Po-04-183 artificial intelligence-enabled model for early detection of atrial fibrillation during sinus rhythm and mortality risk stratification
Heart Rhythm(2023)
摘要
It remains a challenge to diagnose a paroxysmal episode of atrial fibrillation (AF) as the physician often fails to capture the episode during an instantaneous attack. Several previous trials have demonstrated the need for a more targeted approach to the screening of atrial fibrillation. We aimed to develop an AI-enabled model for early detection and risk stratification of AF using 12-lead electrocardiograms (ECGs) during sinus rhythm. ECGs of patients diagnosed with paroxysmal AF were compared to those without any history of the disease using a deep neural network. Medical records, 12-lead ECGs, or ambulatory ECGs were used to diagnose AF. A proposed risk stratification algorithm of AF diagnoses by AI model divided patients into four groups: (1) low-risk patients (CHA2DS2-VASc 0-1 for male and 0-2 for female) with AI determined AF or not AF (2) high-risk patients (CHA2DS2-VASc 2-8 for male and 3-9 for female) with AI determined AF or not AF. Those patients were further correlated with future cardiovascular and all-cause mortality. A total of 47,892 consecutive patients were retrieved for ECGs with sinus rhythm. Among them, 4121 (8.6%) patients with AF history from 1 January 2009 to 31 December 2017. The area under the curve (AUC) of the AI model in detecting the presence of AF during sinus rhythm was 0.92 (sensitivity: 84.5%, specificity: 86.1%). The Kaplan-Meier curves showed that there was significantly higher mortality (p < 0.001 for cardiovascular [CV] mortality; p < 0.001 for all-cause mortality) in AI determined AF group in high-risk patients but no difference (p = 0.44 for cardiovascular [CV] mortality; p = 0.44 for all-cause mortality) in low-risk patients during six years follow-up (Figure). In another independent test dataset, the AUC of the deep learning model in detecting the presence of AF was 0.82 (sensitivity: 75.5%, specificity: 75.4%). In addition to potentially detecting AF even during sinus rhythm, the AI ECG model is an attractive tool for risk stratification. In the high-risk population, timely identification by the AI model increases the likelihood that adverse outcomes might be reversed.
更多查看译文
关键词
atrial fibrillation,sinus rhythm,early detection,mortality,intelligence-enabled
AI 理解论文
溯源树
样例
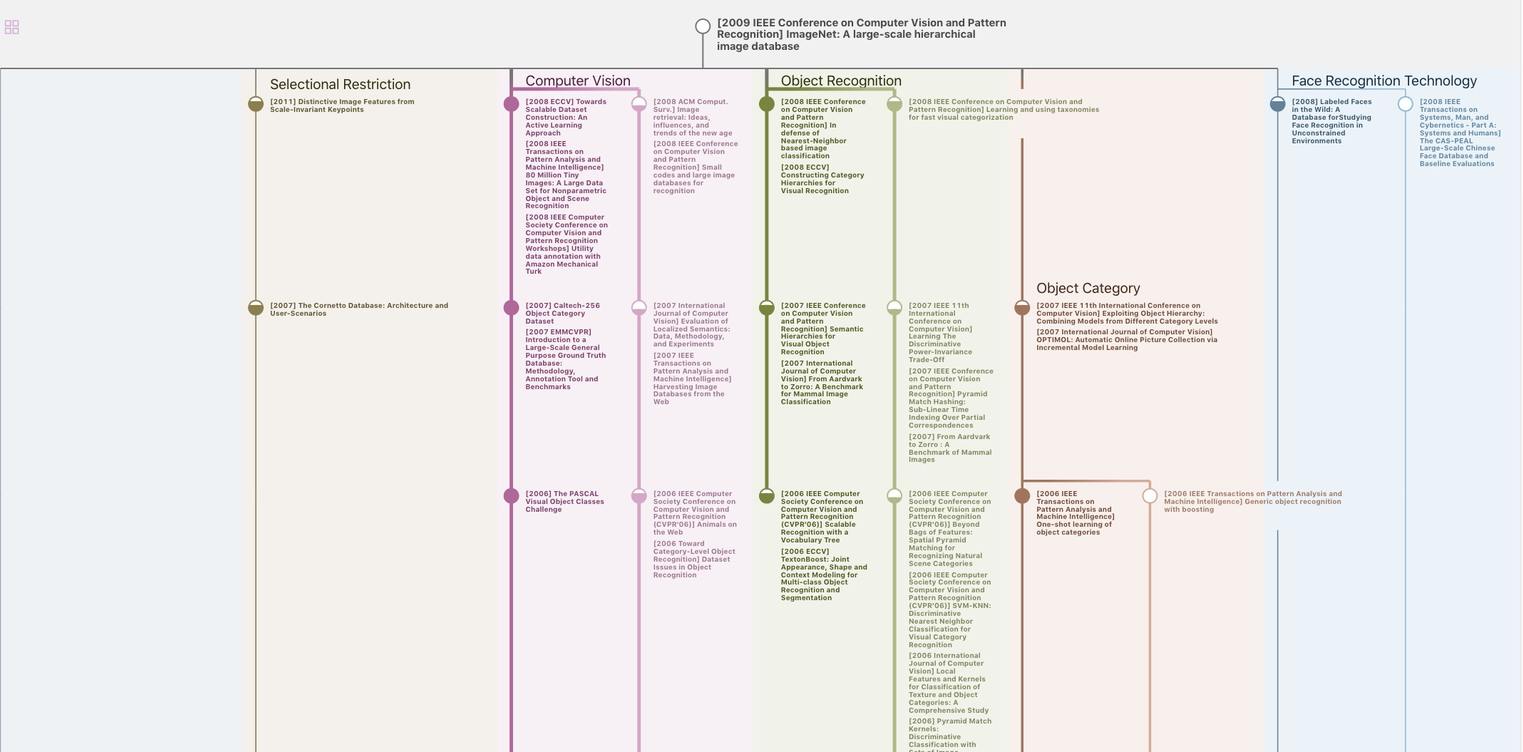
生成溯源树,研究论文发展脉络
Chat Paper
正在生成论文摘要