Learning Interpretable Heuristics for WalkSAT
CoRR(2023)
摘要
Local search algorithms are well-known methods for solving large, hard instances of the satisfiability problem (SAT). The performance of these algorithms crucially depends on heuristics for setting noise parameters and scoring variables. The optimal setting for these heuristics varies for different instance distributions. In this paper, we present an approach for learning effective variable scoring functions and noise parameters by using reinforcement learning. We consider satisfiability problems from different instance distributions and learn specialized heuristics for each of them. Our experimental results show improvements with respect to both a WalkSAT baseline and another local search learned heuristic.
更多查看译文
关键词
interpretable heuristics,walksat,learning
AI 理解论文
溯源树
样例
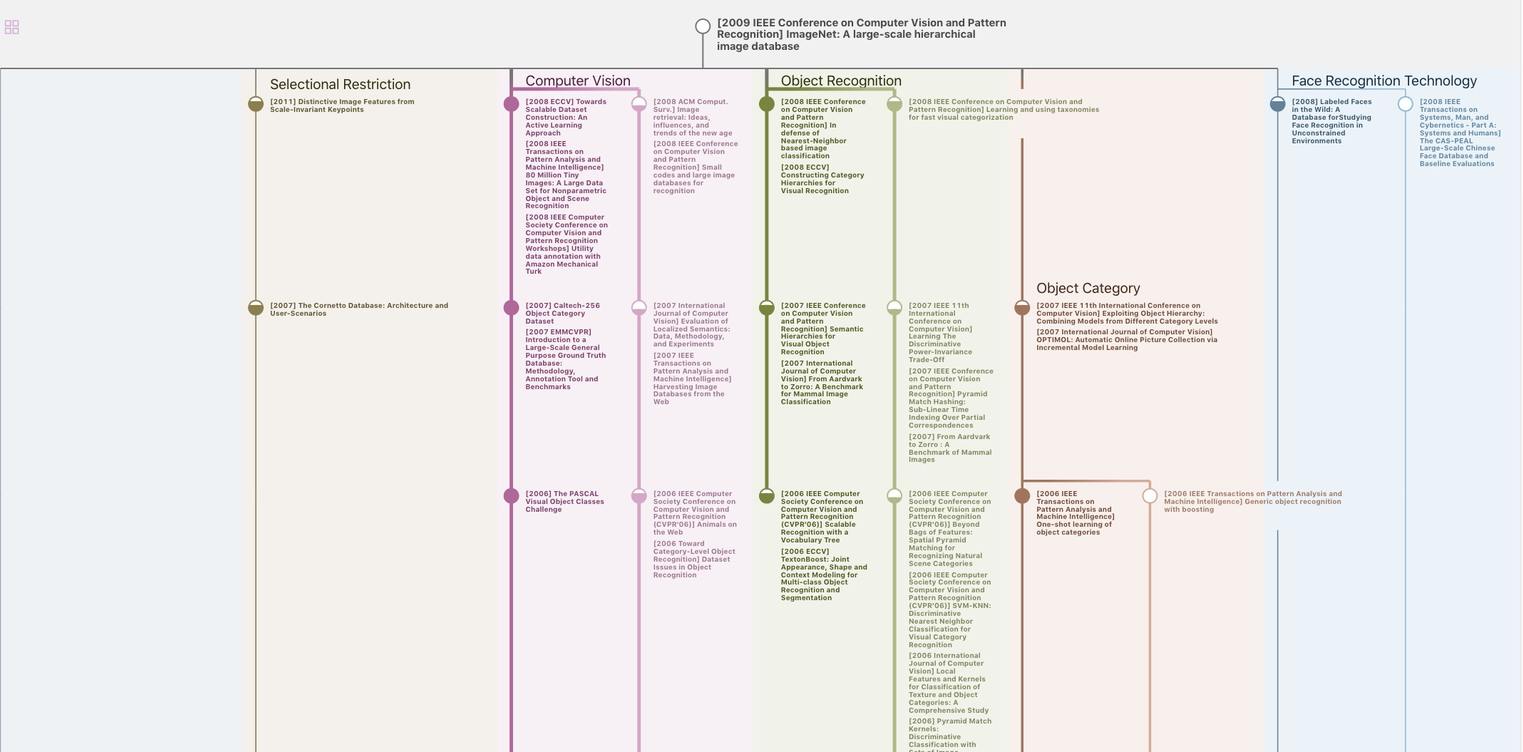
生成溯源树,研究论文发展脉络
Chat Paper
正在生成论文摘要