Benchmarking methods and data for the whole-outline geometric morphometric analysis of lithic tools
Evolutionary anthropology(2023)
摘要
Originally developed for the quantitative analysis of organismal shapes, both two-dimensional (2D) and 3D geometric morphometric methods (GMMs) have recently gained some prominence in archaeology for the analysis of stone tools1-3—unquestionably the primary deep-time data source for the earliest periods of human cultural evolution.4 The key strength of GMM rests in its ability to statistically quantify and hence qualify complex shapes, which in turn can be used to infer social interaction,5 function,6, 7 reduction,8 as well as to assess classification systems and cultural relatedness.9-11 The methodological diversification that has accompanied the rise in popularity of this particular suite of methods has, however, also resulted in an increasing lack of comparability and interoperability, which—ironically—works against the promise of GMM to provide a tool for comparing artifact shapes that is not sensitive to interanalyst variation. Standardized protocols, vetted datasets, as well as case-transferable and fully reproducible methods do not currently exist, hampering the full utility of geometric morphometrics as an approach to comparatively understand human behavior as reflected in these lithic proxies. Additionally, the emerging issue of methodological diversity in the geometric morphometric analysis of stone tools is further compounded by issues related to landmark selection. When applied to organisms, landmark selection is guided by a priori knowledge about ontogeny, homology, and function. For stone tools, however, only very few such evident landmarks suggest themselves.2 Instead, many studies have used landmarks selected specifically to highlight particular design features of a given tool class (e.g., stemmed points or leaf points). These cannot, however, be easily compared across tool classes. Other studies have used sets of equidistant landmarks measured perpendicularly from a given tool's longest axis to its margins to describe overall shape. In this context, whole-outline geometric morphometrics offers an alternative approach that circumvents landmark selection by describing the entire outline of the recorded artifact. It is computationally tractable, readily replicable, and well-suited for 2D object representations such as drawings and photographs, many of which exist in excavation reports, catalogs, finds registers and the published literature at large. Furthermore, emerging approaches in paleobiology now allow such continuous shape data to be used in phylogenetic applications, opening up the possibility of effectively combining stone tool geometric morphometrics with cultural phylogenetics in one workflow. From 26 to 30 September 2022, the authors convened for a workshop with the title “Cultural evolutionary tools for stone tool shape analysis: Geometric morphometrics and Bayesian phylogenetics” at the Aarhus Institute for Advanced Studies, in Aarhus, Denmark. This workshop was held under auspices and with funding from Cultural Evolution Society (https://culturalevolutionsociety.org/) and in direct continuation of the Society's biannual conference. The aim was to stimulate and foster the use and application of whole-outline GMM to questions of cultural evolution, and to begin assembling a data set of stone tools—probable projectile points in the first instance, but other classes of artifacts as well—that may be used to explore these methods and benchmark interpretations. introducing the attendees to the application of reproducible outline based GMM in the programming language R12 for the analysis of 2D stone tools; and assembling an initial data set of lithic projectile point shapes from different times and places that can subsequently be used by the research community for comparative analyses using these or other methods. The specific outline based GMM approach applied in this workshop follows the protocol recently published by Matzig13, 14 who also led the workshop. This approach includes the semi-automated extraction of outlines from legacy data, such as drawings or photographs. Vitally, this protocol relies entirely on open-source software and is, beyond basic image preparation, fully replicable and reproducible. Before the start of the workshop, all participants had prepared their own individual sets of photographs or drawings related to their expertise alongside associated metadata such as geographical coordinates and dating. During the workshop, focus rested initially on how to prepare the images for the extraction of artifact outlines using the open-source imaging software GIMP (http://www.gimp.org) and R. Thereafter, the outline datasets created in this way and ranging from Late Pleistocene Europe and Northern Africa to Holocene North and South America (Figure 2) were analyzed in a multivariate framework closely following the approach of Matzig et al.15 The performance of this methodology has been directly compared to previous published analyses that use both traditional typo-technological attributes as well as those using landmark-based GMM and was shown to capture salient differences in artifact forms where they exist. On the first day of workshop, each participant presented their data set and shared their assumptions regarding the cultural evolutionary processes they sought to test; these hypotheses related variously to chronological and spatial differentiation, or to cultural taxonomic assessments of the material at hand. Each participant's data set and research questions differed substantially in their geographical and chronological scope, and the number of artifacts in each data set also varied. Some datasets were best suited to analyses regarding their diachronic, intra-site patterns of cultural evolution, while for others, patterns on a continental, and temporally deep scale were most pertinent. After each participant's presentation of their datasets and objectives, they completed their metadata sheets with all relevant information. The second day was dedicated to image preparation to a common standard so that these could be transferred into the automated outline extraction protocol. The third and fourth days then focused on the main analytical pipeline, the first steps of which consist of the quantification of the extracted outlines using elliptic Fourier analysis16 and principal component analysis for initial visualization. Then, the resulting data are further interrogated using both hierarchical clustering and disparity analysis. The latter, implemented using the R package dispaRity,17 represents a multivariate measure of variance within a morphometric data set that is comparable to the coefficient of variation (CV) for linear measurements. By quantifying variance, the CV is commonly used in cultural transmission research to infer the dominant modes of social learning related to ancient craft production, including stone tools.18, 19 but see Premo.20 The disparity measures, together with multivariate analyses that reveal internal structure within the stone tool shape data at hand, facilitate interpretations of social transmission and cultural evolution (Figure 3). On the fifth and last day, participants presented their results and discussed them in relation to their a priori expectations. Furthermore, all datasets were combined and analyzed together following the exact same analytical pipeline. With its focus on both conceptual issues as well as data wrangling and analysis, this workshop was intense, productive, and collaborative. Participants walked away with a set of tools to reproducibly analyze 2D lithic outlines. By the same token, the heterogeneity of the data and research questions brought to the table by the participants afforded the occasion to review the analytical workflow's strengths and weaknesses. For most datasets, the hierarchical clustering proved to be a useful tool to visualize the relations between artifact shapes and compare the efficacy of existing classifications. As all analyses were performed in the flexible computing environment of R, mapping or others forms of downstream visualizations can be added in a straightforward manner, all the while retaining reproducibility.21 The final day ended with a stimulating discussion concerning the suitability of the methods to capture tool shape heterogeneity, and raising vital issues such as the orientation criteria for asymmetrical tools, such as backed pieces. Issues of sampling bias and analytical scale were also raised, with the current workflow being best suited to macroarchaeological approaches. Besides these important findings and the training of the participants, the data set collated as part of this workshop is now freely available (https://doi.org/10.5281/zenodo.7757171); relevant metadata are available as Supporting Information alongside this report. We hope that future studies will use, update, and add to these data. In time, such a public repository would be a first step towards the comparative study of cultural evolution at large geographic and chronological scales. The workshop's final discussion revolved around the potential to couple whole-outline GMM with the analysis of specific technological traits, and how to integrate these into emerging phylogenetic applications. So far, phylogenetic analyses of stone projectile points have partitioned artifacts using different traits to capture their key characteristics as well as their shape. Only such trait- and landmark-based GMM have offered an integration with phylogenetic methods.22 Yet, both BEAST23 as well as RevBayes24 in principle allow continuous characters to be used, not least within a Bayesian statistical framework. Thanks to such recent developments, a fuller integration between these powerful quantitative methods for stone tool analysis looms on the horizon. The potential thus emerges that both rich outline shape data can be combined with technological traits under one analytical protocol. The authors gratefully acknowledge the Cultural Evolution Society for funding this workshop. Additional costs were covered by funds made available by the Danish Agency for Higher Education and Science (project COMPARCH, Grant Number 1113-00015B). David N. Matzig and Felix Riede's contributions are part of CLIOARCH, an ERC Consolidator Grant project funded by the European Research Council (ERC) under the European Union's Horizon 2020 research and innovation program (Grant Agreement No. 817564). Mercedes Okumura's research is funded by the São Paulo Research Foundation (FAPESP, Grant No. 2018/23282-5). Astolfo G. M. Araujo's research is funded by the São Paulo Research Foundation (FAPESP, Grant No. 2019/18664-9). Renata P. Araujo's research is funded by a doctoral grant from Brazilian National Council for Scientific and Technological Development (CNPq, Grant No. 142353/2019-1). Alice Leplongeon's research is funded by a postdoctoral grant from the Research Foundation in Flanders (FWO #12U9220N). José R. Rabuñal is supported by Margarita Salas fellowship (MARSALAS21-22) funded by the Spanish Ministry of Universities, the European Union-Next Generation EU and the University of Alicante. The authors also thank the Aarhus Institute of Advanced Studies for hosting all participants during this event. The authors have nothing to report. Please note: The publisher is not responsible for the content or functionality of any supporting information supplied by the authors. Any queries (other than missing content) should be directed to the corresponding author for the article.
更多查看译文
关键词
geometric morphometric analysis,tools,benchmarking methods
AI 理解论文
溯源树
样例
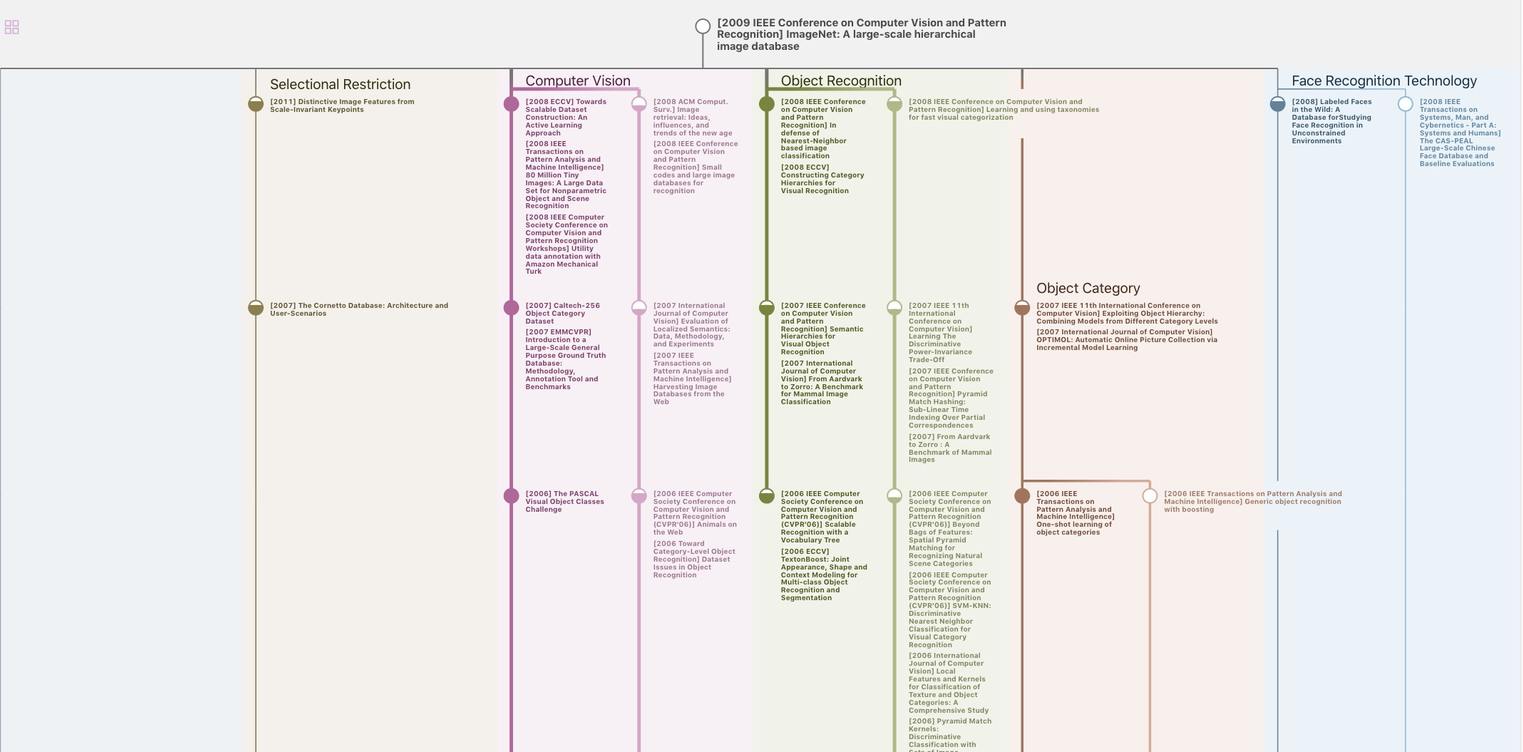
生成溯源树,研究论文发展脉络
Chat Paper
正在生成论文摘要