SPLAL: Similarity-based pseudo-labeling with alignment loss for semi-supervised medical image classification
BIOMEDICAL SIGNAL PROCESSING AND CONTROL(2024)
摘要
Medical image classification presents significant challenges due to limited labeled samples and class imbalance resulting from varying disease prevalence. In many real-world scenarios, a scarcity of labeled images pertaining to specific ailments coexists with an abundance of unlabeled ones. The process of annotating these unlabeled medical images by domain experts is both time-intensive and financially burdensome. Consequently, medical image classification remains an active and evolving research area. Semi-supervised learning (SSL) techniques hold promise in addressing these challenges by effectively leveraging both labeled and unlabeled data. However, the application of SSL to medical image classification necessitates overcoming two primary obstacles: (1) the reliable estimation of pseudo-labels for unlabeled images and (2) the mitigation of biases stemming from class imbalance. In this work, we introduce a novel semi-supervised learning approach, denoted as SPLAL, which adeptly addresses these challenges. SPLAL leverages the concept of class prototypes and employs a weighted combination of classifiers to predict dependable pseudo-labels for a subset of unlabeled medical images. Additionally, we introduce an alignment loss term designed to alleviate model biases, particularly those arising from the overrepresentation of majority classes. This alignment loss mechanism ensures the model's consistency in delivering the same classification output for diverse augmented images of the same disease. To evaluate the effectiveness of our proposed approach, we conducted comprehensive experiments on two publicly accessible benchmark datasets for medical image classification. Our empirical findings unequivocally demonstrate that SPLAL outperforms several state-of-the-art semi-supervised learning methods across various evaluation metrics.
更多查看译文
关键词
Medical image classification,Semi-supervised learning,Medical imaging,Deep learning,Machine learning
AI 理解论文
溯源树
样例
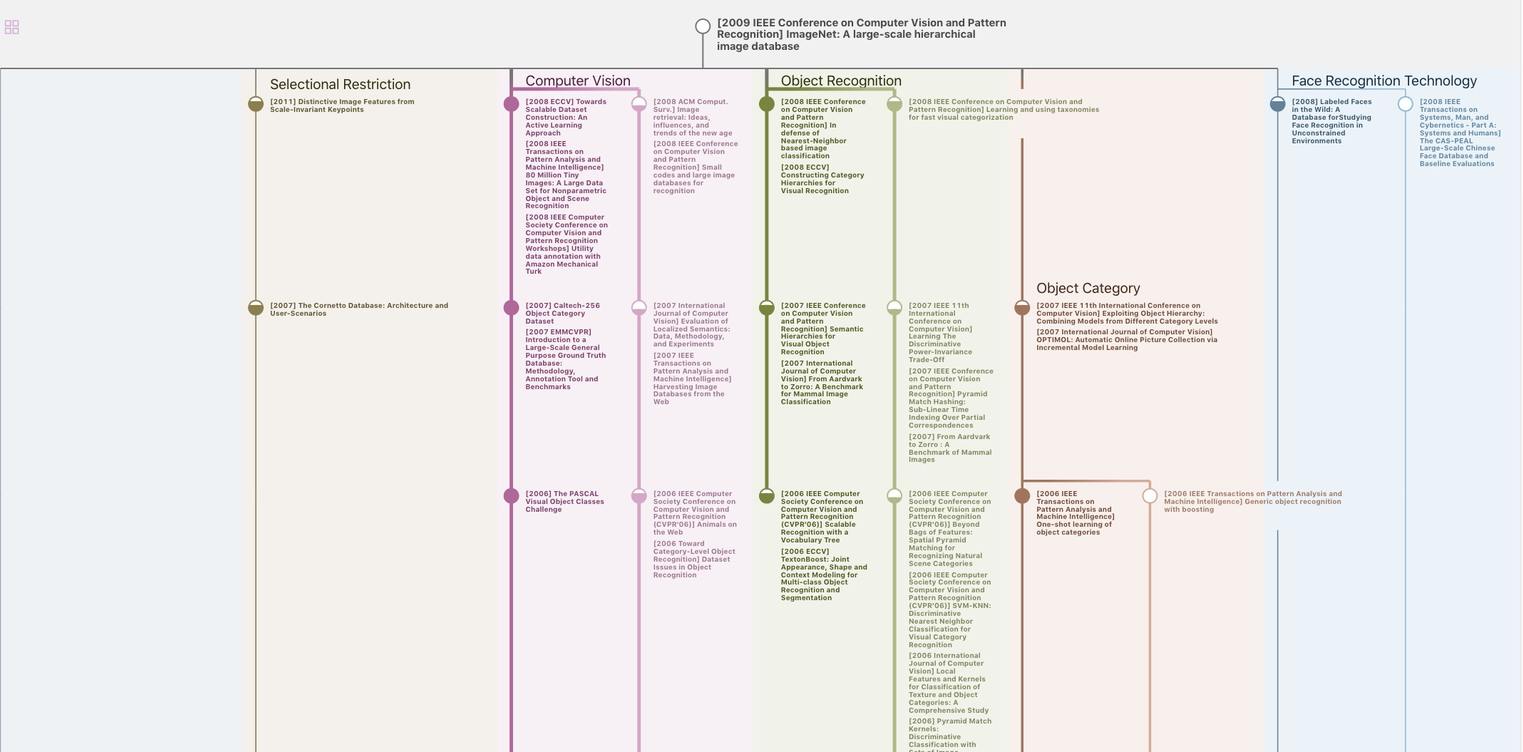
生成溯源树,研究论文发展脉络
Chat Paper
正在生成论文摘要