Automated Diagnosis of Neuromuscular Disorders using EMG Signals
2023 5th International Conference on Bio-engineering for Smart Technologies (BioSMART)(2023)
摘要
Electromyography (EMG) signals are anatomical and physiological properties representation of muscles; their analysis is crucial for diagnosing neuromuscular diseases, and it typically involves the manual inspection of the signals. However, in recent years, experts have developed automatic classification systems to support practitioners and enhance the diagnostic process. In this study, we have used diverse methodologies to extract features from the raw EMG signal in the time and time-frequency domain, using DWT, WPD and a combination of both, with and without preprocessing and for varying levels of decomposition from level 3 to 10, with the support of higher order stats. We have used optimized ensemble tree and optimized SVM classifiers with Bayesian optimization of hyper parameters. The experiments were conducted using the publicly available dataset EMG lab. We got good training results reaching a 100% accuracy from many combinations and testing accuracy of 78.35% when using DWT without preprocessing at level 9 with the linear SVM classifier. The obtained outcomes demonstrate the effectiveness of the suggested method in accurately categorizing the EMG signals. We have also highlighted that the classifier ensemble trees discriminate better between ALS and healthy and doesn’t recognize well Myopathy. Furthermore, the suggested framework has the potential to support clinicians in the diagnosis of neuromuscular disorders.
更多查看译文
关键词
Electromyography (EMG),Myopathy (MYO),Amyotrophic Lateral Sclerosis (ALS),DWT,WPD,SVM
AI 理解论文
溯源树
样例
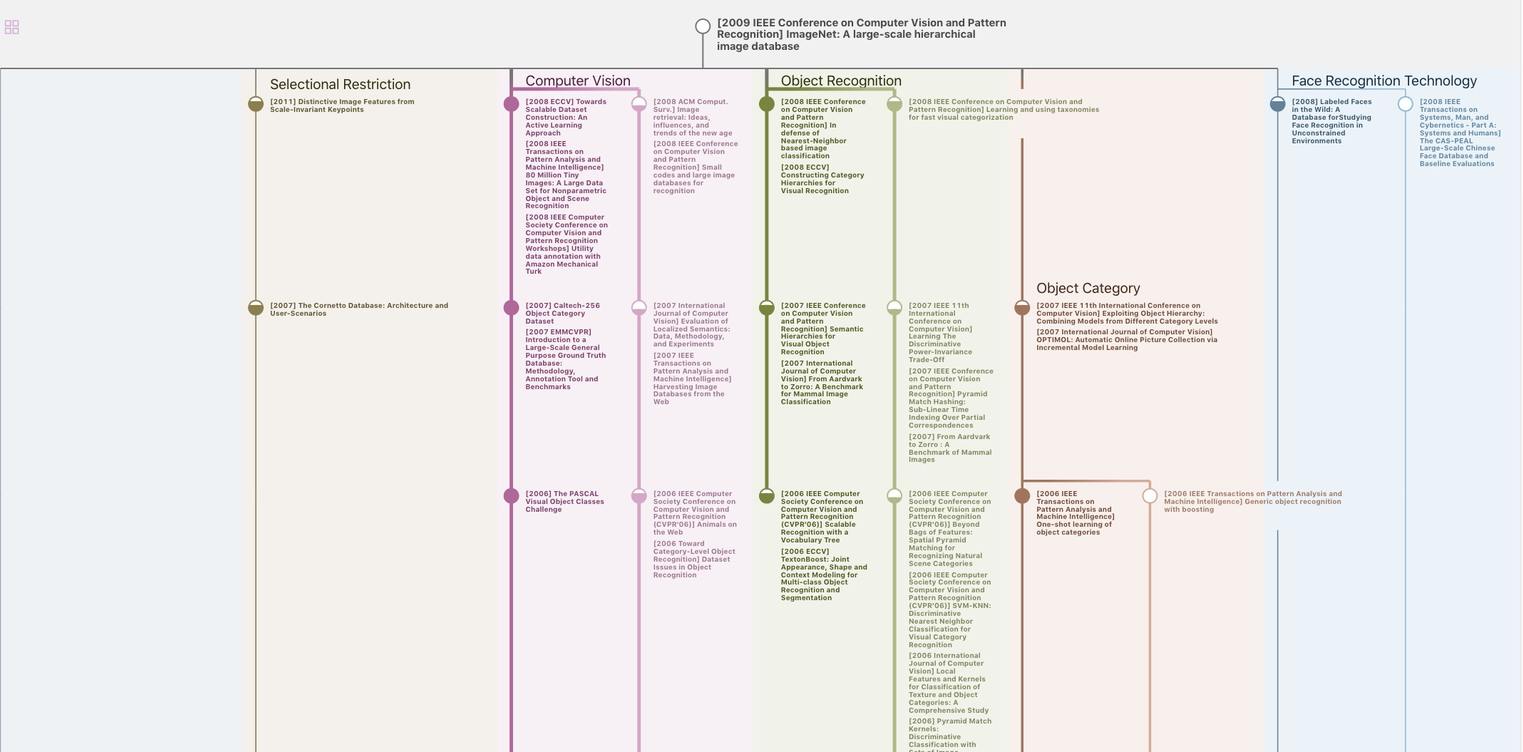
生成溯源树,研究论文发展脉络
Chat Paper
正在生成论文摘要