Are Message Passing Neural Networks Really Helpful for Knowledge Graph Completion?
conf_acl(2023)
摘要
Knowledge graphs (KGs) facilitate a wide variety of applications. Despite great efforts in creation and maintenance, even the largest KGs are far from complete. Hence, KG completion (KGC) has become one of the most crucial tasks for KG research. Recently, considerable literature in this space has centered around the use of Message Passing (Graph) Neural Networks (MPNNs), to learn powerful embeddings. The success of these methods is naturally attributed to the use of MPNNs over simpler multi-layer perceptron (MLP) models, given their additional message passing (MP) component. In this work, we find that surprisingly, simple MLP models are able to achieve comparable performance to MPNNs, suggesting that MP may not be as crucial as previously believed. With further exploration, we show careful scoring function and loss function design has a much stronger influence on KGC model performance. This suggests a conflation of scoring function design, loss function design, and MP in prior work, with promising insights regarding the scalability of state-of-the-art KGC methods today, as well as careful attention to more suitable MP designs for KGC tasks tomorrow.
更多查看译文
关键词
message passing neural networks,knowledge graph
AI 理解论文
溯源树
样例
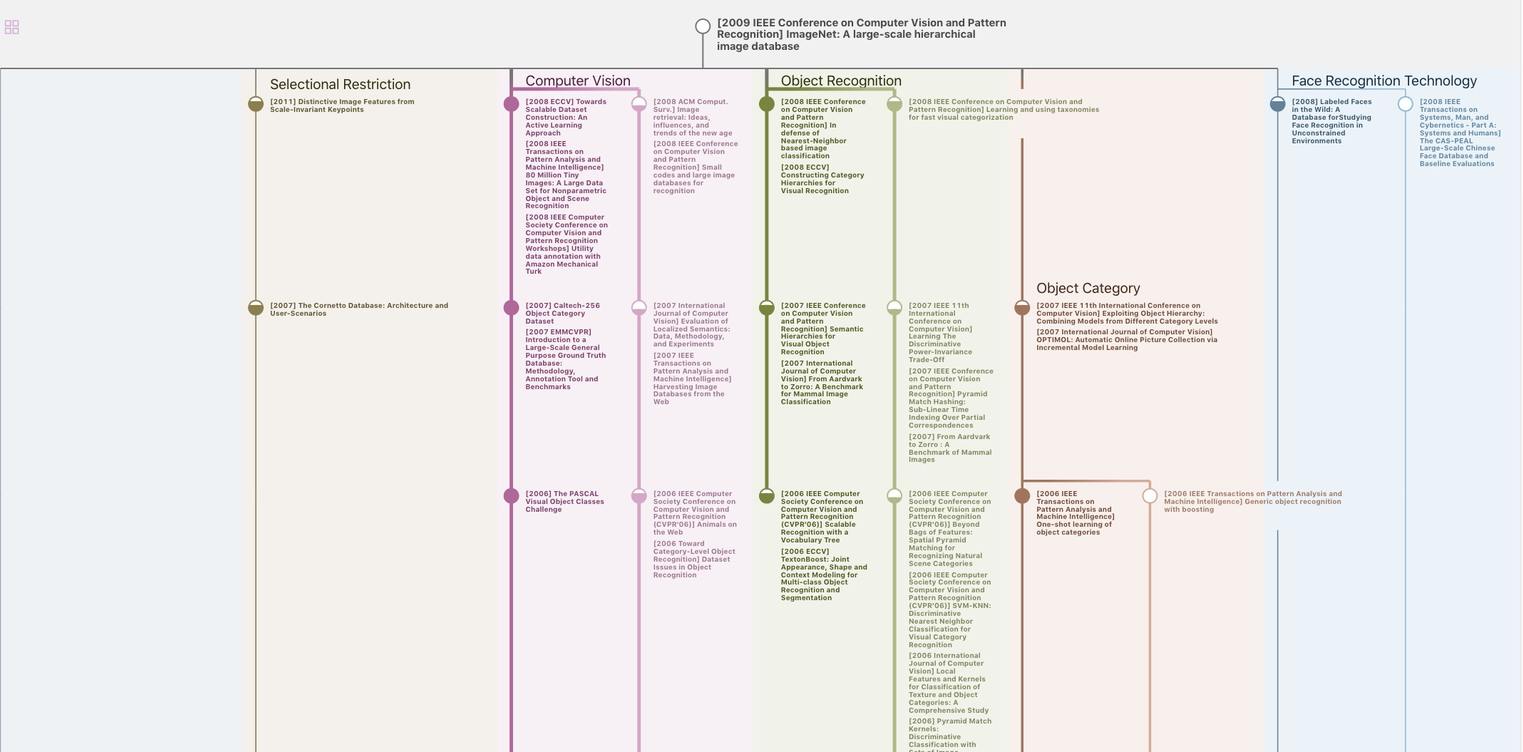
生成溯源树,研究论文发展脉络
Chat Paper
正在生成论文摘要