Level-set evolution for medical image segmentation with alternating direction method of multipliers
Signal Processing(2023)
Abstract
Segmentation via level-set methods plays a significant role in natural and medical image analysis. Recently, the distance regularized level-set evolution (DRLSE) model, which is based on variational level-set formulation has been proven very useful. However, the DRLSE model suffers from difficulties related to its computational cost due to the sign distance function (SDF) and Courant-Friedrichs-Lewy (CFL) condition applied by the gradient descent method. This paper analyzes and tests the well-known fast computing algorithm known as alternating direction method of multipliers (ADMM) for level-set based image segmentation. That is, we compute the DRLSE model by using the ADMM algorithm. The comparison between the classical gradient descent method and the proposed ADMM for two-phase and multi-phase level-set formulation is provided. The numerical experiments on standard public data sets, particularly in the case of medical image segmentation, demonstrate the effectiveness and efficiency of the proposed method. The average segmentation coefficients for Dice and Jaccard against ground truth are 0.97 and 0.92, respectively, and the average running time is 1.70 seconds. 0.0932, 0.993, 0.981, and 0.964 are average estimated values for mean absolute distance (MAD), accuracy, sensitivity, and specificity, respectively. The source code can be found at link https://github.com/WaliSamad/DRLSE-ADMM-Medical-Image-Segmentation.
MoreTranslated text
Key words
Level-set evaluation,Variational models,Image segmentation,Alternating direction method of multipliers,Medical image analysis
AI Read Science
Must-Reading Tree
Example
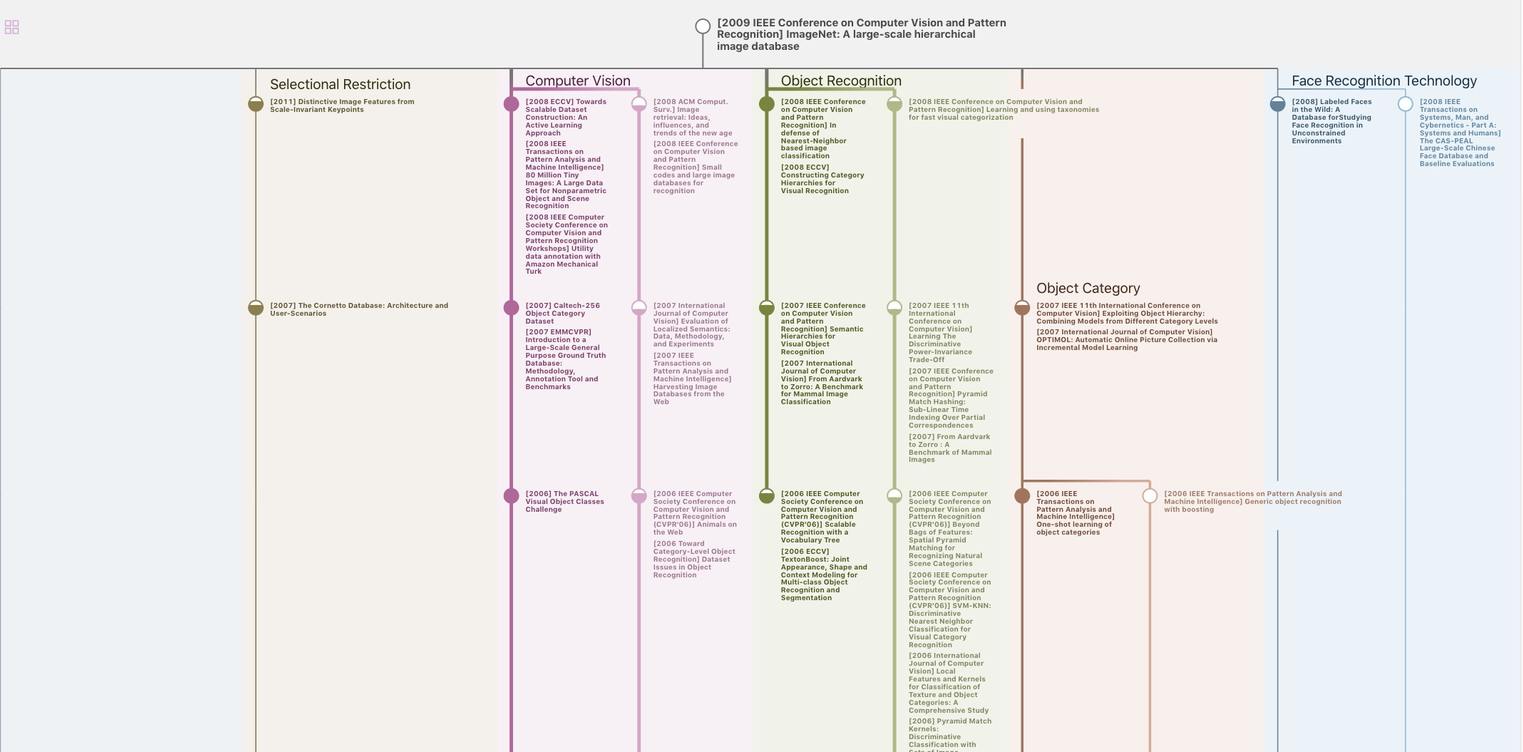
Generate MRT to find the research sequence of this paper
Chat Paper
Summary is being generated by the instructions you defined