Searching Density-Increasing Path to Local Density Peaks for Unsupervised Anomaly Detection
IEEE Transactions on Big Data(2023)
摘要
Unsupervised anomaly detection (AD) is a challenging problem in the data mining community. Clustering-based AD methods aim to group normal data points into clusters and then regard a point belonging to none of the clusters as an anomaly. However, they may suffer from the problems of unknown cluster numbers and arbitrary cluster shapes. This paper presents a novel clustering-based AD method named Density-increasing Path (DIP) to tackle these challenges. DIP searches a path for each data point. The path starts at the data point itself, passes through several points with monotonically increasing densities, and ends at a density peak. Further, DIP defines the climbing difficulty of each path by combining the distance and density increment of each step along the path, which can be regarded as the anomaly score of the path starting point. DIP can adaptively decide the number of peaks to address the challenge of unknown cluster numbers. Since DIP requires the path to pass several points rather than directly reaching the peak, it handles arbitrary cluster shapes. We also propose the ensemble DIP to improve prediction accuracy. The experimental results on four synthetic datasets and eleven real-world benchmarks demonstrate that DIP outperforms existing methods.
更多查看译文
关键词
Anomaly detection,clustering analysis,imbalance learning,outlier detection
AI 理解论文
溯源树
样例
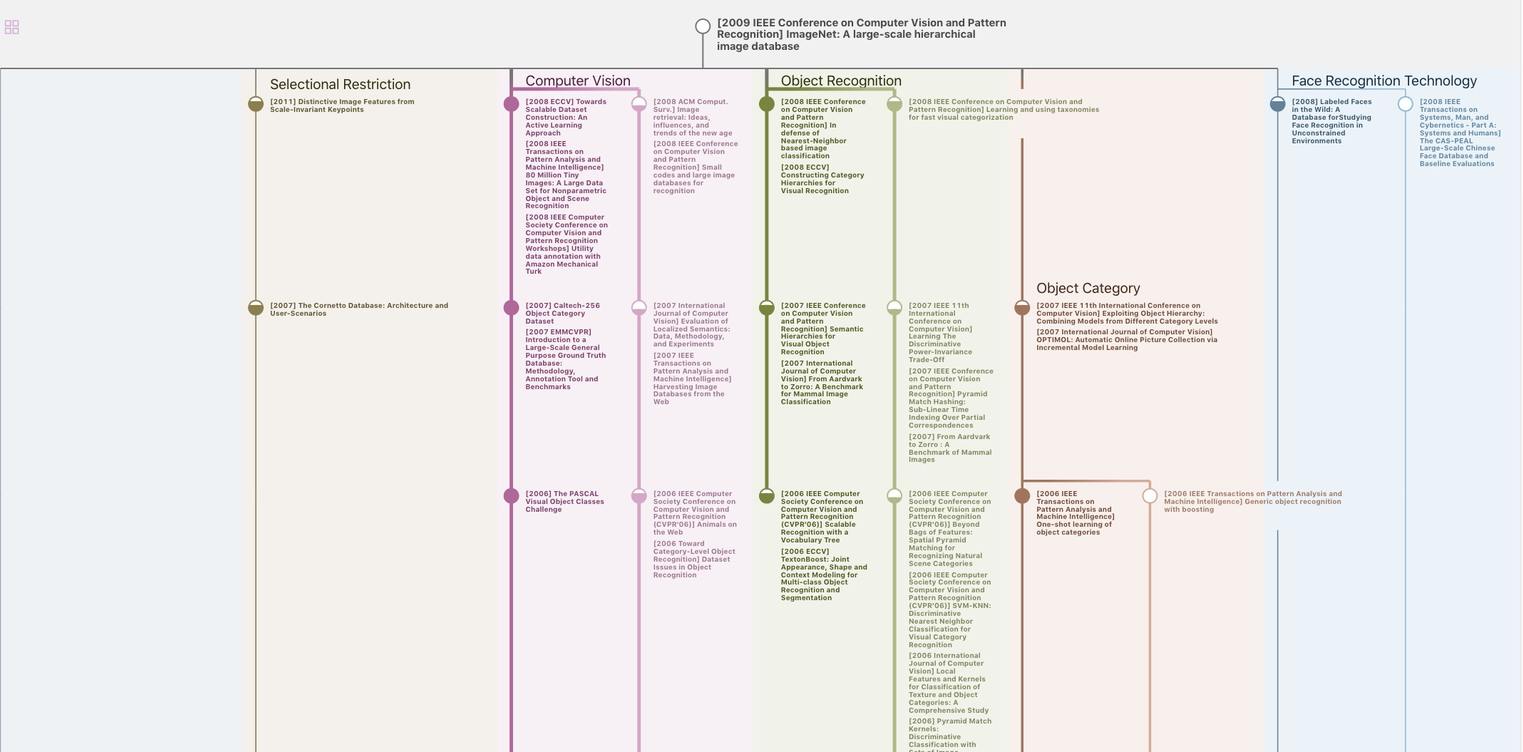
生成溯源树,研究论文发展脉络
Chat Paper
正在生成论文摘要