Interpreting Sentiment Composition with Latent Semantic Tree
conf_acl(2023)
摘要
As the key to sentiment analysis, sentiment composition considers the classification of a constituent via classifications of its contained sub-constituents and rules operated on them. Such compositionality has been widely studied previously in the form of hierarchical trees including untagged and sentiment ones, which are intrinsically suboptimal in our view. To address this, we propose semantic tree, a new tree form capable of interpreting the sentiment composition in a principled way. Semantic tree is a derivation of a context-free grammar (CFG) describing the specific composition rules on difference semantic roles, which is designed carefully following previous linguistic conclusions. However, semantic tree is a latent variable since there is no its annotation in regular datasets. Thus, in our method, it is marginalized out via inside algorithm and learned to optimize the classification performance. Quantitative and qualitative results demonstrate that our method not only achieves better or competitive results compared to baselines in the setting of regular and domain adaptation classification, and also generates plausible tree explanations.
更多查看译文
关键词
sentiment composition,latent semantic tree
AI 理解论文
溯源树
样例
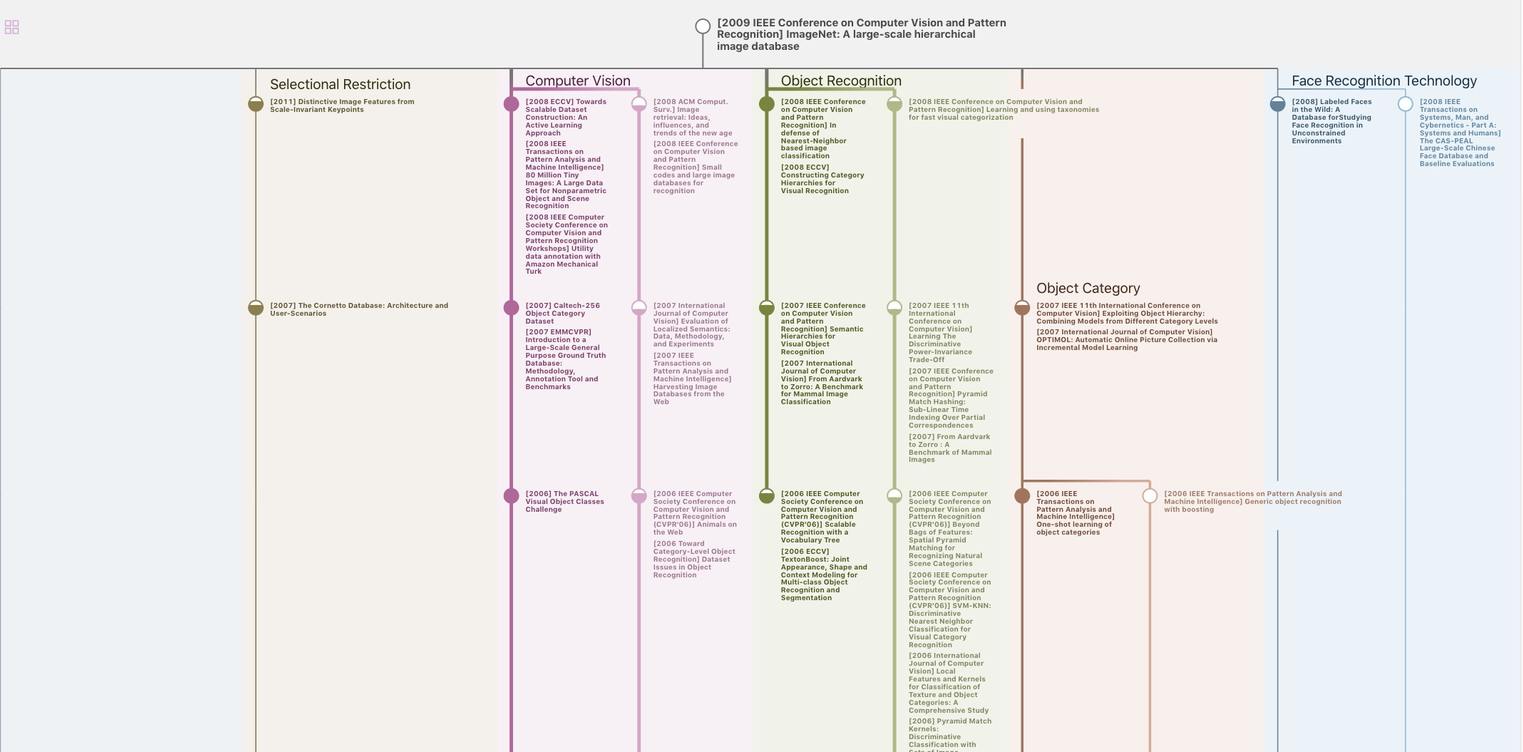
生成溯源树,研究论文发展脉络
Chat Paper
正在生成论文摘要