Semi-supervised deep embedded clustering with pairwise constraints and subset allocation.
Neural networks : the official journal of the International Neural Network Society(2023)
摘要
Semi-supervised deep clustering methods attract much attention due to their excellent performance on the end-to-end clustering task. However, it is hard to obtain satisfying clustering results since many overlapping samples in industrial text datasets strongly and incorrectly influence the learning process. Existing methods incorporate prior knowledge in the form of pairwise constraints or class labels, which not only largely ignore the correlation between these two supervision information but also cause the problem of weak-supervised constraint or incorrect strong-supervised label guidance. In order to tackle these problems, we propose a semi-supervised method based on pairwise constraints and subset allocation (PCSA-DEC). We redefine the similarity-based constraint loss by forcing the similarity of samples in the same class much higher than other samples and design a novel subset allocation loss to precisely learn strong-supervised information contained in labels which consistent with unlabeled data. Experimental results on the two industrial text datasets show that our method can yield 8.2%-8.7% improvement in accuracy and 13.4%-19.8% on normalized mutual information over the state-of-the-art method.
更多查看译文
关键词
clustering,pairwise constraints,semi-supervised
AI 理解论文
溯源树
样例
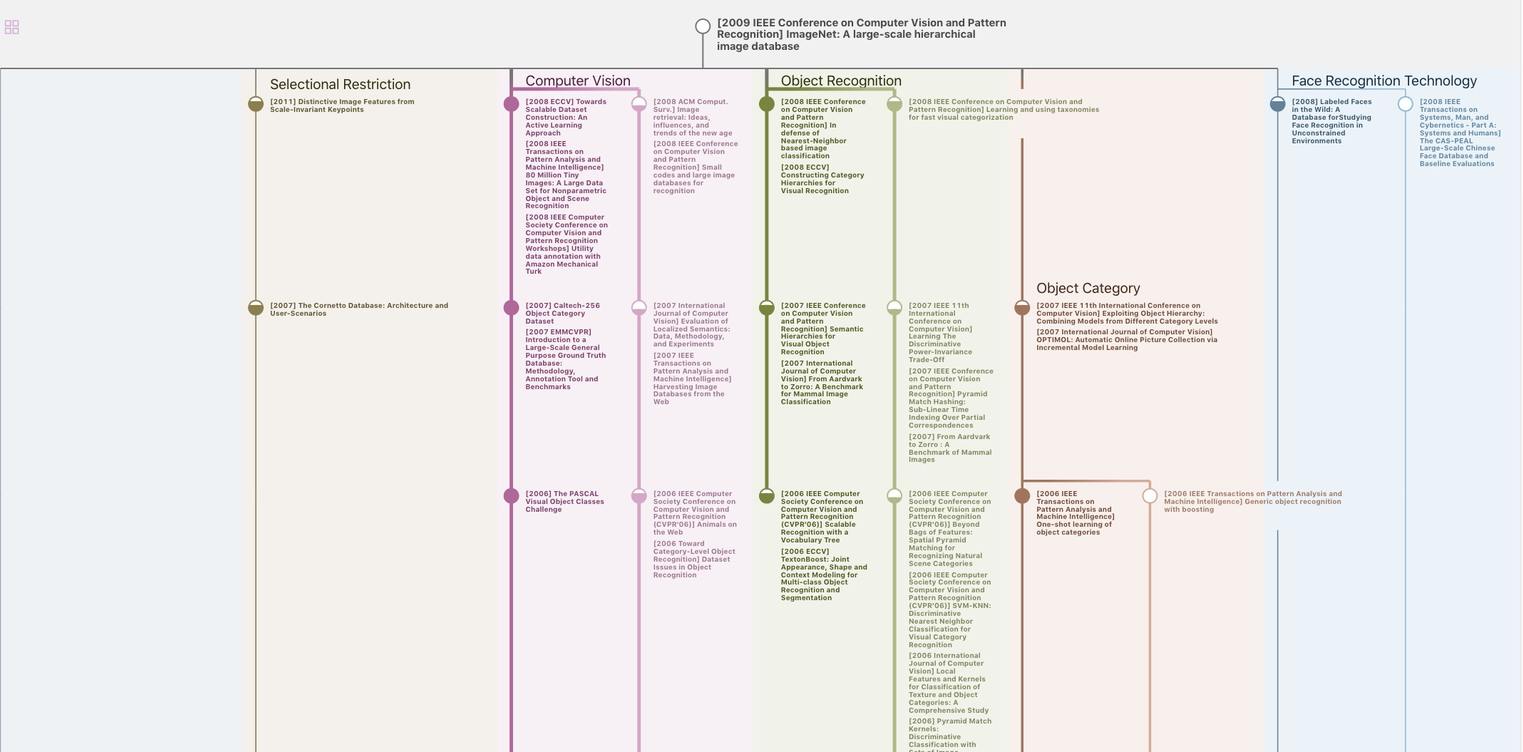
生成溯源树,研究论文发展脉络
Chat Paper
正在生成论文摘要