Po-04-213 overcoming errors of machine learning algorithms: a deep learning modular ecg approach for cardiologist assisted adjudication of atrial fibrillation and atrial flutter episodes
Heart Rhythm(2023)
摘要
Deep learning (DL) methods substantially improve the accuracy of automated ECG algorithms. Long-term ECG data (up to 30 days) collected in ambulatory environments significantly reduce performance rates, lead to many diagnostic errors and therefore increase the time to adjudication by qualified physicians. Overcome the diagnostic accuracy limitations of computerized ECG analysis with a modular lead-dependent DL software and a user interface to enable or disable DL module. Errors in computerized ECG analysis happens for different reasons. The most frequent errors are related to poor quality segments during daily activities and to low-amplitude electrical activity in atrial arrhythmias. We have developed for each Holter lead a three-part modular deep neural network (DL modules) based on three ECG features of atrial fibrillation (Afib) and atrial flutter AFL : regular or irregular heart rate, presence of absence of P, fibrillatory or flutter waves, and general appearance of arrhythmia. The validation database consisted of annotated two-leads 24-hour recordings collected from 187 patients with both paroxysmal and permanent Afib and AFL episodes divided into 60-second non-overlapping windows (i.e. 249,419 one-minute sample database). F1 scores for the fully automated analysis were 0.99 for normal sinus rhythm (NSR), 0.95 for Afib and 0.89 for AFL. The system includes a dedicated user interface, each DL module in a given Holter ECG lead can be transiently or permanently disabled by a trained cardiologist, depending on ECG quality and amplitude of the atrial electrical activity. Only 8 patients required manual deactivation of at least one DL module, which required a negligible editing time per recording. The main manual decisions were to remove a poor-quality lead and/or to disable the P-wave DL module. The second-pass F1 score were 0.99 for NSR, 0.98 for Afib and 0.96 for AFL. A modular deep learning architecture with user interface to disable ECG features significantly improves the performance rates of computerized Holter ECG analysis and decrease editing time. Further developments will include automated activation and deactivation to avoid any manual re- reading
更多查看译文
关键词
atrial fibrillation,machine learning algorithms,cardiologist assisted adjudication
AI 理解论文
溯源树
样例
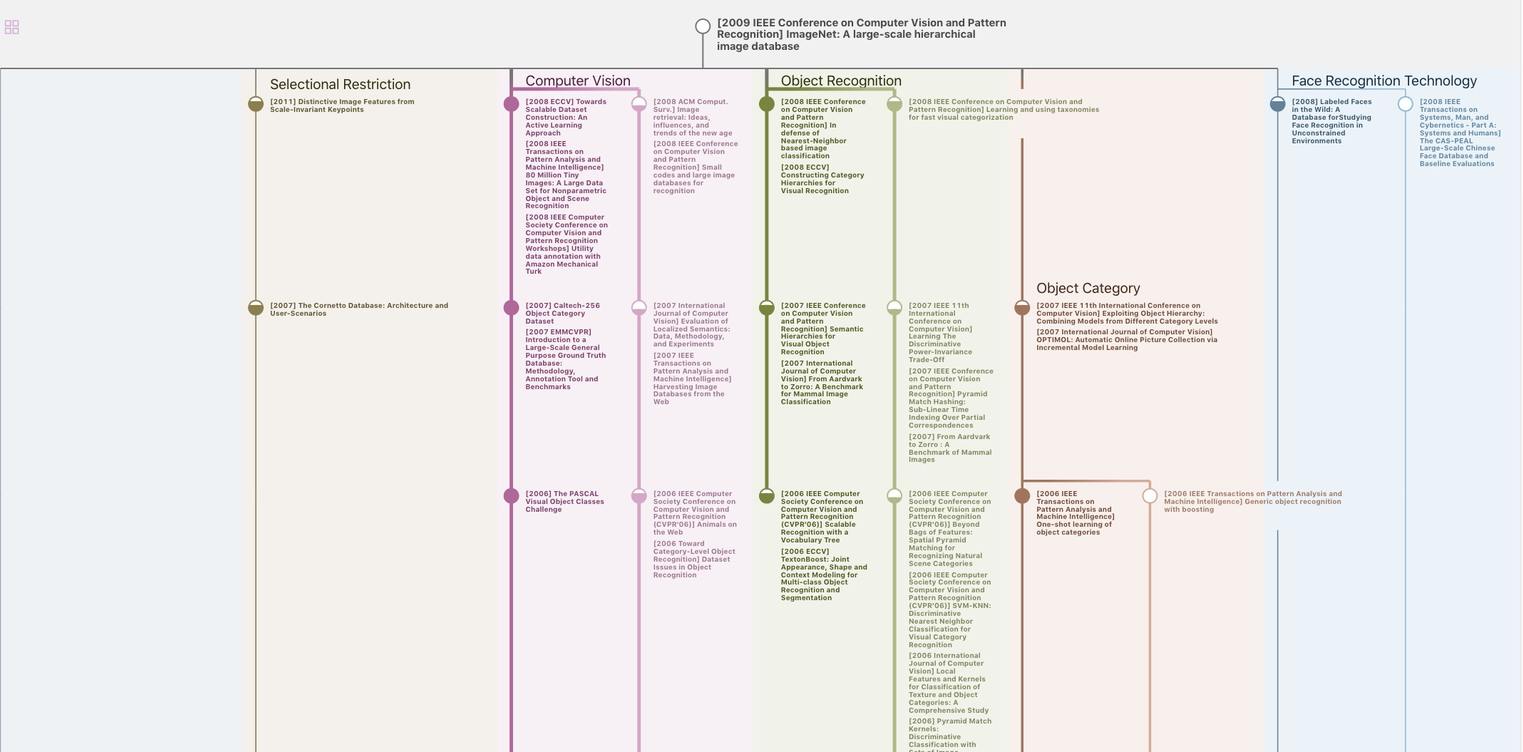
生成溯源树,研究论文发展脉络
Chat Paper
正在生成论文摘要