87P Prognostic models of recurrence-free survival in non-small cell lung cancer
Journal of Thoracic Oncology(2023)
摘要
Accurate prediction of recurrence-free survival (RFS) in patients undergoing radical surgery for early-stage non-small cell lung cancer (NSCLC) is necessary to improve outcomes. We aimed to develop pre- and post-operative prognostic models based on a range of clinicopathological factors using machine learning. Retrospective data was collected from patients treated with radical surgery from 2015 to 2021, and 66 clinicopathological features were extracted. Three regularised Cox models were trialled (Ridge, LASSO and Elastic Net) and features were selected using a ‘maximum relevancy-minimum redundancy’ approach. Model development and validation were performed using nested cross-validation. Performance was assessed using the Concordance Index (C-index), Cumulative Dynamic Area Under the Receiver Operating Characteristic Curve (AUROC) and Dynamic Brier Score. 392 patients were included; 145 (37%) patients developed recurrence or died from all causes, and median RFS was 74 months. The Elastic Net model – trained using systemic inflammatory response index [SIRI], eosinophil count, pre-operative nodal stage, weight loss, performance status and maximum standardized uptake value (SUVmax) – and the Ridge model – using performance status, weight loss, SIRI, eosinophil count, lymphovascular invasion, visceral pleural invasion, and pathological stage – proved optimal for pre- and postoperative prognosis, respectively (table). Table 87PPrognostic performance of pre- and post-surgical modelsModelRegularisationN FeaturesC-IndexMean AUROCMean Brier ScorePre-surgicalElastic Net60.70 ± 0.030.72 ± 0.020.18 ± 0.05Post-surgicalRidge70.75 ± 0.040.79 ± 0.020.16 ± 0.04 Open table in a new tab Both models had better performance at predicting earlier recurrence or death with a pre-surgical and post-surgical 1-year AUROC of 0.73 ± 0.03 and 0.83 ± 0.08 respectively. Our prognostic models demonstrate robust prediction of RFS in early-stage NSCLC, and may identify patients who will benefit from peri-operative anti-cancer therapy and/or closer post-operative surveillance. Future work is required to validate these models externally and prospectively.
更多查看译文
关键词
lung cancer,prognostic models,recurrence-free,non-small
AI 理解论文
溯源树
样例
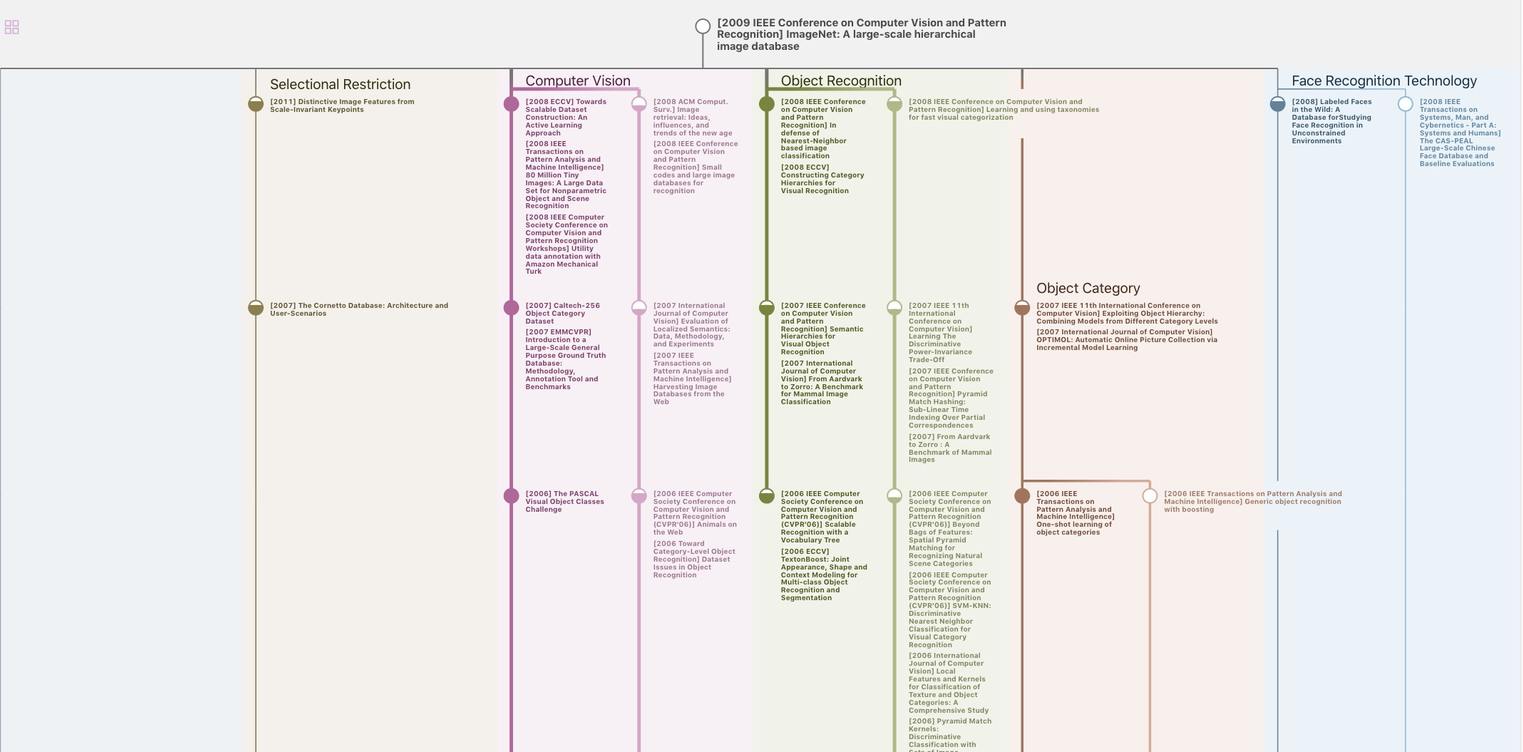
生成溯源树,研究论文发展脉络
Chat Paper
正在生成论文摘要