Harvesting spatiotemporal correlation from sky image sequence to improve ultra-short-term solar irradiance forecasting
Renewable Energy(2023)
摘要
With photovoltaic power being increasingly integrated into power grid, accurately forecasting solar irradiance is of critical for ensuring stable and economical operation of power systems. The forecast accuracy in ultra-short-term horizons can be greatly improved by employing ground-based sky images. Although wide range of computer vision methods have been used for modelling, effectively extracting spatiotemporal features from sky image sequence is still a tough task. In this study, a sparse spatiotemporal feature descriptor is introduced to enhance the process of dynamic spatiotemporal information extraction from continuous grayscale sky images, while spatial pyramid pooling is used for feature refinement. Parallelly, dense convolutional network is used to extract static features from the nearest single-frame RGB sky images. Both dynamic and static spatiotemporal features were adequately extracted and subsequently fused for the multi-step prediction of global horizontal irradiance. In addition, various competitive models in object detection are adopted as benchmarks for comparison. The experimental results revealed that the proposed method outperformed baseline models, with up to 5.51% reduction on normalized root mean square error (NRMSE) and 9.38% improvement on ramp event forecast. The proposed method can be widely applied to photovoltaic stations equipped with all-sky-imagers.
更多查看译文
关键词
sky image sequence,spatiotemporal correlation,ultra-short-term
AI 理解论文
溯源树
样例
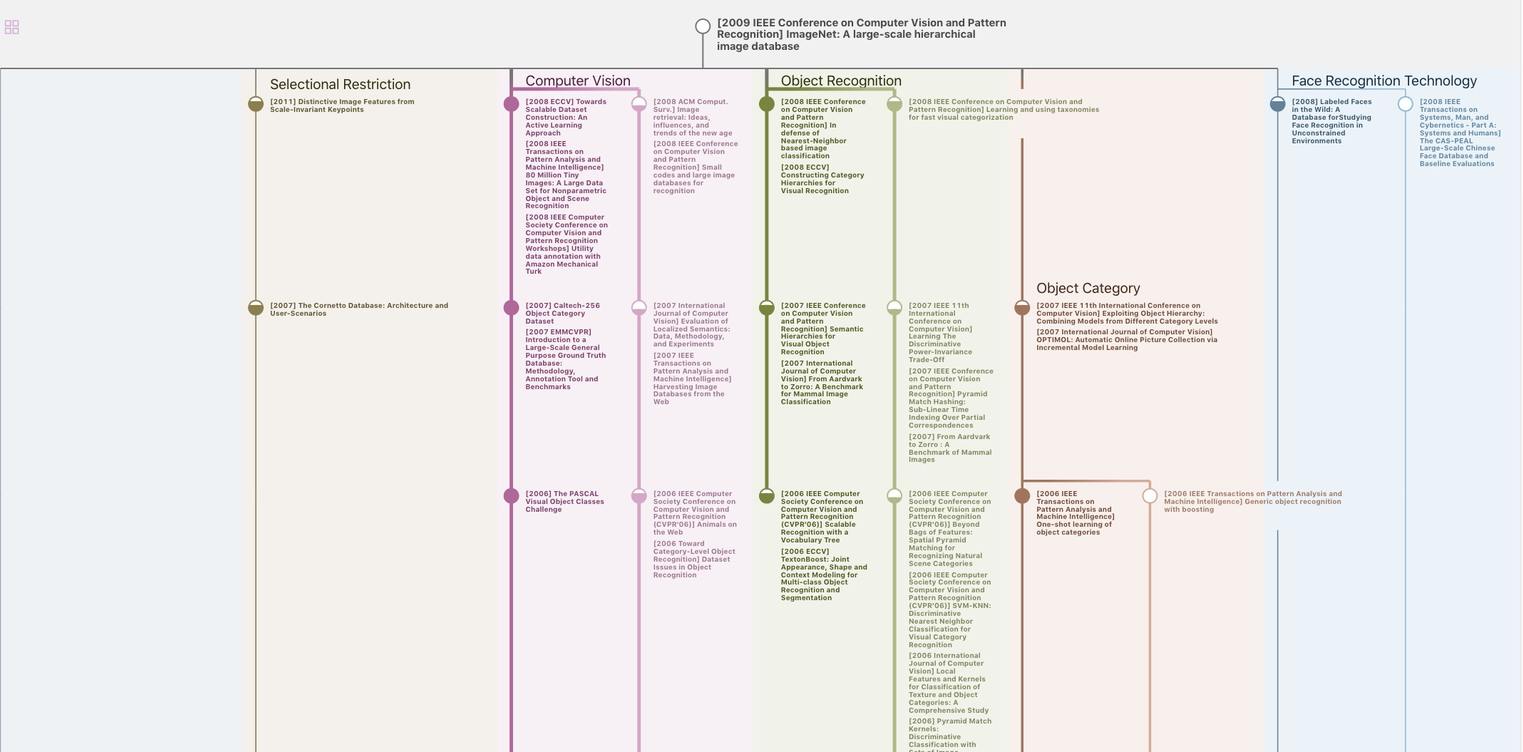
生成溯源树,研究论文发展脉络
Chat Paper
正在生成论文摘要