Combinatorial online high‐order interactive feature selection based on dynamic graph convolution network
Signal Processing(2023)
摘要
•Based on information theory, this paper defines new measurement forms of feature interaction, relevance, and redundancy, and analyzes the relationship between feature correlation and feature interaction.•This paper proposes a combined high-order interactive feature selection method, which includes three components: LO-OIFS, HO-OIFS, and sparse constraint. For LO-OIFS, a strategy for selecting features in pairs is designed to ensure that the selected features are relevant to their corresponding categories and interactive with each other. In the HO-OIFS module, the feature graph convolutional network and pseudo-label dynamic generation mechanism are used to determine the high-order interaction among features. The former can analyze the information between adjacent features, and the latter can explore the similarity between unlabeled features. The combination of the two can improve the reliability of pseudo-labels and help obtain more accurate high-order relationships. The sparse constraint uses regularization and variable selection methods, elastic net, to select part of the feature group and further reduce redundancy.•To verify the effectiveness of the algorithm, this paper selected eleven real-world data sets from the UCI database, NeurIPS 2003 and WCCI 2006 classification challenge. This paper also simulated dynamic feature flow by processing only one feature in each iteration to demonstrate that the CHOIFS-DGS algorithm can dynamically select relevant features and interactive features.
更多查看译文
关键词
Graph convolution network,High-order feature interaction,Online feature selection,Elastic net,Information gain
AI 理解论文
溯源树
样例
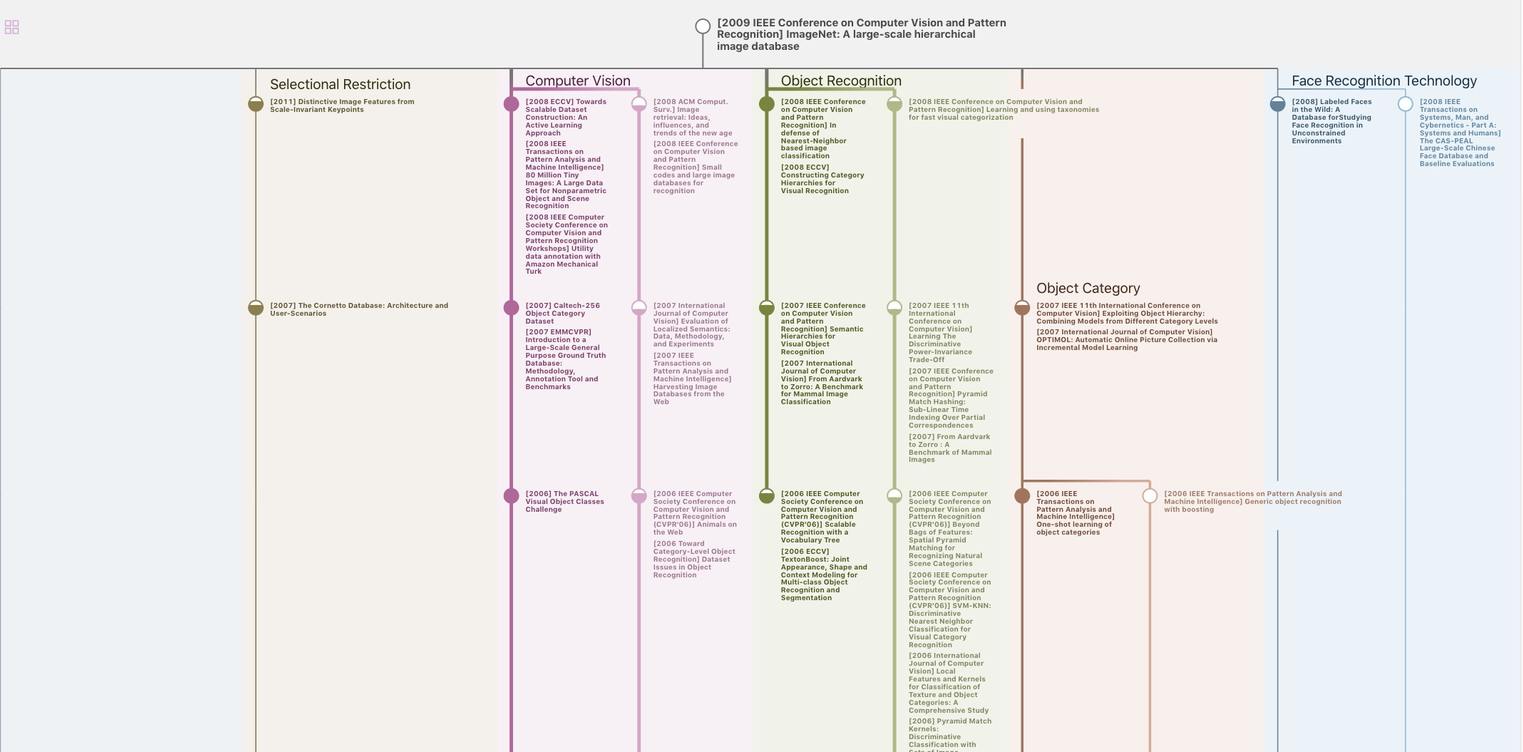
生成溯源树,研究论文发展脉络
Chat Paper
正在生成论文摘要