Deep reinforcement learning based relaying for buffer-aided cooperative communications
Physical Communication(2023)
Abstract
The advances in deep reinforcement learning (DRL) have shown a great potential in solving physical layer-related communication problems. This paper investigates DRL for the relay selection in buffer-aided (BA) cooperative networks. The capability of DRL in handling highly-dimensional problems with large state and action spaces paves the way for exploring additional degrees-of-freedom by relaxing the restrictive assumptions around which conventional cooperative networks are usually designed. This direction is examined in our work by advising and analyzing advanced DRL-based BA relaying strategies that can cope with a variety of setups in multifaceted cooperative networks. In particular, we advise novel BA relaying strategies for both parallel-relaying and serial-relaying systems. For parallel-relaying systems, we investigate the added value of merging packets at the relays and of activating the inter-relay links. For serial-relaying (multi-hop) systems, we explore the improvements that can be reaped by merging packets and by allowing for the simultaneous activation of sufficiently-spaced hops. Simulation results demonstrate the capability of DRL-based BA relaying in achieving substantial improvements in the network throughput while the adequate design of the reward/punishment in the learning process ensures fast convergence speeds.
MoreTranslated text
Key words
Cooperative networks, Relaying, Buffers, Reinforcement learning, 5G networks
AI Read Science
Must-Reading Tree
Example
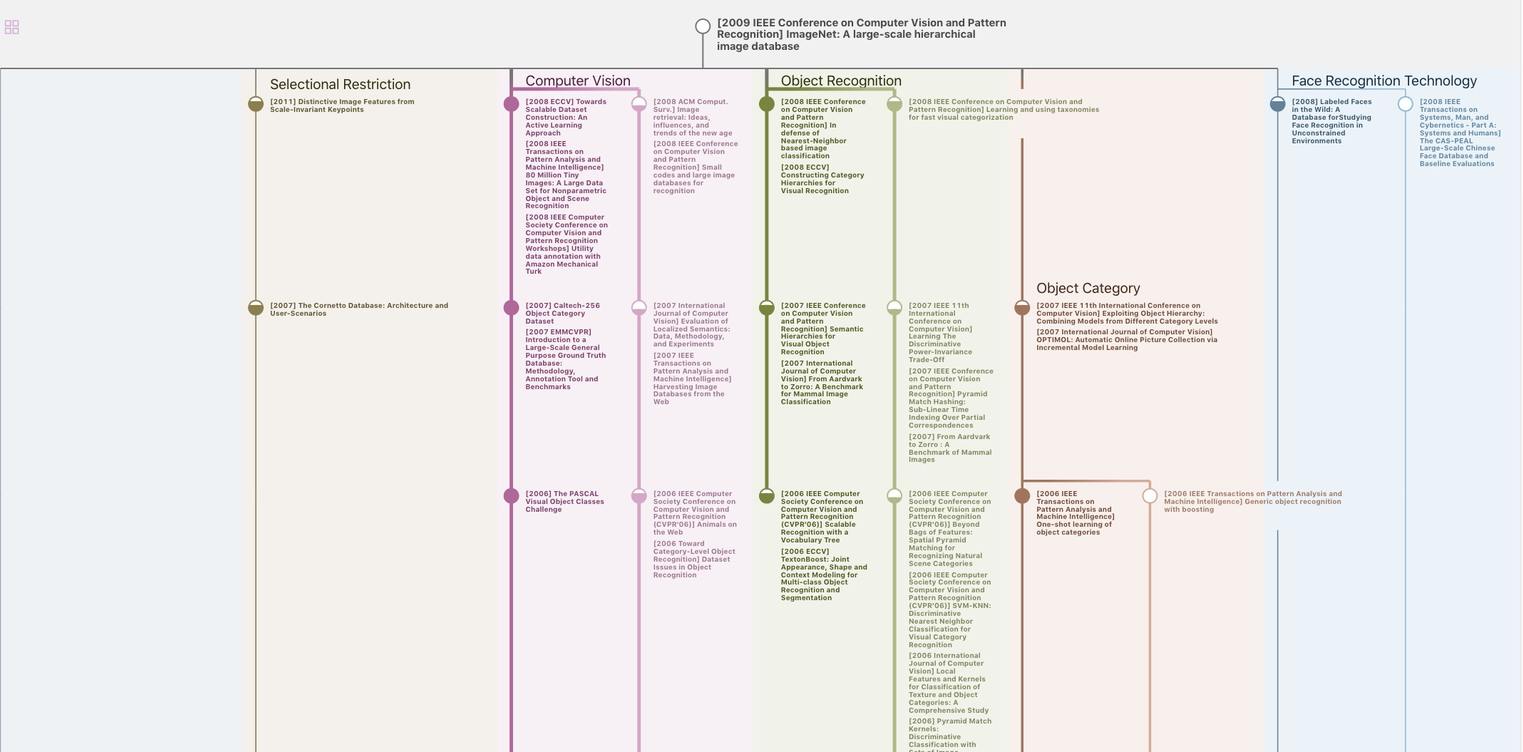
Generate MRT to find the research sequence of this paper
Chat Paper
Summary is being generated by the instructions you defined