Multi-robot target entrapment using cooperative hierarchical gene regulatory network
Swarm and Evolutionary Computation(2023)
摘要
For accomplishing a variety of challenging tasks, multi-robot systems perform better than single robots because they have certain properties that a single robot lacks. Target entrapment is one such task; its challenges include finding ways to adapt to different environments to improve entrapment performance. This paper proposes a cooperative hierarchical gene regulatory network (CH-GRN) with the aim of enhancing mutual cooperation between robots neighbours and the utilisation of obstacles to achieve more effective and efficient entrapment. A target–neighbour–obstacle (TNO) pattern generation method is proposed in the upper layer of the CH-GRN design; it integrates the information on targets, neighbours, and obstacles in order to generate more accurate patterns for surrounding the targets. A concentration-vector method is applied in the lower layer of the CH-GRN to enable the robots to adapt quickly to the pattern and thereby complete the entrapment task. At the same time, a proposed obstacle avoidance method is incorporated, which leads to more timely obstacle avoidance. Several simulation experiments are conducted to quantitatively analyse CH-GRN’s performance on the target entrapment task in a variety of environments consisting of different types of obstacles. In addition, experiments with Kilobots are conducted to further evaluate CH-GRN’s effectiveness. The results show that the proposed model can guide a robot swarm to perform target entrapment tasks in challenging environments with a variety of obstacles, such as various shapes obstacles, narrow channel obstacles, and dynamic obstacles.
更多查看译文
关键词
cooperative hierarchical gene,target,multi-robot
AI 理解论文
溯源树
样例
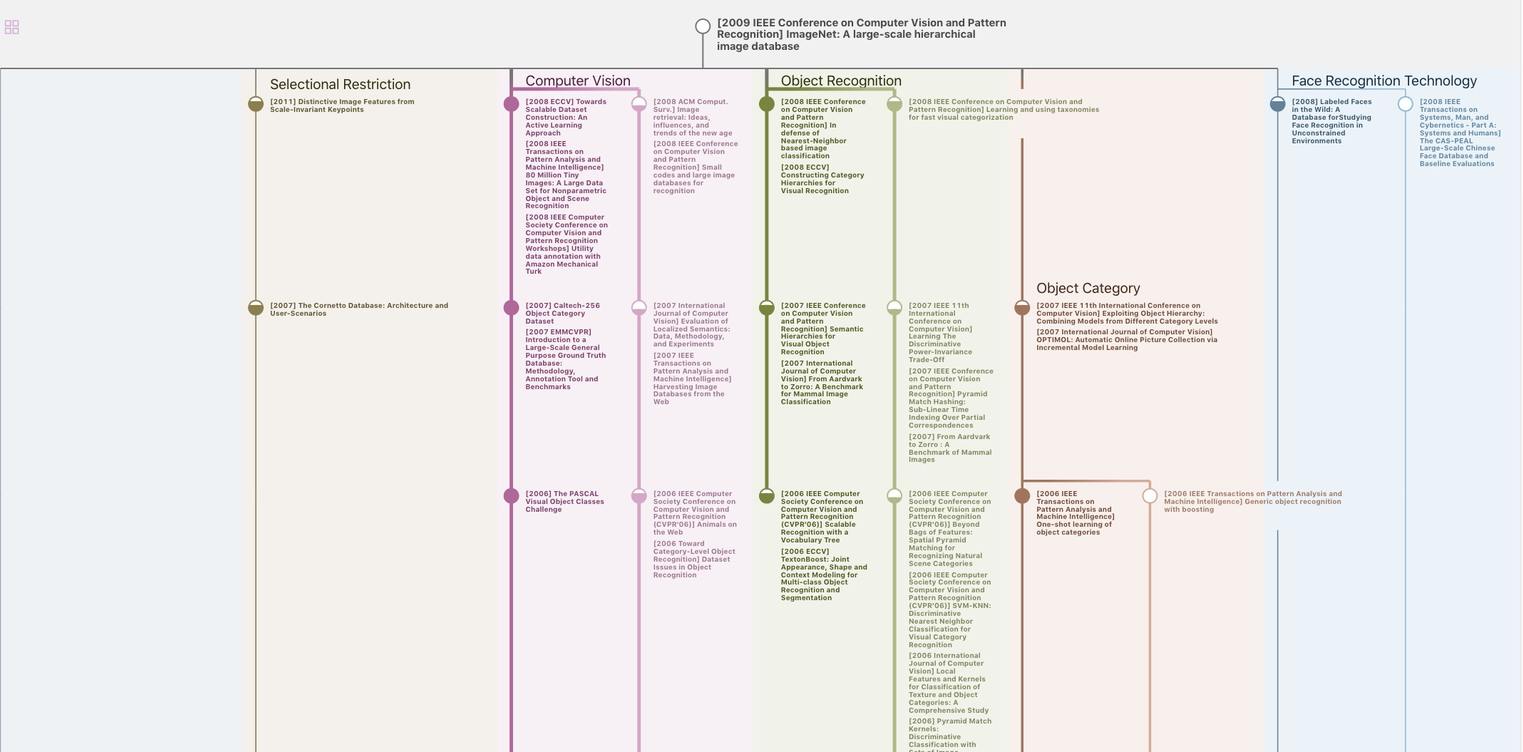
生成溯源树,研究论文发展脉络
Chat Paper
正在生成论文摘要