Cross-modal attention fusion network for RGB-D semantic segmentation
Neurocomputing(2023)
摘要
RGB-D semantic segmentation is crucial for robots to understand scenes. Most existing methods take depth information as an additional input, leading to cross-modal semantic segmentation networks that cannot achieve the purpose of multi-scale global and local cross-modal feature complementation. In this paper, we propose a cross-modal attention fusion network for RGB-D semantic segmentation. Specifically, we adopt a coordinate attention feature interaction module (CA-FIM) to aggregate RGB and depth features at the spatial and channel levels through the coordinate attention mechanism. Then, the gated cross-attention feature fusion module (GC-FFM) fuses the expanded modal features to achieve cross-modal global inference by the gated cross-attention mechanism. Utilizing the above two modules in four stages of the network, our framework can learn multi-modal and multi-level information to reduce the uncertainty of the final prediction. Extensive experiments on the NYU Depth V2, SUN RGB-D, and Cityscapes datasets demonstrate that our cross-modal attention fusion network is effective in RGB-D semantic segmentation for various complicated scenes.
更多查看译文
关键词
fusion,segmentation,attention,cross-modal
AI 理解论文
溯源树
样例
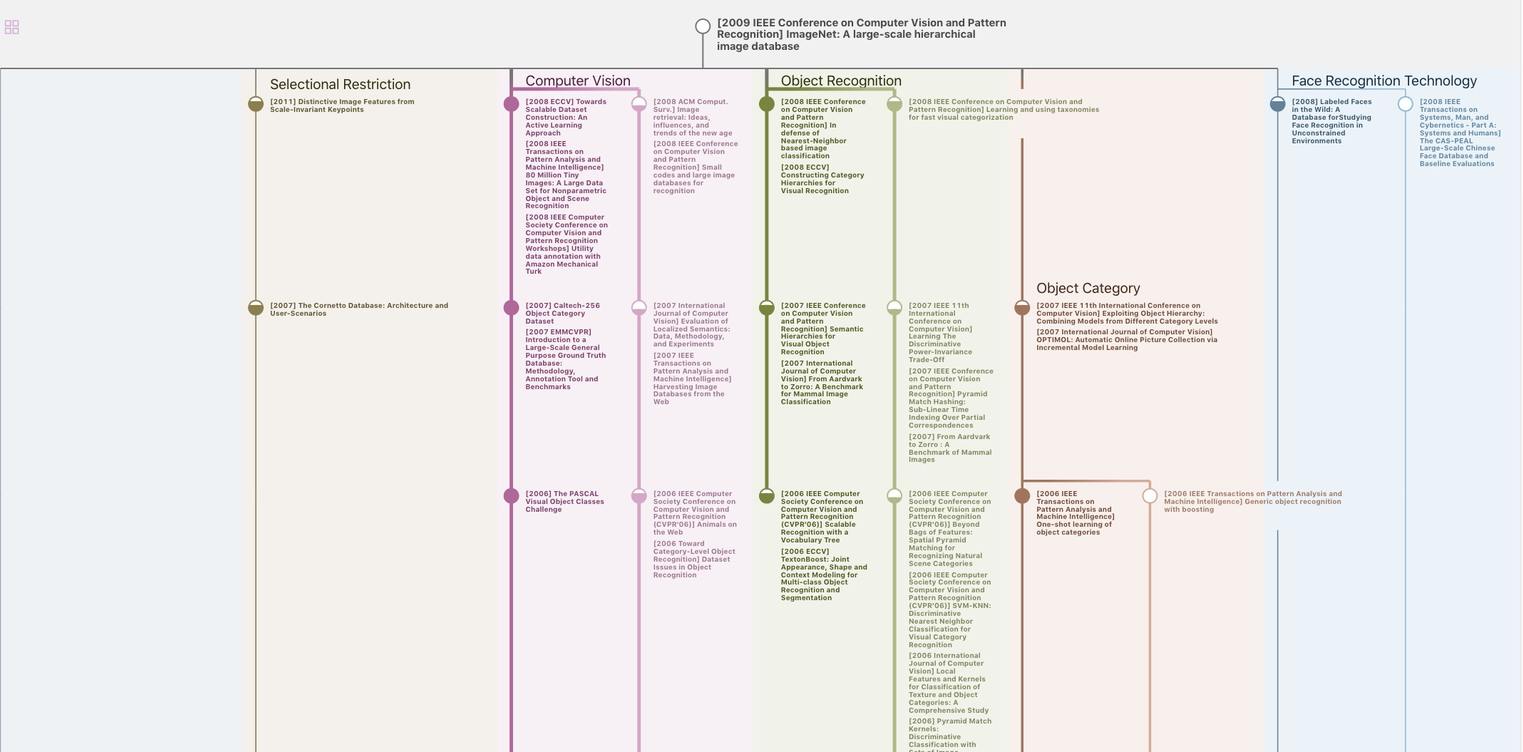
生成溯源树,研究论文发展脉络
Chat Paper
正在生成论文摘要