Towards Better Hierarchical Text Classification with Data Generation
conf_acl(2023)
摘要
Hierarchical text classification (HTC) focuses on classifying one text into multiple labels, which are organized as a hierarchical taxonomy.Due to its wide involution in realistic scenarios, HTC attracts long-term attention from both industry and academia.However, the high cost of hierarchical multi-label annotation makes HTC suffer from the data scarcity problem.In view of the difficulty in balancing the controllability of multiple structural labels and text diversity, automatically generating high-quality data for HTC is challenging and under-explored.To fill this blank, we propose a novel data generation framework tailored for HTC, which can achieve both label controllability and text diversity by extracting high-quality semantic-level and phrase-level hierarchical label information.Experimental results on three benchmarks demonstrate that, compared with existing data augmentation methods, the data generated from our method can bring the most significant performance improvements of several strong HTC models.Extensive analysis confirms that the improvements yielded by our proposed method do correlate to the enhancement of label controllability and text diversity.
更多查看译文
AI 理解论文
溯源树
样例
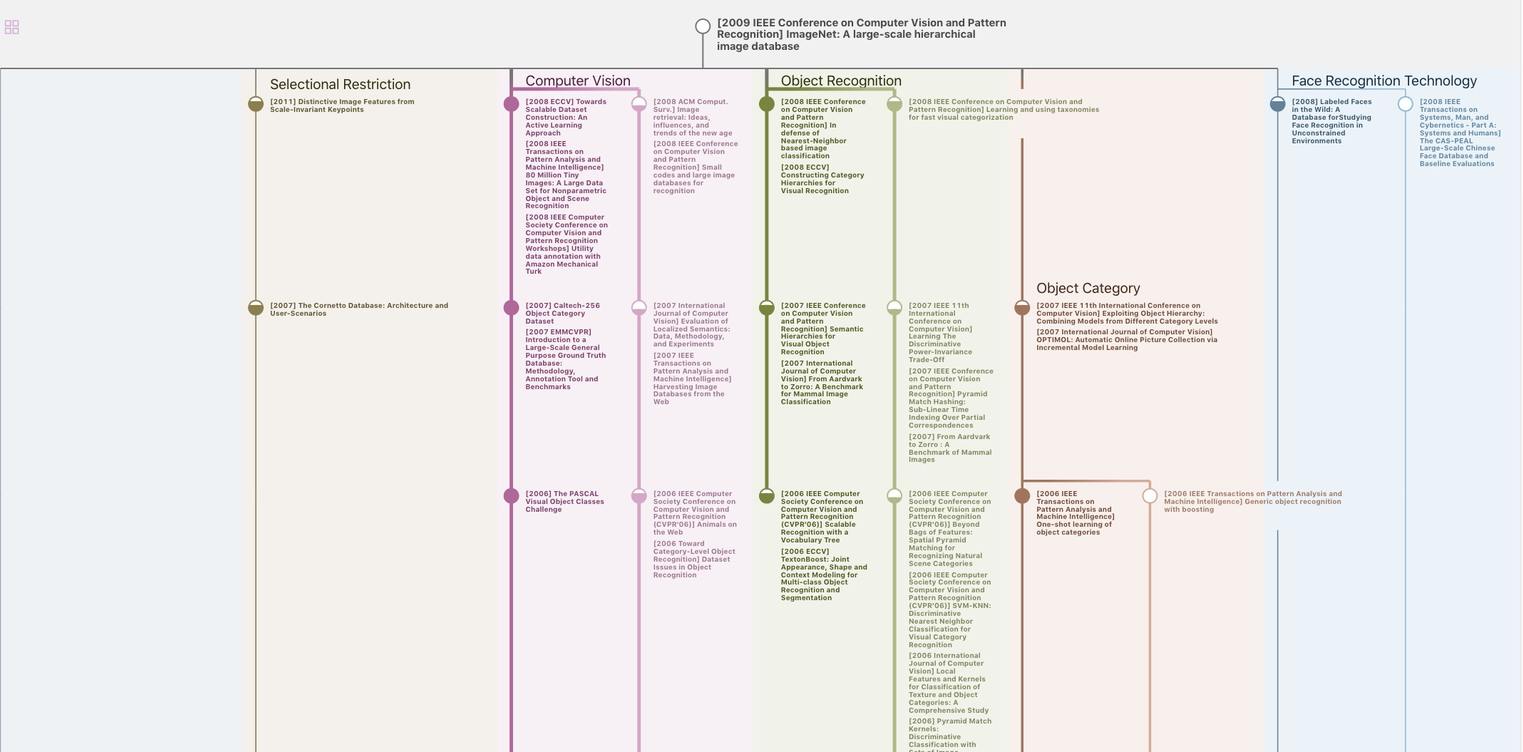
生成溯源树,研究论文发展脉络
Chat Paper
正在生成论文摘要