Traffic Congestion Prediction Based on Multivariate Modelling and Neural Networks Regressions
Procedia Computer Science(2023)
摘要
Smart traffic congestion reduction is actually a real challenge for big cities. Machine learning algorithms can play a significant role in traffic analysis, congestion prediction, and rerouting. In this paper, we propose a new prediction approach to reduce the traffic congestion problem by studying a scheme for predicting traffic flow information using four machine learning techniques: Feed Forward Neural Networks (FFNN), Radial Basis Function Neural Networks (RBFNN), simple linear regression model, and polynomial linear regression model. This prediction scheme is based on the following parameters: the average waiting time at entry and exit street pairs, the days of the week, hours of movement, holidays, and the rain rate. The results indicate that the FFNN technique overcomes the other techniques producing 97.6% prediction accuracy.
更多查看译文
关键词
Traffic congestion,Machine Learning (ML),Radial Basis Function Neural Network (RBFNN),linear regression
AI 理解论文
溯源树
样例
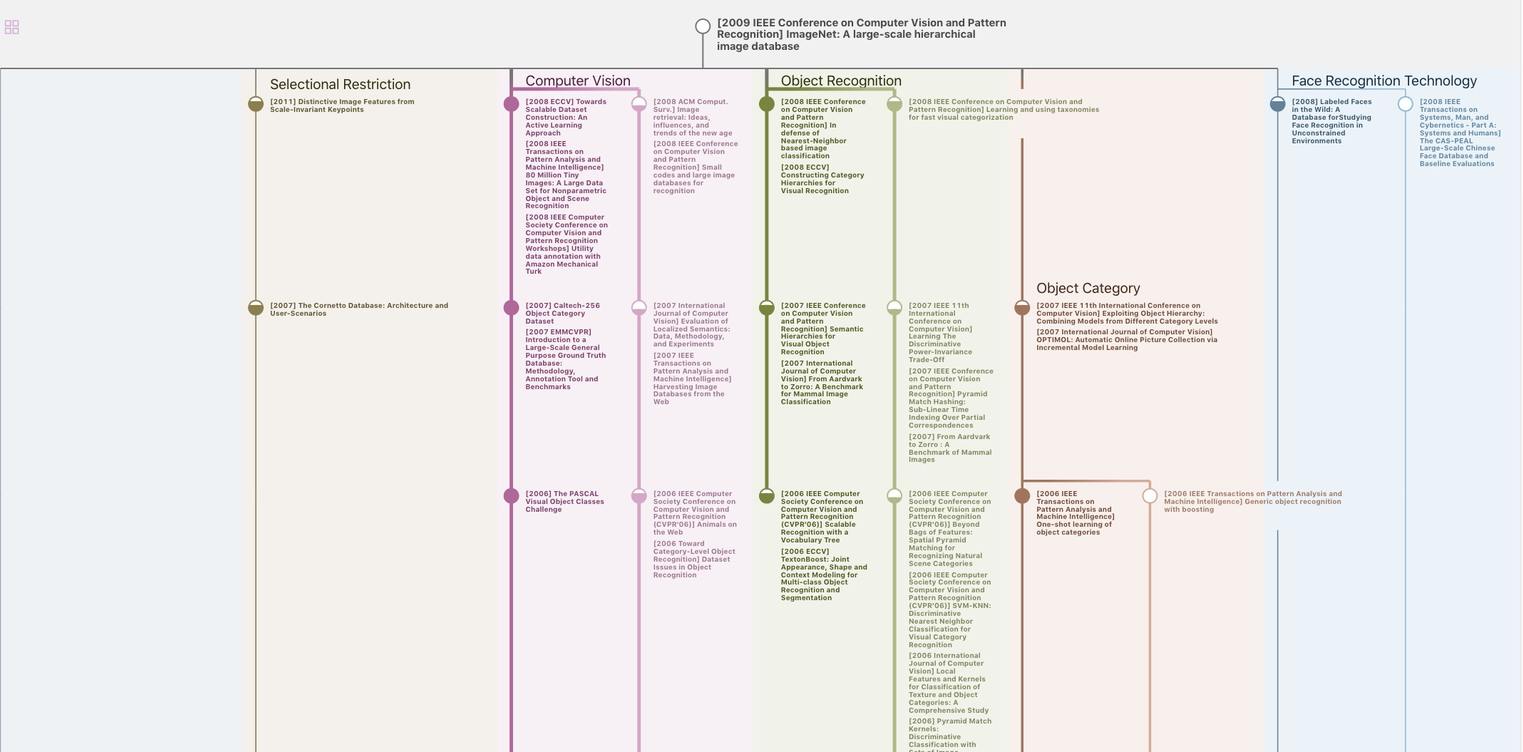
生成溯源树,研究论文发展脉络
Chat Paper
正在生成论文摘要