Deep forest auto-Encoder for resource-Centric attributes graph emb e dding
Pattern Recognition(2023)
摘要
Graph embedding is an important technique used for representing graph structure data that preserves intrinsic features in a low-dimensional space suitable for graph-based applications. Graphs containing node attributes and weighted links are commonly employed to model various real-world problems and issues in computer science. In recent years, a hot research topic has been the exploitation of diverse in-formation, including node attributes and topological semantic information, in graph embedding. However, due to limitations in deep learning based on neural networks, such information has not been fully uti-lized nor adequately integrated in existing models, leaving graph embedding unsatisfactory, especially for large resource graphs (e.g., knowledge graphs and task interaction graphs). In this study, we introduce a resource-centric graph embedding approach based on deep random forests learning, which reconstructs graphs using a deep autoencoder to achieve high effectiveness. To accomplish this, our approach employs three key components. The first component is a preprocessor driven by graph similarity, alongside mod-ularity and self-attention modules, to comprehensively integrate graph representation. The second com-ponent utilizes local graph information structures to enhance the raw graph. Finally, we integrate diverse information using multi-grained scanning and dual-level cascade forests in the deep learning extractor and generator, ultimately producing the final graph embedding. Experimental results on seven real-world scenarios show that our approach outperforms state-of-the-art embedding methods.& COPY; 2023 Elsevier Ltd. All rights reserved.
更多查看译文
关键词
Graph embedding,Deep random forest,Deep auto -encoder,Self-attention
AI 理解论文
溯源树
样例
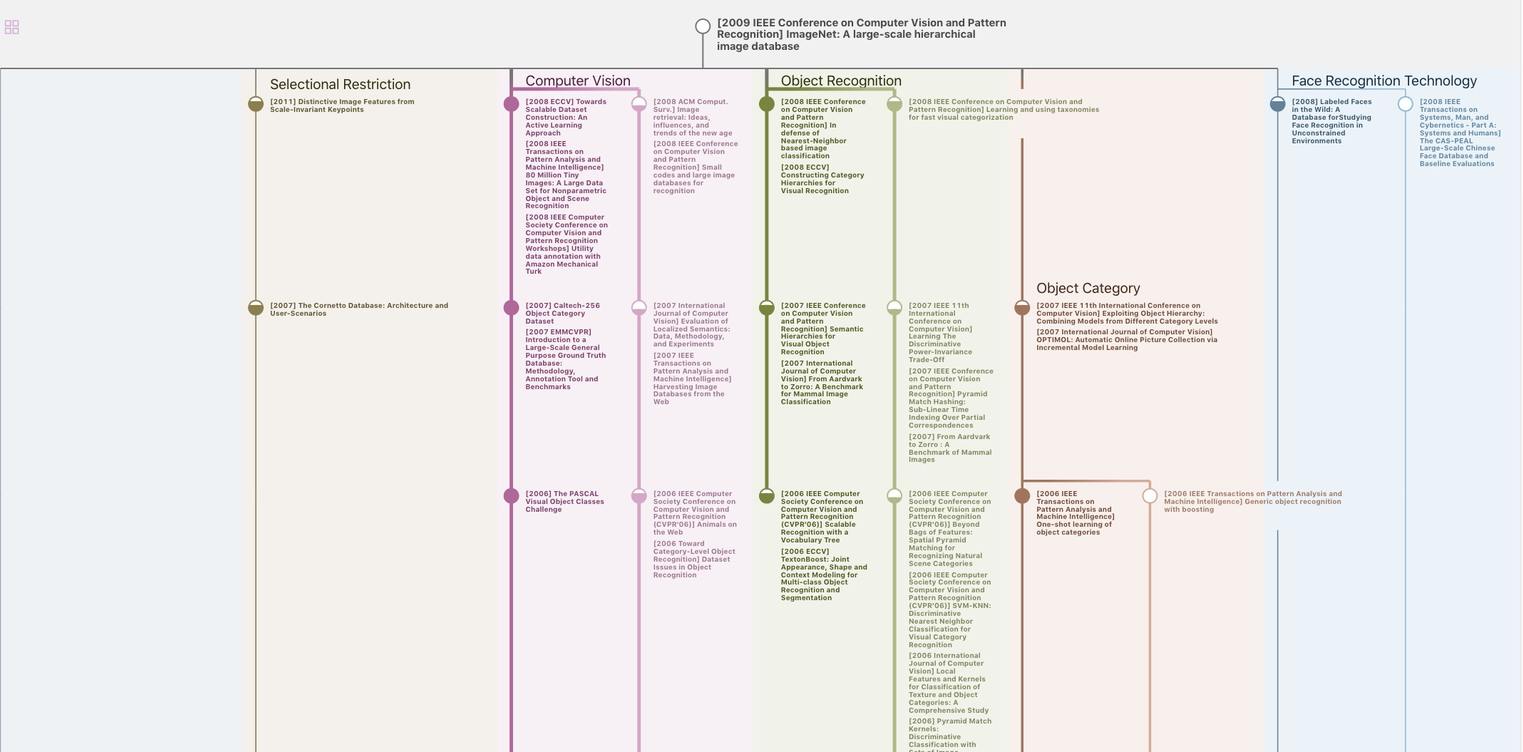
生成溯源树,研究论文发展脉络
Chat Paper
正在生成论文摘要