Feasibility of Monte-Carlo maximum likelihood for fitting spatial log-Gaussian Cox processes
Spatial Statistics(2023)
Abstract
Log-Gaussian Cox processes (LGCPs) are a popular and flexible tool for modelling point pattern data. While maximum likelihood estimation of the parameters of such a model is attractive in principle, the likelihood function is not available in closed form. Various Monte Carlo approximations have been proposed, but these have seen very limited use in the literature and are often dismissed as impractical. This article provides a comprehensive study of the computational properties of Monte Carlo maximum likelihood estimation (MCMLE) for LGCPs. We compare various importance sampling algorithms for MCMLE, and also consider their performance against other methods of inference (such as minimum contrast) in numerical studies. We find that the best MCMLE algorithm is a practical proposition for parameter estimation given modern computing power, but the performance of this methodology is rather sensitive to the choice of reference parameters defining the importance sampling distribution.
MoreTranslated text
Key words
monte-carlo,log-gaussian
AI Read Science
Must-Reading Tree
Example
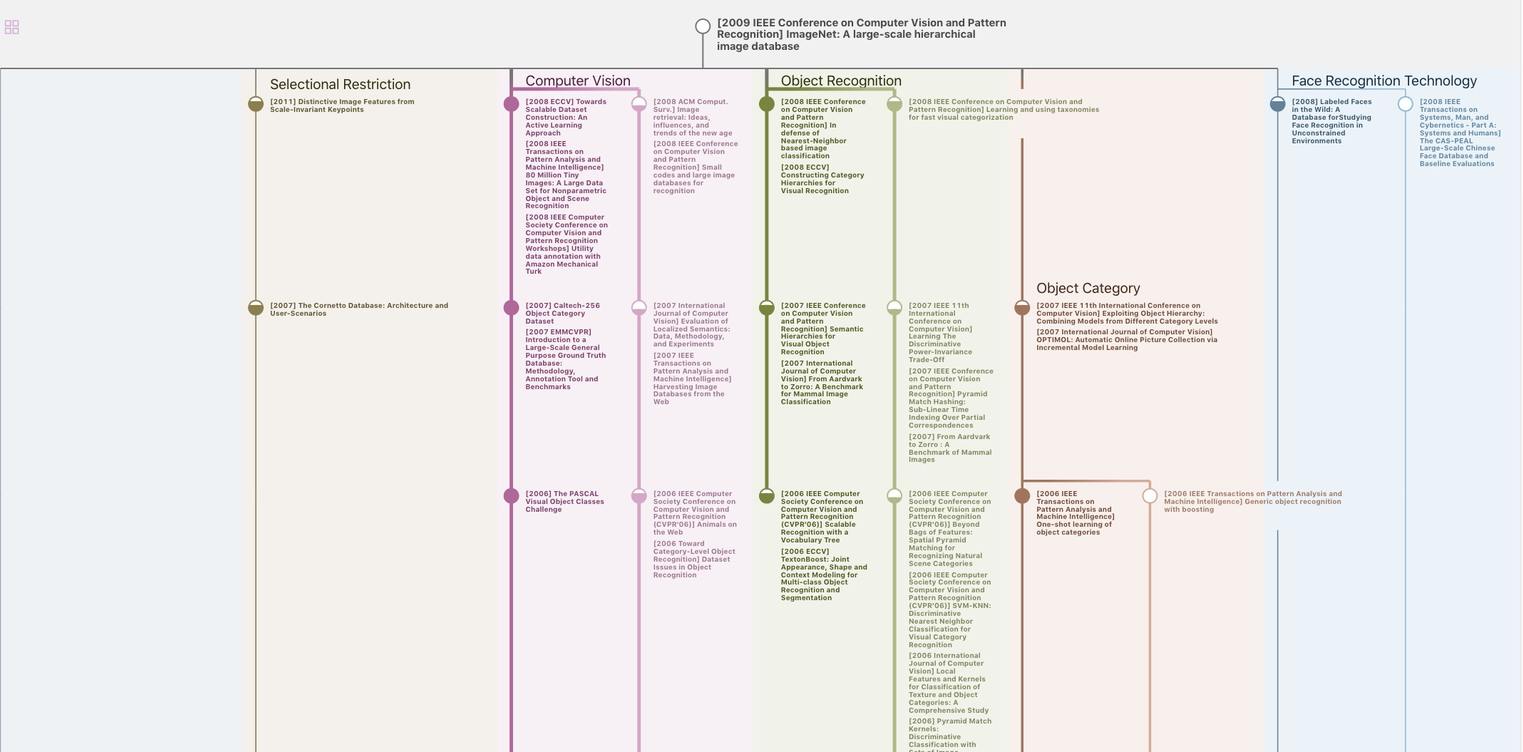
Generate MRT to find the research sequence of this paper
Chat Paper
Summary is being generated by the instructions you defined