CPNet: Conditionally parameterized graph convolutional network for traffic forecasting
Physica A: Statistical Mechanics and its Applications(2023)
Abstract
Traffic forecasting has attracted increasing attention to its vital role in intelligent transportation systems (ITS), many approaches have been proposed for improving the performance of traffic forecasting. Aiming at capturing complex spatial and temporal dependencies while maintaining efficient inference, we propose a novel model, named Conditionally Parameterized Graph Convolutional Network (CPNet), to model the dy-namics of traffic data from spatial and temporal dimensions for traffic forecasting. Specifically, in the temporal dimension, we design a novel multi-scale temporal con-volution module, which captures the temporal dynamics of traffic data from different scales. It is beneficial for both long and short-term forecasting. In the spatial dimension, we develop a conditional parameterized graph convolution module to exploit the spatial dependencies in different ranges. In addition, an attention layer is designed to model the nonlinear and dynamic features. Extensive experiments on four real-world datasets demonstrate the superiority of CPNet against the state-of-the-art baseline models. & COPY; 2023 Elsevier B.V. All rights reserved.
MoreTranslated text
Key words
Traffic prediction,Temporal correlations,Spatial correlations,Attention mechanism,Graph convolution network
AI Read Science
Must-Reading Tree
Example
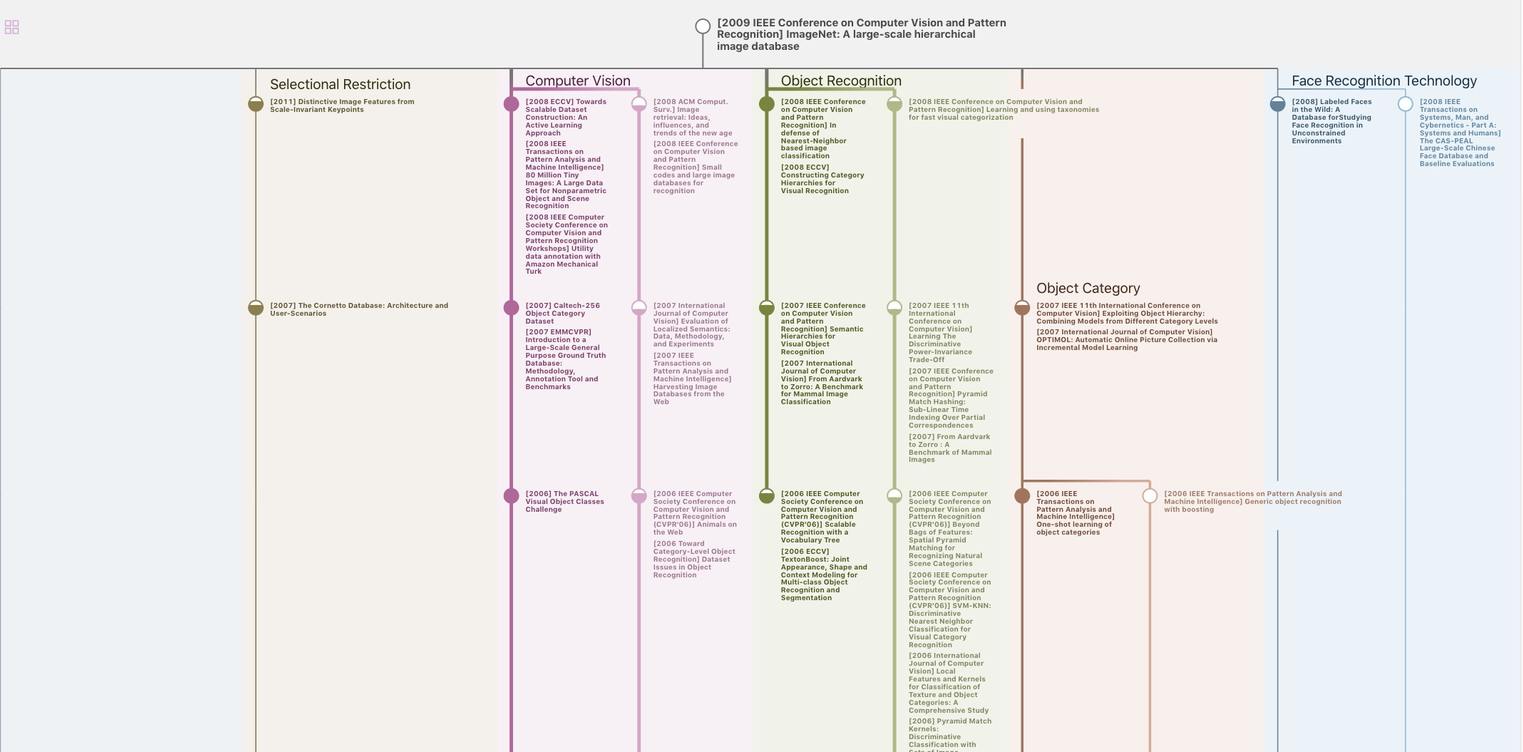
Generate MRT to find the research sequence of this paper
Chat Paper
Summary is being generated by the instructions you defined