Robust communication-efficient decentralized learning with heterogeneity
Journal of Systems Architecture(2023)
摘要
In this paper, we propose a robust communication-efficient decentralized learning algorithm, named RCEDL, to address data heterogeneity, communication heterogeneity and communication efficiency simultaneously in real-world scenarios. To the best of our knowledge, this is the first work to address the above challenges in a united framework. In detail, we design a compressed cross-gradient aggregation mechanism with delay to resolve the Non-IID issues, a blocking-resilient mechanism which allows receiving delayed parameters and gradients, and a communication-efficient mechanism including parameters compression and adaptive neighbors selection methods to reduce the communication cost as much as possible. In addition, we also provide convergence analysis of RCEDL and prove its convergence rate O(1NK) same with the state-of-the-art decentralized learning algorithms. Finally, we conduct extensive experiments to evaluate RCEDL algorithm on two widely used datasets CIFAR-10 and MNIST under different experimental settings. Compared with the state-of-the-art baseline methods, the proposed RCEDL is much more robust with higher accuracy and at least 3.4 × communication cost reduction under the heterogeneous environment.
更多查看译文
关键词
Decentralized learning, Communication-efficient, Heterogeneity
AI 理解论文
溯源树
样例
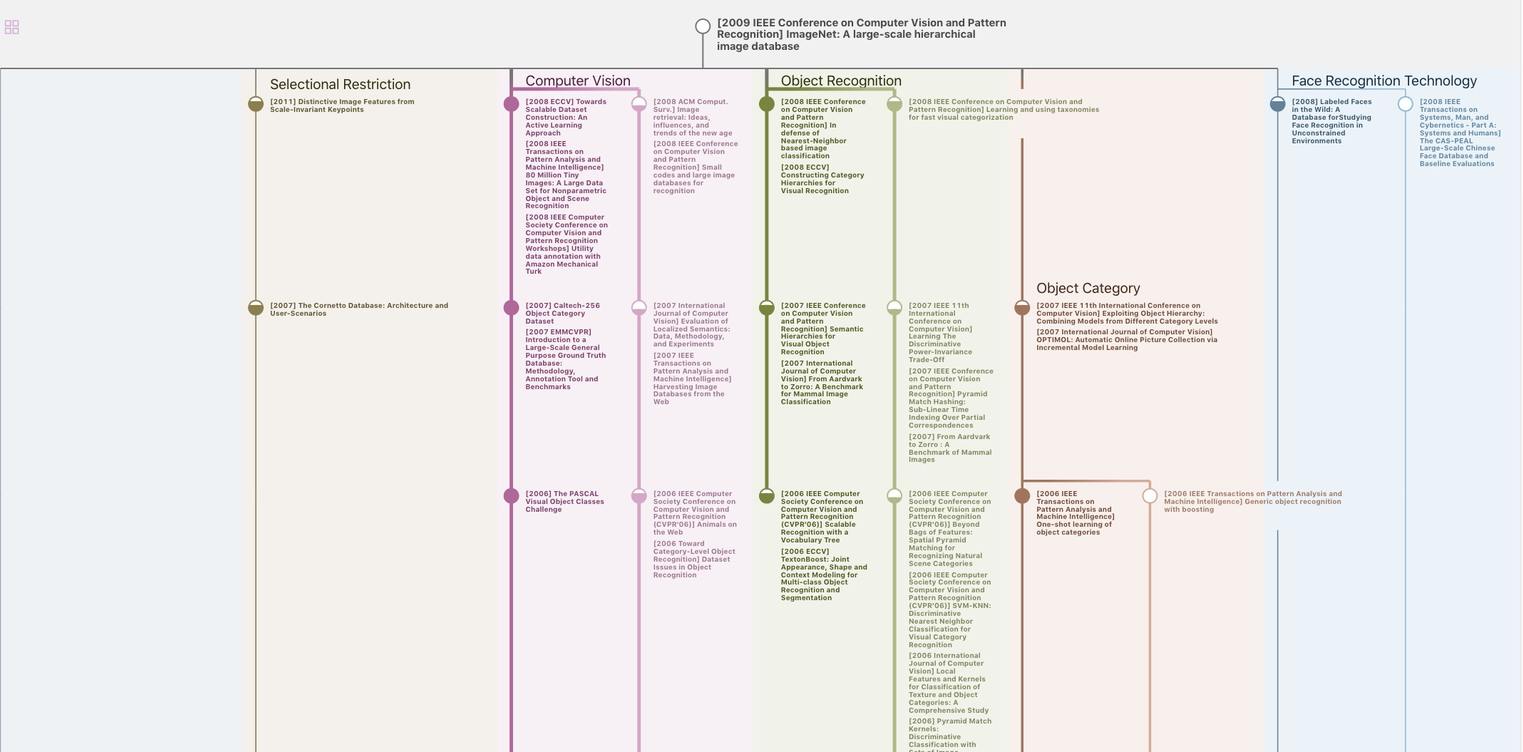
生成溯源树,研究论文发展脉络
Chat Paper
正在生成论文摘要