Impact of the model error on the neural network-based damage detection
Procedia Structural Integrity(2023)
摘要
Machine Learning (ML) techniques applied to vibration-based damage detection of structures showed promising results in identifying damage for their capability of feature discrimination even in presence of noise-corrupted data. In this context, the paper presents the application of a damage detection procedure based on neural networks to a railway bridge and proposes a procedure to take into account for the model error in the training phase. The output of the network, that depends on the dynamic features given as input, allows to classify the structure state as undamaged, lightly damaged or severely damaged. The procedure employs only simulated data but includes a series of expedients to approach a real situation, like the stochastic modelling of measurement errors and the use of two different models to account for the model error. The performances of the networks are analyzed with respect to datasets generated by the two different models. The results show the relevance of accounting for model error in the calibration of the network to obtain a robust damage identification.
更多查看译文
关键词
damage detection,neural,model error,network-based
AI 理解论文
溯源树
样例
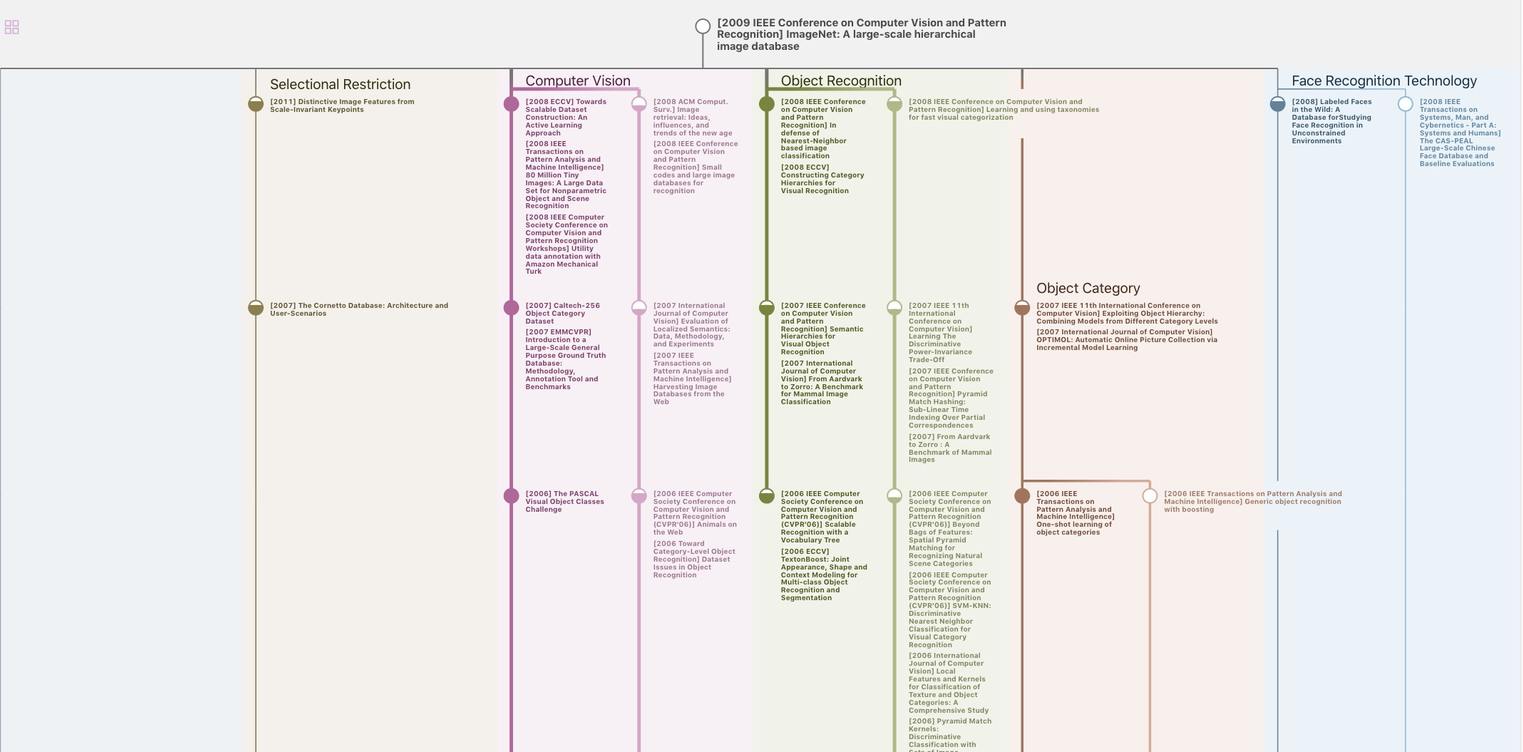
生成溯源树,研究论文发展脉络
Chat Paper
正在生成论文摘要