.010(negative 0.028−0.028, 0.038)negative 0.029−0.029(negative 0.057−0.057, negative 0.000−0.000)negative 0.019−0.019(negative 0.048−0.048, 0.009)0.03particulate matter begin subscript 2.5 end subscriptPM2.5—8.17, 9.16(lowercase italic n equals 9,129n=9,129)9.17, 9.65(lowercase italic n equals 9,029n=9,029)9.66, 10.04(lowercase italic n equals 8,973n=8,973)10.05, 10.54(lowercase italic n equals 8,937n=8,937)10.55, 18.41(lowercase italic n equals 8,978n=8,978)— Model 1negative 0.079−0.079(negative 0.181−0.181, 0.024)Refnegative 0.029−0.029(negative 0.057−0.057, negative 0.000−0.000)0.006(negative 0.022−0.022, 0.035)negative 0.014−0.014(negative 0.043−0.043, 0.015)negative 0.031−0.031(negative 0.060−0.060, negative 0.02−0.02)0.16 Model 2negative 0.057−0.057(negative 0.168−0.168, 0.055)Refnegative 0.022−0.022(negative 0.052−0.052, 0.009)0.015(negative 0.016−0.016, 0.046)negative 0.001−0.001(negative 0.032−0.032, 0.030)negative 0.026−0.026(negative 0.058−0.058, 0.005)0.39 Model 3negative 0.043−0.043(negative 0.146−0.146, 0.060)Refnegative 0.005−0.005(negative 0.034−0.034, 0.023)0.021(negative 0.007−0.007, 0.049)0.011(negative 0.017−0.017, 0.040)negative 0.017−0.017(negative 0.046−0.046, 0.012)0.64Black carbona—0.83, 1.01(lowercase italic n equals 9,358n=9,358)1.02, 1.14(lowercase italic n equals 9,031n=9,031)1.15, 1.28(lowercase italic n equals 8,735n=8,735)1.29, 1.44(lowercase italic n equals 8,977n=8,977)1.45, 3.71(lowercase italic n equals 8,945n=8,945)— Model 1negative 0.038−0.038(negative 0.071−0.071, negative 0.006−0.006)Refnegative 0.037−0.037(negative 0.066−0.066, negative 0.009−0.009)0.005(negative 0.024−0.024, 0.034)negative 0.025−0.025(negative 0.054−0.054, 0.004)negative 0.039−0.039(negative 0.068−0.068, negative 0.009−0.009)0.05 Model 2negative 0.051−0.051(negative 0.833−0.833, negative 0.185−0.185)Refnegative 0.038−0.038(negative 0.069−0.069, negative 0.008−0.008)0.008(negative 0.023−0.023, 0.040)negative 0.034−0.034(negative 0.065−0.065, negative 0.002−0.002)negative 0.047−0.047(negative 0.079−0.079, negative 0.016−0.016)0.01 Model 3negative 0.036−0.036(negative 0.068−0.068, negative 0.004−0.004)Refnegative 0.004−0.004(negative 0.033−0.033, 0.024)0.035(0.007, 0.064)negative 0.010−0.010(negative 0.039−0.039, 0.018)negative 0.027−0.027(negative 0.056−0.056, negative 0.002−0.002)0.08nitrogen dioxideNO2—12.93, 21.61(lowercase italic n equals 9,023n=9,023)21.62, 25.66(lowercase italic n equals 9,029n=9,029)25.67, 29.72(lowercase italic n equals 8,992n=8,992)29.73, 33.38(lowercase italic n equals 9,027n=9,027)33.39, 97.74(lowercase italic n equals 8,995n=8,995)— Model 1negative 0.012−0.012(negative 0.028−0.028, 0.001)Refnegative 0.007−0.007(negative 0.036−0.036, 0.022)negative 0.012−0.012(negative 0.041−0.041, 0.017)negative 0.002−0.002(negative 0.031−0.031, 0.027)negative 0.028−0.028(negative 0.057−0.057, 0.002)0.14 Model 2negative 0.012−0.012(negative 0.025−0.025, 0.002)Refnegative 0.001−0.001(negative 0.031−0.031, 0.030)negative 0.009−0.009(negative 0.040−0.040, 0.022)0.004(negative 0.027−0.027, 0.035)negative 0.028−0.028(negative 0.059−0.059, 0.004)0.18 Model 3negative 0.008−0.008(negative 0.021−0.021, 0.005)Ref0.017(negative 0.012−0.012, 0.045)0.021(negative 0.008−0.008, 0.049)0.025(negative 0.003−0.003, 0.054)negative 0.015−0.015(negative 0.045−0.045, 0.014)0.57Note: Model 1: Adjusted for age (continuous), sex (men and women), self-reported ethnic background (White, Black, Asian, and Other), and study center; Model 2: model 1 plus average annual incomeModel 1+average annual income (less than 18,000 pounds<£18,000, £18,000–£30,999, £31,000–£51,999, £52,000–£100,000, and greater than 100,000 pounds>£100,000), education level (less than college, college or university degree, professional degree, and other), smoking (never, former, and current), alcohol intake (never, former, and current), physical activity (walking, moderate, and vigorous physical activity), body mass index (continuous), and history of hypertension, diabetes, hyperlipidemia, and cardiovascular disease; Model 3: model 2 plus cell countsModel 2+cell counts (red blood cells, neutrophils, lymphocytes, basophils, eosinophils, monocytes, and platelets as continuous variables). Hypertension was defined as a self-reported physician’s diagnosis of hypertension, a self-reported use of antihypertensive medication, or a measured systolic blood pressure greater than or equal to 140 millimeters of mercury≥140 mmHg or diastolic blood pressure greater than or equal to 90 millimeters of mercury≥90 mmHg. Diabetes was defined as a self-reported physician’s diagnosis of diabetes, a self-reported use of antidiabetic medication, or a measured HbA1c greater than or equal to 6.5 percent≥6.5%. Hyperlipidemia was defined as a self-reported use of lipid-lowering medication, or a measured total cholesterol greater than or equal to 200 milligrams per deciliter≥200mg/dL or triglycerides greater than or equal to 150 milligrams per deciliter≥150mg/dL. Cardiovascular disease was defined as the presence of either myocardial infarction or stroke based on the algorithm developed by the UK Biobank. —, not applicable; CI, confidence interval; HbA1c, hemoglobin A1c; mtDNA-CN, mitochondrial DNA copy number; nitrogen dioxideNO2, nitrogen dioxide; particulate matter begin subscript 2.5 end subscriptPM2.5, particulate matter less than 2.5 micrograms≤2.5μg in aerodynamic diameter; particulate matter begin subscript 10 end subscriptPM10, particulate matter less than or equals to 10 micrograms≤10μg in aerodynamic diameter; Ref, reference.aThe linear estimates for black carbon are per 1 microgram per meter cubed1-μg/m3 increase.Figure 1. Average mtDNA-CN levels by 2010 residential particulate matter begin subscript 10 end subscriptPM10, particulate matter begin subscript 2.5 end subscriptPM2.5, black carbon, and nitrogen dioxideNO2 concentrations, in 45,665 participants from the UK Biobank. The curves represent estimated mtDNA-CN levels (solid line) and their 95% CIs (gray area) by particulate matter begin subscript 10 end subscriptPM10, particulate matter begin subscript 2.5 end subscriptPM2.5, black carbon, and nitrogen dioxideNO2 concentrations based on fully adjusted regression models using restricted cubic splines to model air pollutants with knots at the 10th, 50th, and 90th percentiles of its distribution. The spline regression model was adjusted for age (continuous), sex (men and women), self-reported ethnic background (White, Black, Asian, and Other), average annual income (less than 18,000 pounds<£18,000, £18,000–£30,999, £31,000–£51,999, £52,000–£100,000, and greater than 100,000 pounds>£100,000), education level (less than college, college or university degree, professional degree, and other), smoking (never, former, and current), alcohol intake (never, former, and current), physical activity (walking, moderate, and vigorous physical activity), body mass index (continuous), history of hypertension, diabetes, hyperlipidemia, and cardiovascular disease, and blood cell counts (red blood cells, neutrophils, lymphocytes, monocytes, eosinophils, basophils, and platelets as continuous variables). The histograms show the distribution of the concentrations of each air pollutant in the overall study population. Corresponding numeric data for the spline figure are available in our GitHub repository ( https://github.com/ArkingLab/Air-pollution-and-mtDNA_CN). Note: CI, confidence interval; mtDNA-CN, mitochondrial DNA copy number; nitrogen dioxideNO2, nitrogen dioxide; particulate matter begin subscript 2.5 end subscriptPM2.5, particulate matter less than or equal to 2.5 micrograms≤2.5μg in aerodynamic diameter; particulate matter begin subscript 10 end subscriptPM10, particulate matter less than or equals 10 micrograms≤10μg in aerodynamic diameter.DiscussionIn this large-scale population study, higher concentrations of air pollutants were inversely associated with mtDNA-CN. Exposure to air pollutants impact various mitochondrial functions, including oxidative phosphorylation, calcium regulation, and mitochondrial membrane potential, in both in vitro and in vivo studies.1 In animal studies, particulate matter also accelerated the production of ROS and disturbed the fission and fusion of mitochondria, resulting in mitochondrial dysfunction.1Long-term exposure to particulate matter begin subscript 2.5 end subscriptPM2.5 was inversely associated with mtDNA-CN in 2,758 healthy women from the Nurses’ Health Study.3 In addition, particulate matter begin subscript 2.5 end subscriptPM2.5 exposure in the past year was inversely associated with mtDNA-CN in two populations of older adults.4,5 In our study, nitrogen dioxideNO2 was also inversely associated with mtDNA-CN at higher concentrations. On the other hand, 3-y average exposure to particulate matter begin subscript 10 end subscriptPM10, particulate matter begin subscript 2.5 end subscriptPM2.5, and nitrogen dioxideNO2 measured in 10 kilometers squared10-km2 grids was positively associated with mtDNA-CN in a rural Chinese population (uppercase italic n equals 2,707N=2,707).10 The different results of this study compared with our study may be due to differences in the duration and concentration of exposure, in the methods of mtDNA-CN assessment, in the population characteristics, or to increased variability in small samples.The limitations of our study include the cross-sectional design, the measurement of mtDNA-CN on a single occasion, and the use of air pollution measurements derived from prediction models for the participants’ residential addresses, which may be subject to misclassification. Moreover, the UK Biobank comprises mostly White individuals, and the generalizability of the findings to other populations is unknown.In conclusion, long-term exposure to air pollutants was inversely associated with mtDNA-CN. These findings suggest that oxidative stress-induced mitochondrial dysfunction, reflected by reduced mtDNA-CN, may be a potential mechanism mediating the adverse health effects of air pollution.AcknowledgmentsThis work was supported by the U.S. National Institutes of Health grants R01HL131573 and R01HL144569 (to D.E.A.).This research was also conducted using the UK Biobank Resource under application no. 17731. All data used in this study are available through application to the UK Biobank. Additional information on registration for data access can be found at http://www.ukbiobank.ac.uk/register-apply/.References1. Daiber A, Kuntic M, Hahad O, Delogu LG, Rohrbach S, Di Lisa F, et al.2020. Effects of air pollution particles (ultrafine and fine particulate matter) on mitochondrial function and oxidative stress—implications for cardiovascular and neurodegenerative diseases. Arch Biochem Biophys 696:108662, PMID: 33159890, 10.1016/j.abb.2020.108662. Crossref, Medline, Google Scholar2. Castellani CA, Longchamps RJ, Sun J, Guallar E, Arking DE. 2020. Thinking outside the nucleus: mitochondrial DNA copy number in health and disease. Mitochondrion 53:214–223, PMID: 32544465, 10.1016/j.mito.2020.06.004. Crossref, Medline, Google Scholar3. Wang X, Hart JE, Liu Q, Wu S, Nan H, Laden F. 2020. Association of particulate matter air pollution with leukocyte mitochondrial DNA copy number. Environ Int 141:105761, PMID: 32388147, 10.1016/j.envint.2020.105761. Crossref, Medline, Google Scholar4. Pieters N, Janssen BG, Dewitte H, Cox B, Cuypers A, Lefebvre W, et al.2016. Biomolecular markers within the core axis of aging and particulate air pollution exposure in the elderly: a cross-sectional study. Environ Health Perspect 124(7):943–950, PMID: 26672058, 10.1289/ehp.1509728. Link, Google Scholar5. Peng C, Cayir A, Sanchez-Guerra M, Di Q, Wilson A, Zhong J, et al.2017. Associations of annual ambient fine particulate matter mass and components with mitochondrial DNA abundance. Epidemiology 28(6):763–770, PMID: 28953603, 10.1097/EDE.0000000000000717. Crossref, Medline, Google Scholar6. Sudlow C, Gallacher J, Allen N, Beral V, Burton P, Danesh J, et al.2015. UK Biobank: an open access resource for identifying the causes of a wide range of complex diseases of middle and old age. PLoS Med 12(3):e1001779, PMID: 25826379, 10.1371/journal.pmed.1001779. Crossref, Medline, Google Scholar7. Eeftens M, Beelen R, de Hoogh K, Bellander T, Cesaroni G, Cirach M, et al.2012. Development of land use regression models for PM2.5, PM2.5 absorbance, PM10 and PMcoarse in 20 European study areas; results of the ESCAPE project. Environ Sci Technol 46(20):11195–11205, PMID: 22963366, 10.1021/es301948k. Crossref, Medline, Google Scholar8. Beelen R, Hoek G, Vienneau D, Eeftens M, Dimakopoulou K, Pedeli X, et al.2013. Development of NO2 and NOx land use regression models for estimating air pollution exposure in 36 study areas in Europe—the ESCAPE project. Atmos Environ 72:10–23, 10.1016/j.atmosenv.2013.02.037. Crossref, Google Scholar9. Battle SL, Puiu D, TOPMed mtDNA Working Group, Boerwinkle E, Taylor KD, Rotter JI, et al.2021. A bioinformatics pipeline for estimating mitochondria DNA copy number and heteroplasmy levels from whole genome sequencing data. Medrxiv. Preprint posted online 30 December 2021. 10.1101/2021.12.28.21268452. Crossref, Google Scholar10. Li R, Li S, Pan M, Chen H, Liu X, Chen G, et al.2021. Physical activity counteracted associations of exposure to mixture of air pollutants with mitochondrial DNA copy number among rural Chinese adults. Chemosphere 272:129907, PMID: 33601207, 10.1016/j.chemosphere.2021.129907. Crossref, Medline, Google ScholarThe authors declare they have nothing to disclose.FiguresReferencesRelatedDetails Vol. 131, No. 5 May 2023Metrics About Article Metrics Publication History Manuscript received1 August 2022Manuscript revised10 April 2023Manuscript accepted12 April 2023Originally published16 May 2023 Financial disclosuresPDF download License information EHP is an open-access journal published with support from the National Institute of Environmental Health Sciences, National Institutes of Health. All content is public domain unless otherwise noted. Note to readers with disabilities EHP strives to ensure that all journal content is accessible to all readers. However, some figures and Supplemental Material published in EHP articles may not conform to 508 standards due to the complexity of the information being presented. If you need assistance accessing journal content, please contact [email protected]. Our staff will work with you to assess and meet your accessibility needs within 3 working days.
更多查看译文
关键词
mitochondrial dna copy number,uk biobank data,pollution,long-term
AI 理解论文
溯源树
样例
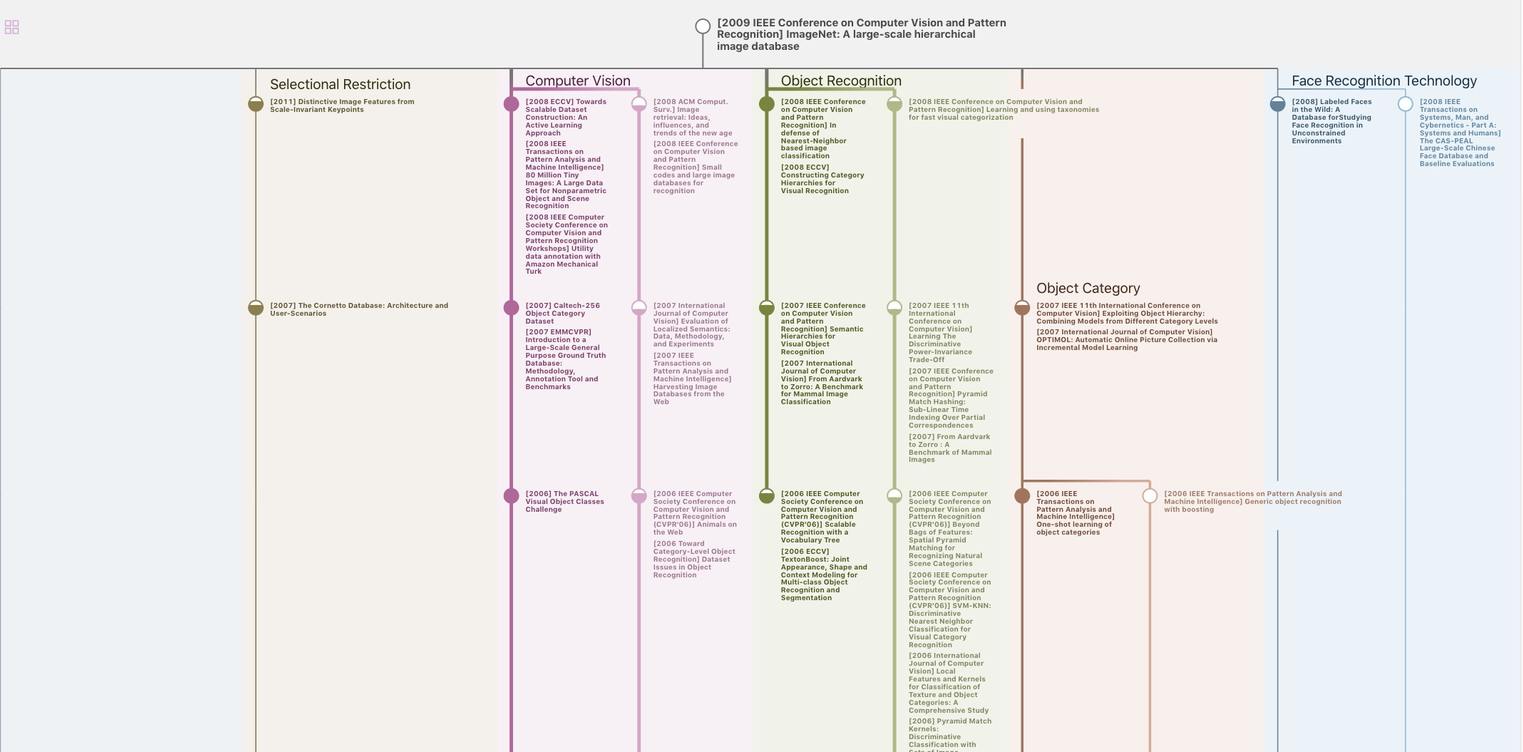
生成溯源树,研究论文发展脉络
Chat Paper
正在生成论文摘要