Integration of machine learning models and metaheuristic algorithms for predicting compressive strength of waste granite powder concrete
Materials Today Communications(2023)
摘要
The utilization of Granite Powder (GP), a by-product of granite processing, has arisen great attention due to environmental challenge posed by its casual disposal. To promote reasonable use of GP in concrete and obtain optimal ratio design of Granite Powder Concrete (GPC), this study explored four advanced machine learning models (Support Vector Machine (SVM), eXtreme Gradient Boosting (XGB), Random Forest (RF), and Multi-Layer Perceptron (MLP)) integrated with two novel metaheuristic algorithms (Salp Swarm Algorithms (SSA) and Ant Lion Optimizer (ALO)) to predict the compressive strength of GPC. The models consider seven effect factors on the compressive strength: cement, water, fine aggregate, granite powder, superplasticizer, coarse aggregates, and curing age. The comprehensive score ranking system was utilized four evaluation metrics to assess models' performance: Coefficient of Determination (R2), Variance Accounted For (VAF), Mean Absolute Error (MAE), and Mean Square Error (MSE). In addition, Taylor diagrams and Regression Error Characteristic (REC) curves were used to compare the performance of the models. The results showed that the XGB-based hybrid model outperformed the other methods, with an optimal model of ALO-XGB achieving a high level of accuracy in predicting compressive strength. Furthermore, the study highlights the importance of understanding the interplay between various factors and their effect on the mechanical properties of GPC, which can inform the development of more sustainable and environmentally friendly building materials.
更多查看译文
关键词
Granite powder,Green concrete,AI,Machine learning,Sustainable development
AI 理解论文
溯源树
样例
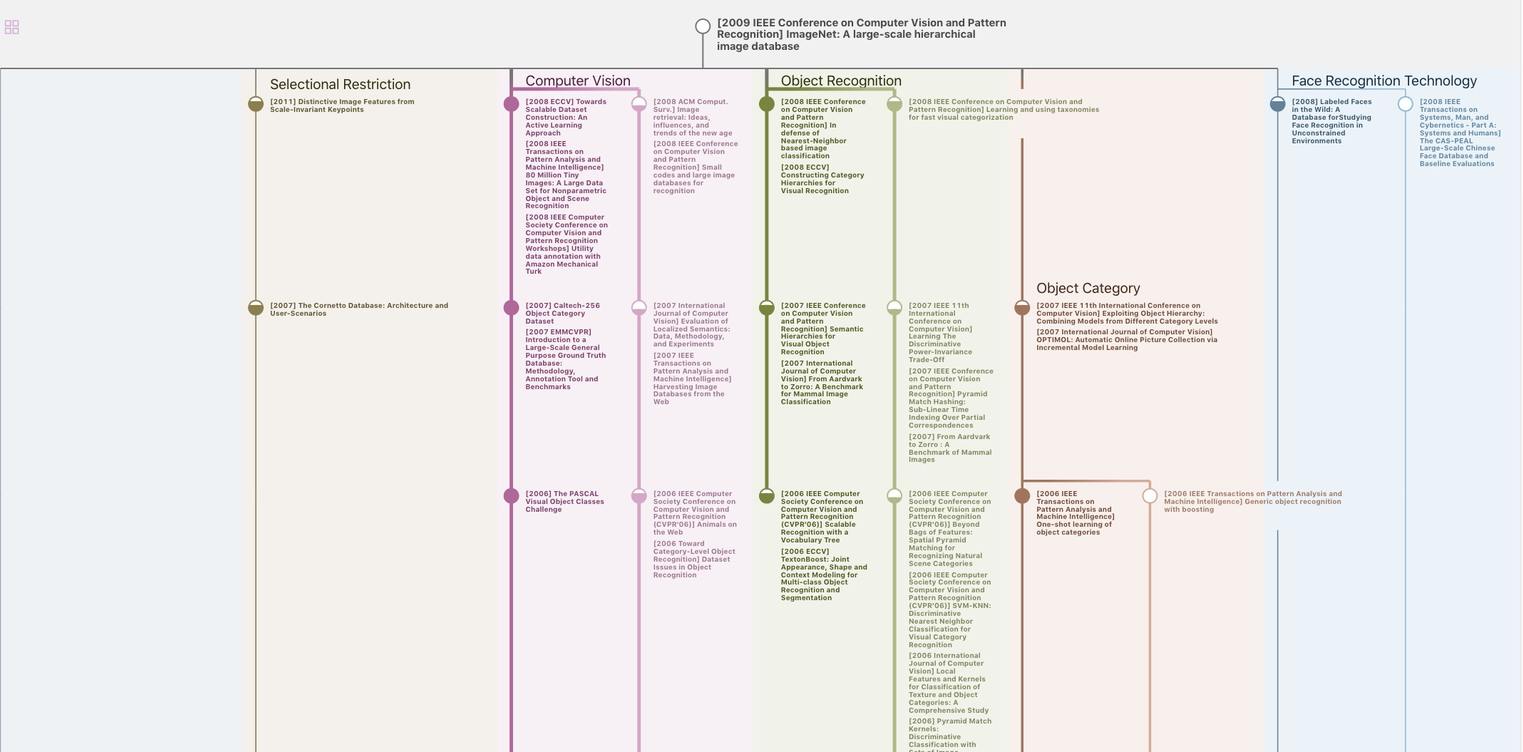
生成溯源树,研究论文发展脉络
Chat Paper
正在生成论文摘要