Towards Assumption-free Bias Mitigation
CoRR(2023)
Abstract
Despite the impressive prediction ability, machine learning models show discrimination towards certain demographics and suffer from unfair prediction behaviors. To alleviate the discrimination, extensive studies focus on eliminating the unequal distribution of sensitive attributes via multiple approaches. However, due to privacy concerns, sensitive attributes are often either unavailable or missing in real-world scenarios. Therefore, several existing works alleviate the bias without sensitive attributes. Those studies face challenges, either in inaccurate predictions of sensitive attributes or the need to mitigate unequal distribution of manually defined non-sensitive attributes related to bias. The latter requires strong assumptions about the correlation between sensitive and non-sensitive attributes. As data distribution and task goals vary, the strong assumption on non-sensitive attributes may not be valid and require domain expertise. In this work, we propose an assumption-free framework to detect the related attributes automatically by modeling feature interaction for bias mitigation. The proposed framework aims to mitigate the unfair impact of identified biased feature interactions. Experimental results on four real-world datasets demonstrate that our proposed framework can significantly alleviate unfair prediction behaviors by considering biased feature interactions.
MoreTranslated text
Key words
assumption-free
AI Read Science
Must-Reading Tree
Example
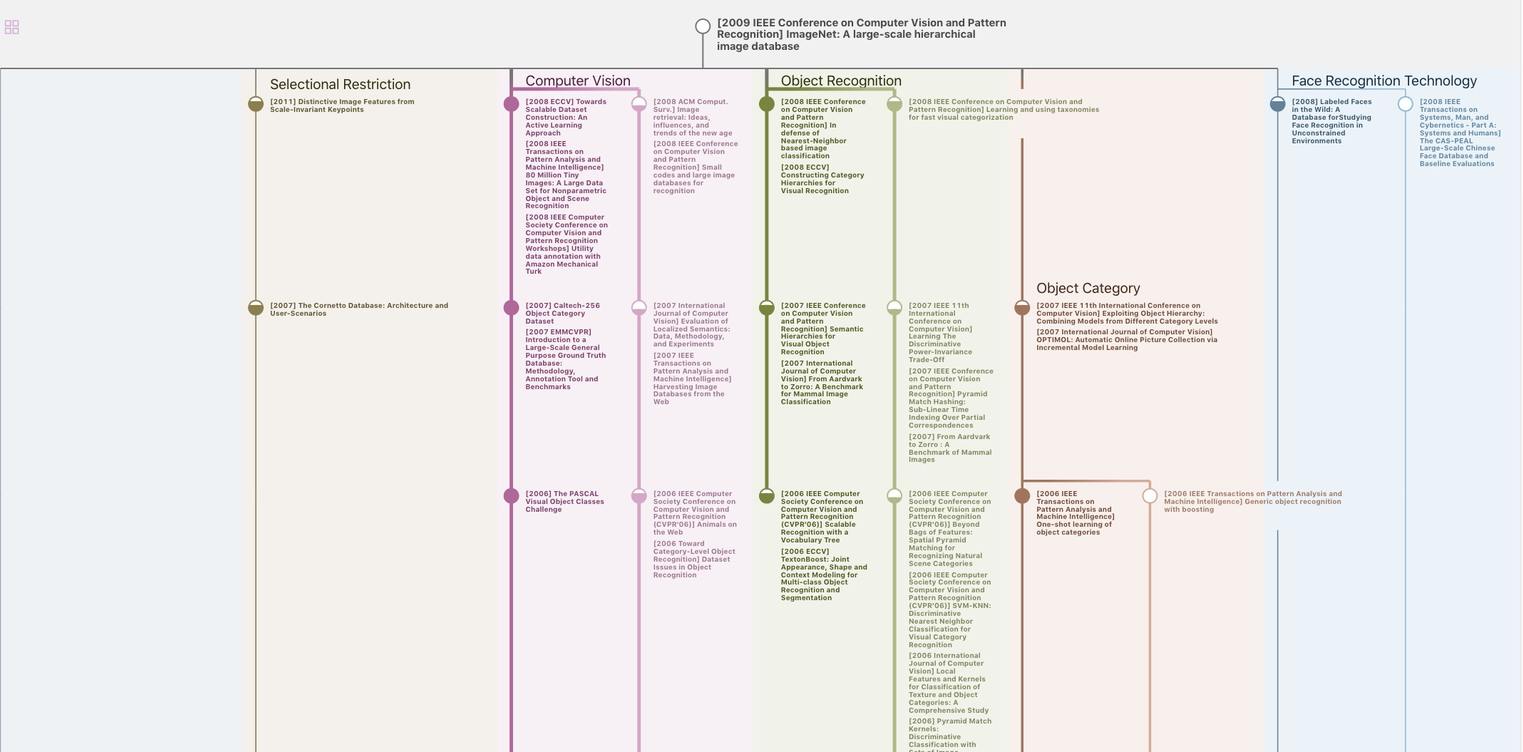
Generate MRT to find the research sequence of this paper
Chat Paper
Summary is being generated by the instructions you defined