PseudoCell: Hard Negative Mining as Pseudo Labeling for Deep Learning-Based Centroblast Cell Detection
CoRR(2023)
摘要
Patch classification models based on deep learning have been utilized in whole-slide images (WSI) of H&E-stained tissue samples to assist pathologists in grading follicular lymphoma patients. However, these approaches still require pathologists to manually identify centroblast cells and provide refined labels for optimal performance. To address this, we propose PseudoCell, an object detection framework to automate centroblast detection in WSI (source code is available at https://github.com/IoBT-VISTEC/PseudoCell.git). This framework incorporates centroblast labels from pathologists and combines them with pseudo-negative labels obtained from undersampled false-positive predictions using the cell's morphological features. By employing PseudoCell, pathologists' workload can be reduced as it accurately narrows down the areas requiring their attention during examining tissue. Depending on the confidence threshold, PseudoCell can eliminate 58.18-99.35% of non-centroblasts tissue areas on WSI. This study presents a practical centroblast prescreening method that does not require pathologists' refined labels for improvement. Detailed guidance on the practical implementation of PseudoCell is provided in the discussion section.
更多查看译文
关键词
centroblast pseudocell detection,hard negative mining,pseudocell labeling,learning-based
AI 理解论文
溯源树
样例
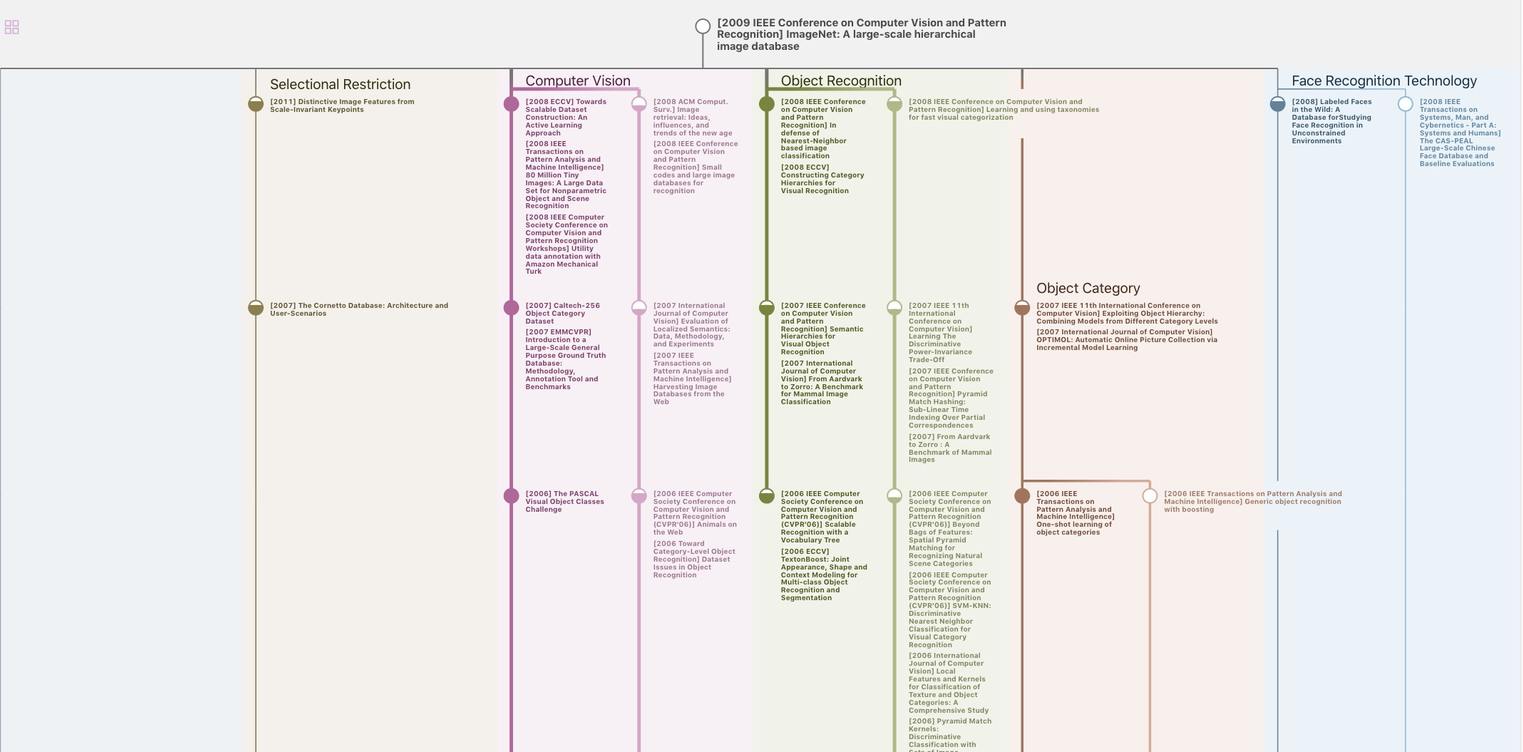
生成溯源树,研究论文发展脉络
Chat Paper
正在生成论文摘要