Po-04-181 automatic prediction of isthmus areas in scar-related ventricular tachycardia using artificial intelligence
Heart Rhythm(2023)
摘要
Ablation of scar-related reentrant VT is challenging, as the rhythm is hemodynamically unstable. Durable freedom from VT requires ablation of protected isthmus areas. Although substrate-based mapping strategies are commonly used, ablation is still done during tachycardia in some institutions. Recurrence is common and improved strategies to identify ablation targets are needed. Recently, machine learning (ML) has been more commonly used in medicine. However common ML techniques use input that is ordered in a regularly spaced matrix, unlike the data collected during ablations. A graph convolutional network (GCN) is a type of ML model that can process spatially heterogenous data. We hypothesized that a GCN could be trained to identify isthmus areas in VT ablation. Train and test a supervised GCN in the automatic prediction of VT isthmuses using a vector of features custom-calculated from the sampled EGMs. Patients undergoing VT ablation during tachycardia using the Abbott or CARTO systems were screened. Thirteen features assessing EGM morphology and wavefront propagation were calculated for each point. Known isthmuses were labelled. A leave-one-out cross-validation strategy was used, wherein each case was successively sequestered as a test case, while the remaining were used for training. During testing, an isthmus probability map was generated, and points with a > 90% probability were grouped into proposed isthmus areas based on density and distance thresholds. The primary outcome was distance between the centroids of the closest proposed and the true isthmus areas. Eleven LV tachycardias were mapped in 10 patients (median age 69, 100% male), 7 with ischemic and 3 with non-ischemic VT. Two patients had an LVAD. One VT (9.1%) was epicardial. Ablations were acutely successful in all patients. A total of 8033 points were analyzed. Of these, 491 (6.1%) were grouped into proposed isthmus areas. No prediction was made in one case. Among cases with predictions, there was 2.6 +/- 0.5 proposed isthmus areas per case. The distance between centroids was 13.5 +/- 2.8 mm. The mean area overlap, measured by the Dice coefficient, was 15.2 +/- 6.8%. EGM features can be calculated and used to train a GCN that can predict isthmus areas in VT. This may help identify critical sites for ablation in their treatment.
更多查看译文
关键词
ventricular tachycardia,isthmus areas,automatic prediction,artificial intelligence,scar-related
AI 理解论文
溯源树
样例
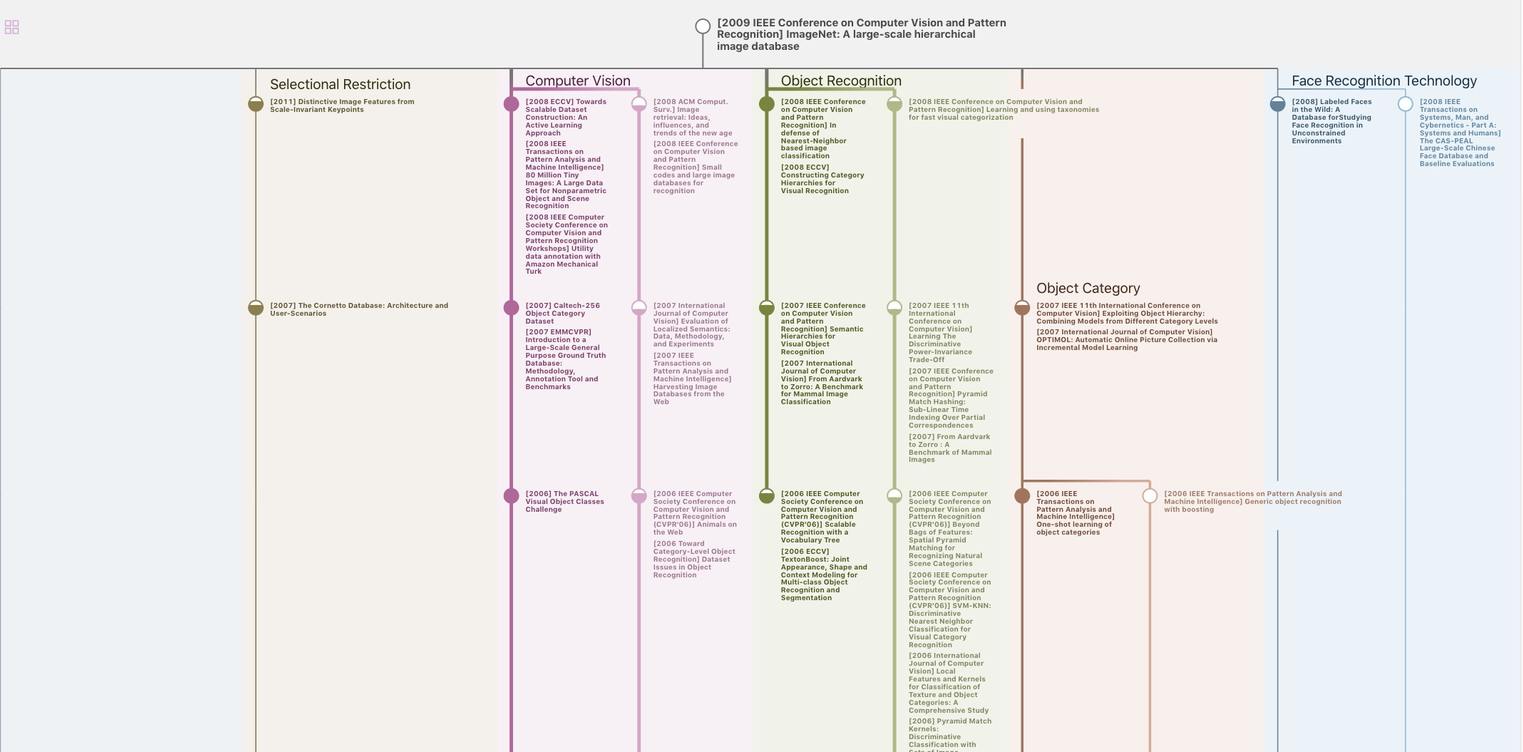
生成溯源树,研究论文发展脉络
Chat Paper
正在生成论文摘要