Molecular Generation with Reduced Labeling through Constraint Architecture.
Journal of chemical information and modeling(2023)
摘要
In the past few years, a number of machine learning (ML)-based molecular generative models have been proposed for generating molecules with desirable properties, but they all require a large amount of label data of pharmacological and physicochemical properties. However, experimental determination of these labels, especially bioactivity labels, is very expensive. In this study, we analyze the dependence of various multi-property molecule generation models on biological activity label data and propose Frag-G/M, a fragment-based multi-constraint molecular generation framework based on conditional transformer, recurrent neural networks (RNNs), and reinforcement learning (RL). The experimental results illustrate that, using the same number of labels, Frag-G/M can generate more desired molecules than the baselines (several times more than the baselines). Moreover, compared with the known active compounds, the molecules generated by Frag-G/M exhibit higher scaffold diversity than those generated by the baselines, thus making it more promising to be used in real-world drug discovery scenarios.
更多查看译文
关键词
molecular generation,reduced labeling
AI 理解论文
溯源树
样例
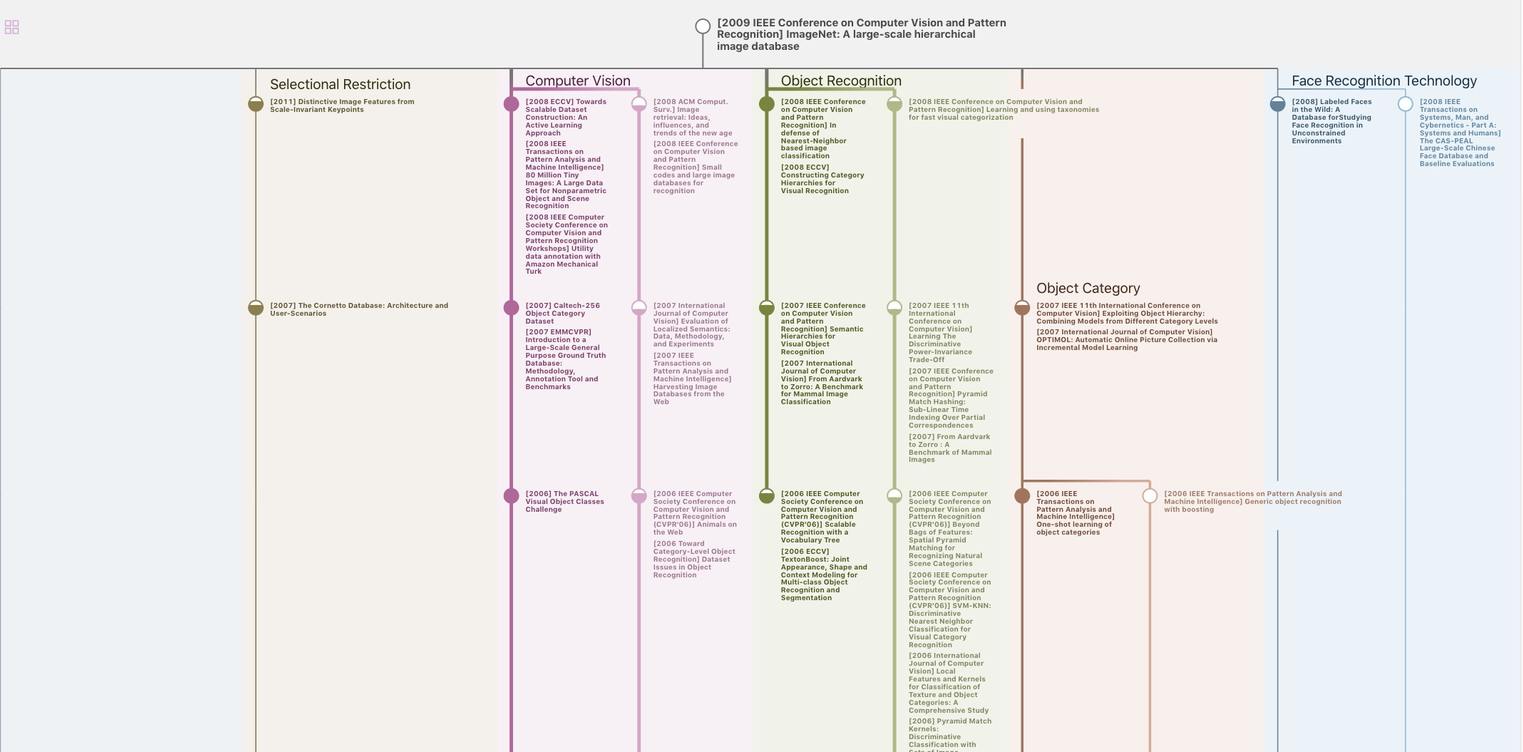
生成溯源树,研究论文发展脉络
Chat Paper
正在生成论文摘要